The second part of the third step concerns the alignment of the micro data to the national accounts totals. Dependent on the size of the gaps, this may have an important impact on the results. This chapter explores potential reasons for the gaps and presents a framework that may assist in their allocation to the relevant households.
OECD Handbook on the Compilation of Household Distributional Results on Income, Consumption and Saving in Line with National Accounts Totals
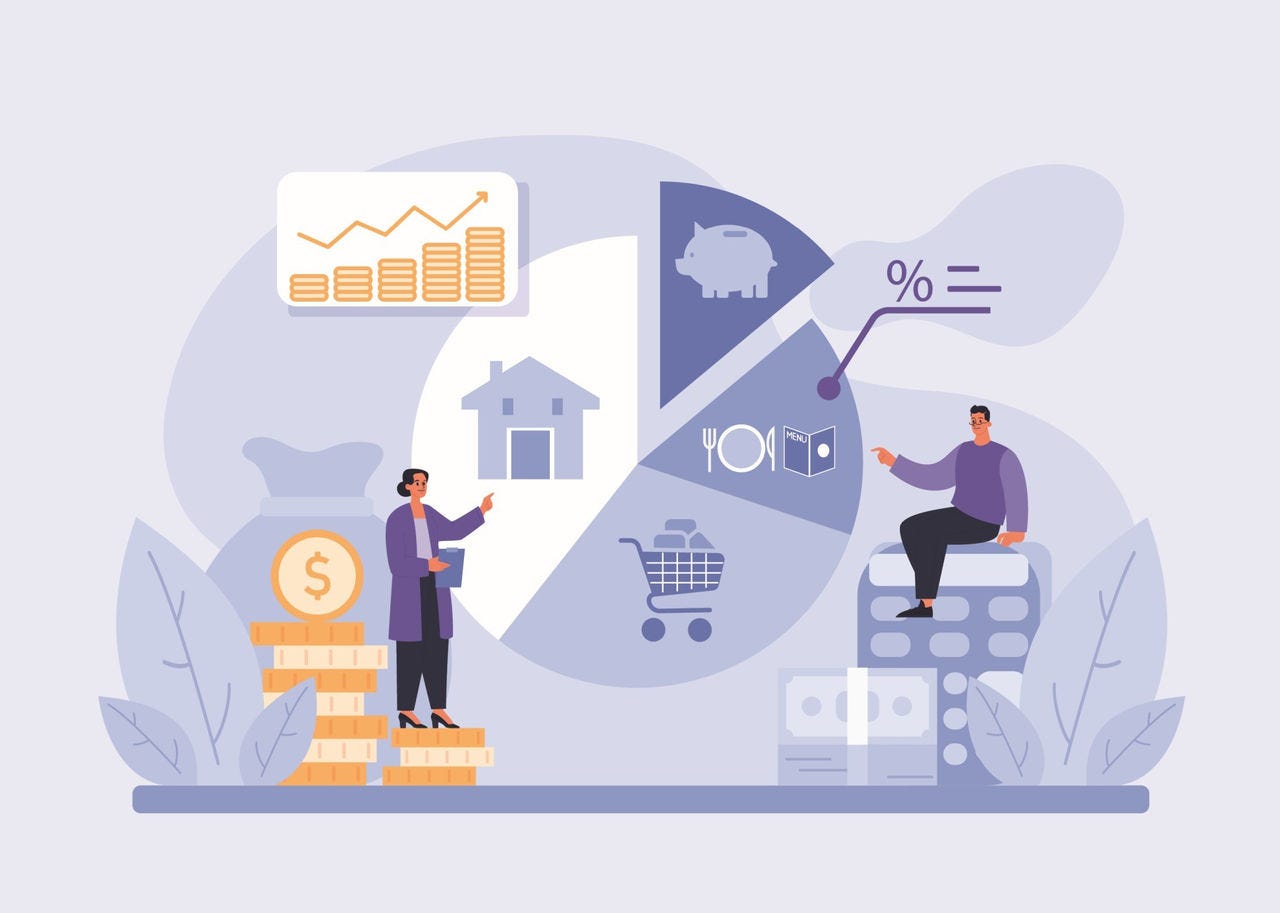
7. Aligning micro data with national accounts totals
Abstract
7.1. Introduction
In the second part of the third step, after imputations have been made for items for which micro data are lacking, micro data have to be aligned to the national accounts totals. How this step may affect distributional results will mainly depend on the size of the gaps between the micro data and the national accounts totals, and on the available information on how to allocate the amounts to the relevant households.
The alignment of the micro data to the national accounts aggregates may have a large impact on the results. That is why it is important to look at the most likely reasons for these gaps and to allocate them to the underlying households accordingly. A simple proportional or equal allocation across the distribution may otherwise only add bias to the distributional results instead of providing a more comprehensive and consequently a more accurate overview of inequality.
This chapter presents a framework that may be used to properly allocate micro-macro gaps to the relevant households. It distinguishes the various reasons that can be causing any gaps between the micro and macro data and provides compilers the possibility to allocate the gaps to underlying households on the basis of the most likely underlying reasons. Compilers are encouraged to use this framework as it is assumed to lead to better results than simply applying a proportional allocation. Whereas this chapter presents the basic framework, Chapters 10 and 11 discuss the various income and consumption items in detail, also providing more background information on the most likely causes for gaps between the micro and macro data.
The chapter first discusses the possible impact of gaps between micro and macro data on distributional results and how compilers may assess this impact for their results in Section 7.2. It then provides an overview of the items that have shown the largest micro-macro gaps in countries in Section 7.3. This is followed by an explanation of the main reasons that may be causing the gaps between the micro and macro aggregates, which forms the first part of the framework in Section 7.4. In Section 7.5, it is explained how the gaps can be allocated to the relevant households on the basis of the most likely underlying reasons. The chapter concludes with an overview of the framework in Section 7.6.
7.2. The impact of micro-macro gaps in compiling distributional results
To assess the possible impact of gaps between the micro data used in the compilation process and the macro totals derived after step 1, one can look at their share in the overall results. In that regard, it has to be understood that the distributional outcomes are the result of underlying micro data,1 imputations for items or part of the population for which micro data is lacking,2 and the alignment of these micro data to the macro aggregates. A method to acquire more insight into the role of these three components in the compilation of distributional results is by deriving their coverage rates. This can be done by looking at the relative shares of micro data, imputations and alignments in the absolute flows that constitute adjusted disposable income and actual consumption expenditure.
As adjusted disposable income consists of positive and negative items, the absolute flows should be considered to get a correct view of the contributions of each of the three factors to this aggregate. The relative shares for the imputations and alignments should then be calculated by dividing the sum of their absolute values by the sum of the absolute flows constituting adjusted disposable income and actual final consumption. In deriving the share of the micro data, it has to be borne in mind that micro totals can exceed the macro aggregates, so simply looking at the sum of the micro totals as percentage of the absolute flows would not provide a correct picture. Therefore, the share of the micro total in the balancing items should be derived as a residual, i.e. after deduction of the shares of the imputations and the alignments.
Table 7.1 provides an example of how this works in practice. It shows an aggregate that is composed of five underlying items, the first three items positively contributing to the aggregate while the last two contributing negatively. For three of the items micro data underlying the distributional results is available. For two items no micro data is available (“NAV”), so distributions will have to be derived via imputations.
Table 7.1. Example of deriving contributions of alignment and imputation
Adjusted NA aggregate (1) |
Micro aggregate (2) |
Micro-Macro gap (3) = (2) – (1) |
Imputation (4) = (1) if (2) is NAV |
|
---|---|---|---|---|
Item A |
90 |
70 |
20 |
- |
Item B |
50 |
NAV |
- |
50 |
Item C |
60 |
85 |
-25 |
- |
Item D (-) |
35 |
30 |
5 |
- |
Item E (-) |
15 |
NAV |
- |
15 |
Total (= A + B + C – D – E) |
150 |
125 |
-10 |
35 |
Source: The Author.
To derive the contribution of the alignment of the micro-macro gaps in the example above, the sum of the absolute amounts of the micro-macro gaps should be divided by the absolute amounts that constitute the total, i.e.
The contribution of the imputation can be computed by dividing the sum of the absolute amounts of the imputations by the absolute amounts that constitute the total, i.e.
The contribution of the micro data can now be derived as a residual, i.e.
Figure 7.1 and Figure 7.2 show the share of each of these three components in the total absolute flows that constitute adjusted disposable income and actual final consumption expenditure for the household sector as a whole, derived from the EG DNA exercise conducted in 2015.
It can be concluded that the distributional results are to the largest extent based on micro data, but that the impact of imputations and alignments on the distributional results is often significant. In the 2015 exercise, micro data sources covered more than 70% of the underlying flows on average for adjusted disposable income, whereas this was more than 60% for actual final consumption expenditure.
The impact of the imputations and alignments on the distributional results can best be reviewed by presenting the size of these adjustments in absolute terms as percentage of the balancing items. This provides insight into the maximum amount that has to be allocated to the various households. Whereas positive and negative adjustments may (partly) cancel out at the level of the household sector as a whole, their overall impact on distributional results may still be significant, especially when they are allocated differently to the various household groups.
Figure 7.3 and Figure 7.4 present the impact of imputations and alignments on adjusted disposable income and actual consumption expenditure respectively, for the household sector as a whole, on the basis of the results of the 2015 exercise. For most countries, the size of the alignments is larger than that of imputations which relates to the fact that most countries have micro data available for the majority of items and only need to rely on imputations for few of them. In that regard, it should also be mentioned that the number of items for which countries report imputations and alignments varies across countries.
Figure 7.1. Contribution of micro data, alignment and imputation to adjusted disposable income
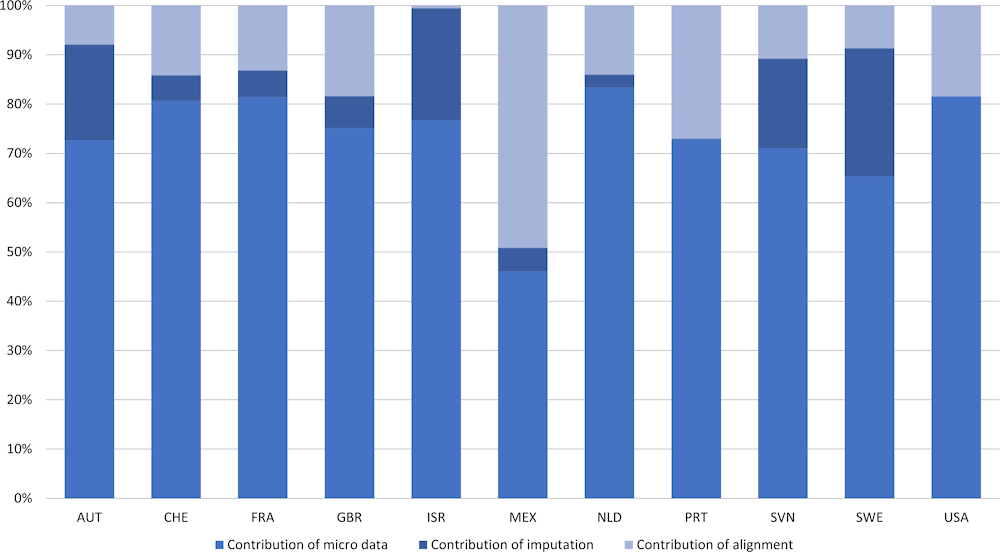
Source: The Author.
Figure 7.2. Contribution of micro data, alignment and imputations to actual final consumption expenditure
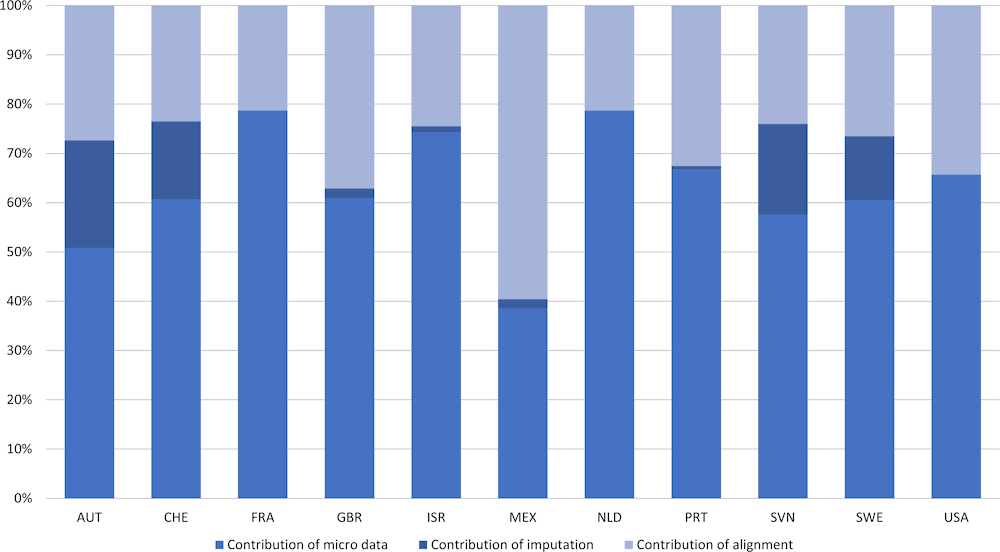
Source: The Author.
The size of the alignments appears to be significant in all countries. When looking at the average for all countries, the impact of alignments was 26.5% for adjusted disposable income and 30.2% for actual consumption expenditure in the 2015 exercise. The method for allocating alignments to underlying individuals or households may significantly affect distributional results. Ideally, information is available for the correct allocation of the amounts to the relevant individuals or households, but often (part of) the allocation may need to be done on the basis of some assumptions. It will depend on the degree of information and on the robustness of the assumptions how this will affect distributional results and to what extent this may add to the margins of error surrounding the results (see also Chapter 12).
Figure 7.3. Size of the absolute alignments and imputations as percentage of adjusted disposable income for the household sector as a whole
Figure 7.4. Size of the absolute alignments and imputations as percentage of actual consumption expenditure for the household sector as a whole
7.3. Items that show largest gaps
The previous section showed that the alignment of gaps between micro and macro data has a large impact on the distributional results in most of the countries. This implies that for some items large gaps exist between the micro aggregates and the national accounts totals. Table 7.2 shows the adjustment coefficients for the main income components on the basis of the exercise conducted in 2015. The adjustment coefficient shows by how much the micro results need to be adjusted to align them with the adjusted national accounts totals. It is calculated as the adjusted national accounts aggregate divided by the micro aggregate. The table shows the number of countries for which an adjustment coefficient could be calculated (i.e. micro data was available to compile the distributional results), the average value of the coefficient, the median value and the minimum and maximum values in the exercise. Table 7.3 presents results for the main expenditure components.
Table 7.2. Adjustment coefficient for the main income components
National accounts aggregate divided by the corresponding micro aggregate, EG DNA exercise 2015.
Code |
Instrument |
Number of countries |
Average |
Median |
Minimum |
Maximum |
|||||||
---|---|---|---|---|---|---|---|---|---|---|---|---|---|
most recent year |
second most recent year |
most recent year |
second most recent year |
most recent year |
second most recent year |
most recent year |
second most recent year |
||||||
B2 |
Operating surplus |
4 |
1.79 |
1.27 |
1.90 |
1.27 |
0.94 |
1.12 |
2.43 |
1.42 |
|||
B3 |
Mixed income |
4 |
2.20 |
1.79 |
2.00 |
1.79 |
1.30 |
1.67 |
3.50 |
1.91 |
|||
D1R |
Compensation of employees |
3 |
1.19 |
… |
1.20 |
… |
1.16 |
… |
1.20 |
… |
|||
D41R |
Interest (not adjusted for FISIM), received |
8 |
2.08 |
1.90 |
1.56 |
1.05 |
0.66 |
0.72 |
6.40 |
4.77 |
|||
D42R |
Distributed income of corporations |
7 |
5.06 |
10.67 |
1.88 |
5.53 |
0.70 |
3.00 |
17.76 |
23.50 |
|||
D41P |
Interest (not adjusted for FISIM), paid |
9 |
3.58 |
2.47 |
2.94 |
1.50 |
1.02 |
1.01 |
11.31 |
4.65 |
|||
D5P |
Current taxes on income and wealth |
10 |
1.18 |
1.19 |
1.18 |
1.15 |
0.78 |
0.74 |
1.54 |
1.78 |
|||
D61P |
Net social contributions |
2 |
1.23 |
2.01 |
1.23 |
2.01 |
1.19 |
1.28 |
1.27 |
2.73 |
|||
D62R |
Social benefits other than STiK |
10 |
1.22 |
1.30 |
1.15 |
1.26 |
0.97 |
0.98 |
1.55 |
1.65 |
|||
D63R1 |
Education |
3 |
0.94 |
0.88 |
0.95 |
0.88 |
0.72 |
0.78 |
1.13 |
0.98 |
|||
D63R2 |
Health |
3 |
1.36 |
1.37 |
1.18 |
1.37 |
1.16 |
0.99 |
1.73 |
1.75 |
Source: Zwijnenburg (2016[1]).
It can be observed that the gaps between the micro and macro data are often quite substantial. An adjustment coefficient that is close to 1 implies good alignment, but the tables show that the average values substantially differ from 1 for most of the income and consumption components. For the majority of the items the coefficient is above 1, meaning that the micro aggregates are lower than the macro aggregates. Only in a few cases, it is the other way around.
In the 2015 exercise, distributed income of corporations (D42R) turned out to have the highest adjustment coefficient on average (5.06), followed by alcoholic beverages, tobacco and narcotics (CP020) (3.60), interest paid (D41P) (3.58), mixed income (B3R) (2.69), health (CP060) (2.47), and interest received (D41R) (2.08). Distributed income of corporations (D42R) also records the highest maximum values. The consumption components generally have smaller differences across components than income. The average coefficients are between 1.09 and 2.47 in the most recent year, and between 0.92 and 2.72 in the second most recent year, when excluding the item alcoholic beverages, tobacco and narcotics (CP020).
Table 7.3. Adjustment coefficient for the main expenditure components
National accounts aggregate divided by the corresponding micro aggregate, EG DNA exercise 2015.
Code |
Instrument |
Number of countries |
Average |
Median |
Minimum |
Maximum |
||||
---|---|---|---|---|---|---|---|---|---|---|
most recent year |
second most recent year |
most recent year |
second most recent year |
most recent year |
second most recent year |
most recent year |
second most recent year |
|||
CP010 |
Food and non-alcoholic beverages |
10 |
1.48 |
1.53 |
1.34 |
1.40 |
1.06 |
0.95 |
2.87 |
2.76 |
CP020 |
Alcoholic beverages, tobacco and narcotics |
9 |
3.60 |
5.37 |
2.51 |
2.52 |
1.68 |
1.13 |
12.00 |
21.03 |
CP030 |
Clothing and footwear |
10 |
1.57 |
1.70 |
1.25 |
1.40 |
1.09 |
1.03 |
2.90 |
2.80 |
CP040 |
Housing, water, electricity, gas and other fuels |
9 |
1.23 |
1.16 |
1.06 |
0.94 |
0.84 |
0.87 |
2.47 |
2.30 |
CP050 |
Furnishings, households equipment & house maint. |
10 |
1.60 |
1.71 |
1.41 |
1.59 |
1.15 |
0.96 |
2.93 |
2.93 |
CP060 |
Health |
9 |
2.47 |
2.72 |
2.16 |
2.27 |
1.22 |
1.15 |
4.78 |
4.74 |
CP070 |
Transport |
8 |
1.56 |
1.59 |
1.34 |
1.36 |
0.98 |
0.95 |
3.18 |
2.87 |
CP080 |
Communications |
10 |
1.25 |
1.53 |
1.26 |
1.34 |
0.71 |
1.08 |
2.28 |
2.50 |
CP090 |
Recreation and culture |
10 |
1.90 |
1.85 |
1.45 |
1.65 |
1.14 |
1.01 |
4.05 |
3.50 |
CP100 |
Education |
10 |
1.09 |
0.92 |
1.08 |
1.05 |
0.19 |
0.09 |
1.87 |
1.51 |
CP110 |
Restaurants and hotels |
10 |
1.54 |
1.32 |
1.52 |
1.29 |
0.97 |
1.06 |
2.20 |
1.64 |
CP120 |
Miscellaneous goods and services |
7 |
1.89 |
1.88 |
2.06 |
1.78 |
0.97 |
1.13 |
2.63 |
2.85 |
Source: Zwijnenburg (2016[1]).
7.4. Possible reasons for micro-macro gaps
Possible reasons for the differences between the micro results and the adjusted national accounts totals are related to the first three steps of the step-by-step approach presented in Chapter 3, related to the quality of the data and of the assumptions used in the process:
Step 1: Adjustment of the national accounts totals:
The quality of the national accounts totals
The quality of the adjustments to the national account totals
Step 2: Linking micro data source variables to the national accounts variables:
Assumptions regarding the conceptual and classification differences
Step 3: Imputation for missing elements and aligning data to national accounts totals
The quality of the correction for missing elements
The quality of the micro data – Estimation and measurement errors
The reasons for the gaps are discussed below, in accordance with the above categorisation.
7.4.1. The quality of the national accounts totals
A first possible reason for the gap between the micro and macro results may be quality issues related to the national account totals. The national accounts totals are the product of a balancing framework in which data from various data sources are combined and confronted. Often source data need to be adjusted to arrive at consistency and comprehensiveness. In that process, choices have to be made that may cause differences from the direct data sources. The quality of the data that are used in the system and the strength of the assumptions made in the balancing process will determine the quality of the final results. Gaps between micro and macro data may point to possible quality issues in this process.
In compiling the national accounts, the data for the household sector may be derived in three ways, i.e. directly based on household micro data sources (i.e. independent of other sectors in the accounts), estimated using counterpart information (from other sectors, e.g. banking statistics, pension data or government statistics), or as a residual after combining all other data sources in the system of national accounts. The robustness of the results for the household sector will depend on the quality of the various data sources used in constructing the national accounts and the amount of detail they provide. Furthermore, it will depend on how good these data sources align.
In analysing the gaps between the micro and macro results, it is important to have a more detailed look at how the national accounts results have been derived and whether this may contain any inconsistencies. Process table information that describes the various steps to get from the basic information to the final national accounts totals may be very relevant for that purpose. This may include information on adjustments made to correct for conceptual differences, to impute for missing elements, and to reach internal consistency within the framework of national accounts.
7.4.2. The adjustment of the national accounts totals
In the compilation process to arrive at distributional results, national accounts totals may have to be adjusted to exclude NPISHs, institutional households and consumption expenditure by non-residents, and to include expenditure of residents abroad at the detailed level of consumption items if this is deemed to lead to better matching of the micro and macro data. In some cases, specific information will be available to make these adjustments, but in other cases, these adjustments will have to be based on assumptions. Gaps between micro and macro results may be due to quality issues in making these adjustments. Therefore, it is also important to be transparent about the specific adjustments made in this step and in case of micro-macro gaps to discuss whether these may be partly due to incorrect adjustments or underlying assumptions in this step. For more information on this specific step, please refer to Chapter 4.
7.4.3. Conceptual differences and classification issues
Gaps may also appear as a consequence of conceptual differences and classification issues between micro and macro data. Sometimes the definition of the national accounts may vary from the one used in the survey or administrative data source, and (part of the) transactions may be classified differently. Chapters 10 and 11 provide more insight into possible conceptual differences between micro and macro results for various items.
Also, the time of recording may differ between the national accounts totals and the micro results. The latter often focus on a certain point in time (e.g. end of the quarter or end of the year), whereas the national accounts aim to capture all transactions within a certain time frame. This may give rise to differences between the micro and macro results, for instance related to changes in the population or for specific economic events that may have occurred during the period.
Moreover, data may refer to different time periods, for example if a specific survey is only conducted every other year, the information of a previous year may be used to derive results for a more recent year. This may also cause gaps between the micro and macro results.
It is important to carefully assess the reliability of any adjustments that may have been made to the micro data to adjust for any conceptual and classification differences when assessing possible reasons for micro-macro gaps.
7.4.4. Correction for missing elements
For some (sub)items or parts of the population, information may be lacking from micro data sources. In those cases, it is expected that compilers come up with imputations to correct for the missing information (see also Chapter 6). Micro-macro gaps may point to the fact that the missing part is actually smaller or larger than initially assessed. Furthermore, it may point to additional elements that may be missing from the micro data. In that regard, in case of a gap between the micro and the macro data, it is important to assess whether this can indeed be related to missing information in the micro data and, in case an imputation has already been made, whether the imputation is deemed to be correct or whether part of the remaining micro-macro gap may still relate to the need for additional imputations.
7.4.5. The quality of the micro data
Just as the macro aggregates may turn out to be incorrect, micro estimates may also be subject to quality issues. This may be increasingly the case, as many statistical offices struggle with the quality of their household surveys due to increased unwillingness to take part in surveys, to respond to specific questions, as well as increasing inaccuracy in filling out the surveys (see for example Meyer et al. (2015[2]) and Pinkovskiy et al. (2014[3])). The increased use of administrative data may partly overcome this issue, although it has to be borne in mind that these data sources come with their own downsides, not always providing matching concepts, and not always having full coverage of all parts of the population.
In general, micro data can suffer from two types of errors, i.e. estimation errors and measurement errors. Estimation errors relate to the extrapolation of the micro results to the target population and can be linked to the sample size, the representativeness of the sample and the magnitude of the non-response. The errors related to the sample size are referred to as standard sampling error, implying that the smaller the sample, the larger the variance surrounding the results, as less data underlie the ultimate estimates. The other two issues are referred to as coverage errors. These occur in the case of the sampling frame being different from or non-representative of the target population, and in the case of selective non-response, both causing bias to the results. As discussed in Chapter 5 all these aspects may cause gaps with the macro results. Especially survey data may suffer from estimation errors. Administrative data sources tend to have broad coverage and are therefore less prone to these kinds of errors. In this regard, Törmälehto (2017[4]) reports a striking example of France that changed from interview to register-based incomes in their EU-SILC results for 2008 which led to “a conspicuous increase in the share of property income: for the top 5 per cent, it jumped from 7.1 to 32.6% from 2006 to 2007”, possibly indicating the existence of estimation errors in the survey results.
In analysing the gaps between the micro data and the adjusted national accounts totals as derived after the first step in the compilation process, it is important to assess the likeliness of the gaps being influenced by estimation errors. For that purpose, it would be helpful to have information on the survey results in terms of underlying micro data, survey weights and standard errors. With regard to estimation errors, this may provide more insight into the margins of error surrounding the results. Furthermore, as this type of error will most likely affect more target variables at the same time, similar micro-macro gaps across multiple items may indeed be an indication of the existence of estimation errors.
Errors may also occur when the recorded values depart from the actual true values. These are referred to as measurement errors and may relate to item non-response or the reporting of incorrect data. These may be due to misinterpretation of the questions, difficulty by respondents to re-call the exact values, and deliberately reporting incorrect data. As was explained in Chapter 5, a lot of statistics have to deal with measurement error and these kinds of errors seemed to have increased over time, at least for specific items. Especially questions on income are usually understood to be relatively sensitive and prone to higher non-response rates or larger measurement errors, both in survey and administrative data. Particularly income from self-employment (see Johns and Slemrod (2008[5]) and Neri and Zizza (2010[6])), property income (see Neri and Zizza (2010[6])) and social benefits (see Meyer et al. (2009[7])) are prone to underreporting. The same goes for specific consumption items, such as illegal goods and services (e.g. illegal drugs and prostitution) or for socially unacceptable goods or services (e.g. alcohol and gambling).
As measurement errors may also be responsible for gaps between the micro and macro aggregates, it is important to assess to what extent the micro data may have been liable to these kinds of errors. This may be done by looking at the consistency and plausibility of the micro results, for example by confronting information on income, consumption and wealth at the micro level, checking the information with data from other data sources, looking at consistency of the data over time, and comparing data with results for comparable households. It is important to do this in close collaboration with the micro experts responsible for the specific statistics as they will have the best knowledge of the underlying data. If any errors are detected, it is recommended to correct for these in the micro data, so that an updated micro data set can be used as new input in the compilation process of the distributional results.
7.5. Allocation of gaps to relevant households
After the gaps have been attributed to the most likely causes, the related estimates have to be allocated to the relevant households or household groups. As the allocation may differ per cause, the allocation on the basis of these underlying causes will lead to more accurate results than allocating the full gap in one go.3 For all causes that concern the micro data underlying the distributional results, specific solutions will have to be found.4 This implies addressing the issue of possible measurement errors and of possible estimations errors.
When looking at estimation errors, research has shown that non-response is often correlated to specific household characteristics. D’Alessio and Faiella (2002[8]) for example show that it is often more frequent among higher income and wealthier households.5 This is confirmed by Sabelhaus et al. (2013[9]) who analysed that high income households are underrepresented in the consumer expenditure survey in the US as they are less likely to participate. Pareto-analyses may be helpful in analysing whether the top tail of the distribution is covered in the micro data and to correct for this if needed (see Grilli et al. (2022[10])). Another option is to impute for the missing information by looking at administrative data, as done by the US Bureau of Labor Statistics in applying non-interview adjustment factors to the results of the consumer expenditure survey based on fiscal data (see U.S. Bureau of Labor Statistics (2022[11])). On the other hand, D’Alessio and Neri (2015[12]) found that in the Survey of Household Income and Wealth (SHIW) non-reporting was more frequent among the poorer and less educated. If that is the case, specific adjustments may be needed to correct for that.
Regarding the issue of measurement errors, it may in some cases be straightforward which micro data to adjust (for example in case of confrontation with data from other micro data sources)6, but in other cases, this may require specific assumptions. In that case, the analysis of the plausibility of the underlying micro results may for example provide some direction where to best allocate specific amounts in relation to the various causes for micro-macro gaps. This can for example be done on the basis of constructing full sets of accounts at the micro level, i.e. comparing information on income, consumption and wealth. As saving derived from the non-financial accounts should match the saving from the financial accounts, it may be checked whether there are inconsistencies or implausibilities at the micro level when comparing data from various sources.
In the case when not all information is available at the micro level one can try to derive results for groups of households and check the consistency and plausibility at the most detailed level possible.7 Looking at outliers and the distribution of the data within the household group may also be helpful for this purpose. Furthermore, it may be useful to look at the development of micro-macro gaps over time, also in relation to trends in micro and meso results for specific groups of households. This may also reveal insight into less plausible trends in some of the elements.
Furthermore, information from research may provide insight into what type of households are most likely involved in specific types of activities or affected by specific types of errors which may provide the underlying rationale for adjustment of these records in this specific step of the process. In relation to the non-observed economy, Coli and Tartamella (2014[13]), for example, show that non-registered workers are not equally distributed across the household sector, but show to be concentrated in specific subgroups. Furthermore, Accardo et al. (2009[14]) made specific adjustments for income “from fraud and undeclared work”, which mainly affected “self-employed, the most well-off senior managers, salaried workers in the first half of the income distribution and non-active persons, excluding retired people”. Carson (1984[15]) provides information on which household types are more likely to be involved in the underground economy or illegal activities.
A lot of research is also available on the impact of measurement errors in various statistics. Several studies confirm that misreporting often depends on socio-demographic characteristics (such as age, family type, ethnicity, income level, region and education), i.e. some groups are more likely to misreport for some items than others. Neri and Zizza (2010[6]) found that in the Survey of Household Income and Wealth (SHIW) misreporting tends to be more diffuse among males, the older, the self-employed and respondents at the higher end of the earnings distribution. They also found some regional differences in the likelihood of misreporting. Sabelhaus et al. (2013[9]) and Cifaldi and Neri (2013[16]) show that there is large underreporting at the top of the distribution. They did not find similar evidence for consumption, explaining that consumption is a less sensitive topic and more difficult to hide from an interviewer. Lohmann (2010[17]) and Romanov and Gubman (2012[18]) explain that there is also evidence that part-time and irregular employees are more likely to incorrectly report their earnings (e.g. reporting income levels for a full month that may not be representative of their average income). Furthermore, whereas some groups tend to underreport their income, it has also been the case that some other socio-demographic groups tend to over-report their income. For example, Bound and Krueger (1989[19]) found that women have a slight tendency to underreport their earnings.8 In that regard, even if the micro-macro gap is zero, there may be the need to adjust some of the underlying data at the household level.
It is clear that the allocation of the micro-macro gaps to the relevant households on the basis of the most likely underlying causes will often involve subjective decisions. The examples provided above may provide some insights into how to approach the allocation question and what groups may be more prone to what specific types of measurement errors, but it will depend on the items, the data sources and the country characteristics which approach will work best to solve and allocate any micro-macro gap for a particular item at the country level. Furthermore, it is important that any decisions on how to allocate the gap is done in close cooperation between the micro and the macro experts, as they both have specific knowledge of the underlying micro and macro data which is relevant to come up with the best possible solution.
The allocation of the amounts to the underlying households should ideally be done at the level of the micro statistics, i.e. by making adjustments to the survey or administrative data, applying imputations at the micro level, or by adjusting the survey weights to arrive at the relevant aggregates. This will lead to improved micro data that underlie the new distributional measures and will make sure that the income group classification is re-adjusted on the basis of these improved data. An alternative is to allocate the amounts at the aggregated level. In that case, the quintile (or other household group) allocation on the basis of the “unadjusted” micro data is taken as starting point and the amounts that have been attributed to the various causes are allocated to the quintiles. It is clear that the distributional results on the basis of this aggregated approach will not be as accurate as in the case of processing the corrections at the micro level, but in the end may lead to better results than simply applying a proportional allocation.
7.6. A framework to allocate the micro-macro gaps
To assist compilers in discussing possible reasons for the gaps and to allocate them to the relevant households (or household groups), a framework has been developed on the basis of the reasons expressed in the previous section. This framework consists of two parts. The first part focuses on assigning parts of the gap to possible underlying causes. This part is presented in Table 7.4. The first block (block I) in the table focuses on the derivation of the adjusted national accounts estimate for a specific item, starting from the national accounts total and adjusting for NPISHs, institutional households and expenditures of non-resident households on the territory respectively. The first column in this block shows the original estimates that were used to derive the adjusted national accounts figure. The second column provides the possibility to correct any of these original figures to close part of the gap between the micro and the macro results. The final result is presented in the third column.
The second block of the framework (block II) confronts the adjusted national accounts result with the micro aggregate, showing the gap between the two. The initial macro-micro gap is presented in the first column. The third column shows the gap that still remains after corrections have been made to the adjusted national accounts aggregate. This remaining gap still needs to be attributed to other reasons. This is done in block III. This block lists possible causes related to conceptual or classification differences, missing items or errors with regard to the micro data. In addition to the reasons presented in the previous section, it also contains an item for reasons that are not covered by the other categories. The block ends with the gap that still remains after attributing parts of the gap to the underlying reasons. Ideally, the amount of this remaining gap is zero, which would imply that the complete gap is explained by the various causes.
After the attribution of the macro-micro gap to the underlying causes, the related amounts should be allocated to the relevant household groups. Table 7.5 presents a framework for this step. Block IV focuses on the allocation on the basis of revised micro data, which, as was explained in the previous section, is the preferred option. In that case, corrections are processed at the micro level and new results are derived following the standard step-by-step approach. However, in some cases, this may be deemed too time-consuming or too complex. In those cases, corrections may be allocated at an aggregated level.9 This can be done in block V which provides the opportunity to allocate the remaining gaps at the quintile level (or other household groups depending on the targeted breakdown). Finally, block VI deals with allocating the remaining gap that could not be linked to any of the possible causes. The sum of the corrected micro data and the consecutive meso-corrections leads to the distributional results for the quintiles (or other household groups).
Results from two studies in which EG DNA members applied the framework to the five items that appear to be most relevant for their country showed that the allocation across quintiles indeed differs across the various reasons and that in most cases they differ from the distributions according to the micro data. The differences turned out to be particularly large for “measurement errors” and “underground activities”. The latter seemingly relates to the non-inclusion of underground economy in initial estimates and shows the importance of a separate estimation of these transactions. Furthermore, the results showed that estimation errors may significantly alter the distribution across households for specific items (e.g. food and non-alcoholic beverages; alcoholic beverages, tobacco and narcotics; transport; restaurants and hotels; and miscellaneous goods and services).10
Table 7.4. Framework for attributing micro-macro gaps to underlying causes
|
Item xx. |
Original estimate |
Correction |
Ultimate Estimate |
---|---|---|---|---|
I |
National account total (A) |
… |
… |
… |
|
- Adjustment for NPISHs (B1) |
… |
… |
… |
|
- Adjustment for institutional households (B2) |
… |
… |
… |
|
- Adjustment for expenditures of non-resident households on the territory (B3) |
… |
… |
… |
+ Adjustment for expenditure of resident households abroad (B4) |
… |
… |
… |
|
|
= Adjusted NA total (C=A-B1-B2-B3+B4) |
… |
… |
… |
II |
Micro total (D) |
… |
… |
|
|
= Macro-Micro gap (E=C-D) |
… |
… |
… |
III |
Conceptual or classification issues (F) |
… |
… |
… |
|
Underground and illegal activities (G) |
… |
… |
… |
|
Other elements missing in micro data (H) |
… |
… |
… |
|
Estimation errors (under-/overcoverage) (I) |
… |
||
|
Measurement errors (under-/overreporting) (J) |
… |
||
|
Reasons n.e.c. (K) |
… |
||
|
= Remaining gap (L=E-F-G-H-I-J-K) |
… |
Source: Zwijnenburg (2016[1]).
Table 7.5. Framework for allocating gaps to household groups
|
Item xx. |
Estimate |
Q1 |
Q2 |
Q3 |
Q4 |
Q5 |
---|---|---|---|---|---|---|---|
IV |
Allocation on the basis of micro data |
||||||
|
Original micro aggregate (P) |
… |
… |
… |
… |
… |
… |
|
Revised micro aggregate (Q) |
… |
… |
… |
… |
… |
… |
V |
Allocation on the basis of meso corrections |
||||||
|
Conceptual or classification issues (R) |
… |
… |
… |
… |
… |
… |
|
Underground and illegal activities (S) |
… |
… |
… |
… |
… |
… |
|
Other elements missing in micro data (T) |
… |
… |
… |
… |
… |
… |
|
Estimation errors (under-/overcoverage) (U) |
… |
… |
… |
… |
… |
… |
|
Measurement errors (under-/overreporting) (V) |
… |
… |
… |
… |
… |
… |
|
Reasons n.e.c. (W) |
… |
… |
… |
… |
… |
… |
VI |
Alignment of remaining gap (X=C-Q-R-S-T-U-V-W) |
… |
… |
… |
… |
… |
… |
|
Final estimate (Y=Q+R+S+T+U+V+W+X) |
… |
… |
… |
… |
… |
… |
Source: Zwijnenburg (2016[1]).
It is recommended that micro and macro experts regularly discuss the gaps between micro and macro results, particularly for the items that show the largest gaps, to find the most likely underlying reason(s), possibly reduce the gaps, and decide to which households these gaps most likely relate. Regular discussions will add to the awareness of these gaps and exchange of expertise may provide useful insights in how to deal with them. This will not only be relevant for projects in which micro and macro results are combined but would also be beneficial to properly explain to users why the results of micro and macro statistics on similar subjects may deviate.
References
[14] Accardo, J. et al. (2009), “Inequality between households in the national accounts”, No. 1265, INSEE, https://www.insee.fr/en/statistiques/1280710 (accessed on 21 September 2017).
[19] Bound, J. and A. Krueger (1989), “The extent of measurement error in longitudinal earnings data: do two wrongs make a right?”, NBER Working Paper Series, No. 2885, National Bureau of Economic Research, http://www.nber.org/papers/w2885.pdf (accessed on 30 October 2017).
[15] Carson, C. (1984), “The underground economy: An introduction”, Survey of current business, Vol. 64/5, pp. 21-37, https://bea.gov/scb/pdf/1984/0584cont.pdf (accessed on 9 January 2018).
[16] Cifaldi, G. and A. Neri (2013), “Asking income and consumption questions in the same survey: what are the risks?”, No. 908, Banca d’Italia, https://www.bancaditalia.it/pubblicazioni/temi-discussione/2013/2013-0908/en_tema_908.pdf?language_id=1 (accessed on 11 October 2017).
[13] Coli, A. and F. Tartamella (2014), “Using Administrative and Survey Data to Analyse Tax Evasion from Unregistered Labour”, http://www.iariw.org/papers/2014/ColiPaper.pdf (accessed on 24 October 2017).
[8] D’Alessio, G. and I. Faiella (2002), “Non-response behaviour in the bank of Italy’s survey of household income and wealth”, No. 462, Banca d’Italia, https://www.bancaditalia.it/pubblicazioni/temi-discussione/2002/2002-0462/tema_462_02.pdf?language_id=1 (accessed on 27 October 2017).
[12] D’Alessio, G. and A. Neri (2015), “Income and wealth sample estimates consistent with macro aggregates: some experiments”, No. 272, Banca d’Italia, https://www.bancaditalia.it/pubblicazioni/qef/2015-0272/QEF_272.pdf?language_id=1 (accessed on 27 October 2017).
[20] Gottschalk, P. and M. Huynh (2007), “Are earnings inequality and mobility overstated? The impact of non-classical measurement error”, http://www.sem.tsinghua.edu.cn/semcms_com_www/upload/home/store/2008/5/12/2767.pdf (accessed on 30 October 2017).
[10] Grilli, J., P. Engelbrecht and J. Zwijnenburg (2022), Pareto tail estimation in the presence of missing rich in compiling distributional national accounts.
[5] Johns, A. and J. Slemrod (2008), “The Distribution of Income Tax Noncompliance”, http://www.bus.umich.edu/OTPR/DITN%20091308.pdf (accessed on 30 October 2017).
[17] Lohmann, H. (2010), “Comparability of EU-SILC survey and register data: The relationship among employment, earnings, and poverty”, http://www.iariw.org/papers/2010/6bLohmann.pdf (accessed on 20 October 2017).
[2] Meyer, B., W. Mok and J. Sullivan (2015), “Household Surveys in Crisis”, NBER Working Paper Series, No. 21399, http://www.nber.org/papers/w21399 (accessed on 24 October 2017).
[7] Meyer, B., W. Mok and J. Sullivan (2009), “The under-reporting of transfers in household surveys: Its nature and consequences”, NBER Working Paper Series, No. 15181, http://www.nber.org/papers/w15181 (accessed on 27 October 2017).
[6] Neri, A. and R. Zizza (2010), “Income reporting behaviour in sample surveys”, No. 777, Banca d’Italia, https://www.bancaditalia.it/pubblicazioni/temi-discussione/2010/2010-0777/en_tema_777.pdf?language_id=1 (accessed on 11 October 2017).
[3] Pinkovskiy, M. et al. (2014), Lights, Camera,…Income! Estimating Poverty Using National Accounts, Survey Means, and Lights, Federal Reserve Bank of New York, https://www.newyorkfed.org/medialibrary/media/research/staff_reports/sr669.pdf (accessed on 26 September 2017).
[18] Romanov, D. and Y. Gubman (2012), “Well-Being and Measurement Error of Income Reported in a Social Survey versus Income Recorded by a Tax Administration”, http://www.iariw.org/papers/2012/RomanovPaper.pdf (accessed on 30 October 2017).
[9] Sabelhaus, J. et al. (2013), “Is the consumer expenditure survey representative by income?”, NBER Working Paper Series, No. 19589, http://www.nber.org/papers/w19589 (accessed on 27 October 2017).
[4] Törmälehto, V. (2017), “High income and affluence: Evidence from the European Union statistics on income and living conditions (EU-SILC)”, Eurostat Statistical Working papers, http://ec.europa.eu/eurostat/documents/3888793/7882117/KS-TC-16-027-EN-N.pdf (accessed on 9 October 2017).
[11] U.S. Bureau of Labor Statistics (2022), BLS Handbook of Methods: Consumer expenditures and income, https://www.bls.gov/opub/hom/cex/calculation.htm#calculation-methodology (accessed on 12 September 2023).
[1] Zwijnenburg, J. (2016), “Further Enhancing The Work On Household Distributional Data – Techniques For Bridging Gaps Between Micro And Macro Results And Nowcasting Methodologies For Compiling More Timely Results”, OECD, http://www.iariw.org/dresden/zwijnenburg.pdf (accessed on 21 September 2017).
Notes
← 1. This relates to the micro data for the items for which Method A (as described in Section 6.2) has been applied.
← 2. This relates to the items for which Method B, C or D (as described in Section 6.2) has been applied.
← 3. An alternative is to apply a proportional allocation of the gap, i.e. simply multiplying all micro data by the same factor to arrive at the macro aggregates. This would assume that all households misreport to the same degree. Whereas this may be a valid assumption if no other information is available (see also Section 12.3.1), it should only be applied as a last resort, i.e. after trying to allocate the majority of the gap on the basis of the most likely underlying reasons and to the most likely households concerned.
← 4. Corrections that relate to the adjusted national accounts totals will only affect the benchmark totals so only having an indirect impact on the distributional results.
← 5. In the Survey of Household Income and Wealth (SHIW) conducted by the Bank of Italy, it was found that respondents that are persuaded to participate after an initial refusal have average income and wealth that is 20% and 30% higher than the overall average. This was confirmed by a study in which data for a sample of 2000 households were matched with banking information. This also showed that non-response was not random, but more frequent among the wealthiest households.
← 6. See for example D’Alessio and Faiella (2002[8]) and D’Alessio and Neri (2015[12]) who have done research in which consistency of micro results within the same survey is checked.
← 7. A good example of such a consistency check is the way in which the French statistical office checks the data. They ask for information on income, consumption and financial well-being in their Household Budget survey, on the basis of which it is possible to adjust incomes on the basis of a coherence filter between income and consumption. Accardo et al. (2009[14]) explain that “when households declared an income which was very much lower than their everyday consumption expenditure (defined as consumption excluding major or exceptional purchases), yet without indicating that they felt they were in any financial difficulty, their income was aligned with the level of their consumption expenditure.”
← 8. Furthermore, Gottschalk and Huynh (2007[20]) explain that measurement error may be mean reverting, “in the sense that persons with low earnings tend to overstate their earnings and persons with high earnings understate their earnings” (see also Lohmann (2010[17])).
← 9. Bearing in mind that this is a sub-optimal solution as it does not take into account possible reclassification of households across household groups on the basis of corrected micro-data. Furthermore, it does not provide the possibility to take into account specific characteristics at the household level that may lead to more nuanced adjustments.