OECD Digital Education Outlook 2021
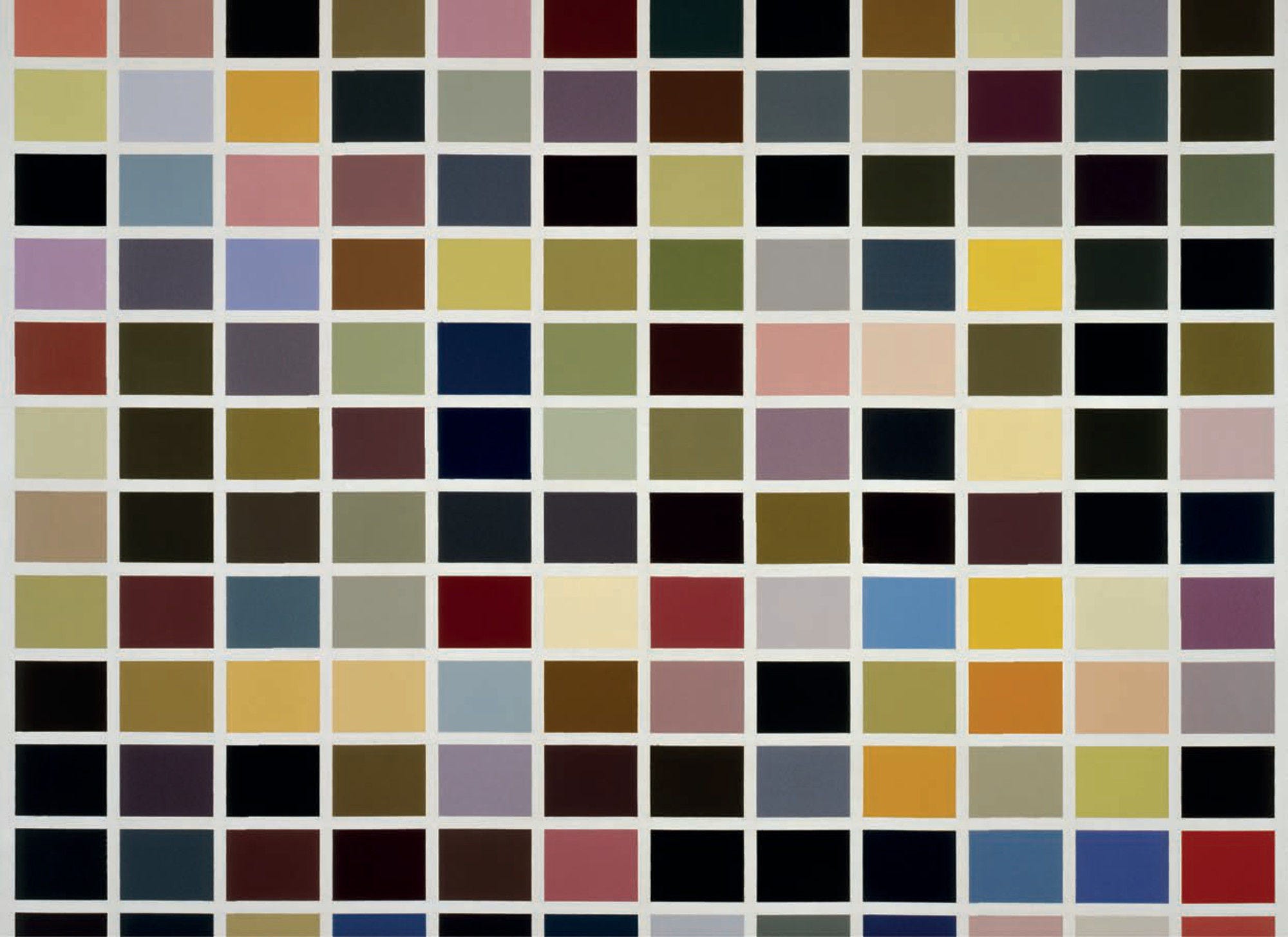
4. Improving student engagement in and with digital learning technologies
Abstract
Engagement is critical to learning, but it has proved challenging to promote both meaningful engagement and deep learning. Can digital learning technologies help? This chapter provides a broad overview of some promising paths to measure students’ level of engagement during learning with digital technologies and how these technologies can be designed to improve engagement on the onset of learning or when disengagement sets in. It discusses why engagement matters for learning, how to measure engagement with digital learning technologies, and presents different types of approaches in using data and technology to improve students’ engagement and learning.
Introduction
Improving sustained student engagement in learning has become a key objective of education for at least two reasons: (1) engagement is a pre-requisite to meaningful learning; and (2) maintaining engagement involves cognitive and socio-emotional skills that are learning objectives in themselves. Progress in digital technologies, including sensors, advanced data analytics techniques, and innovative digital learning experiences are opening new avenues for measurement, theory development, and design of pedagogical interventions that can help students stay engaged in their learning activities.
Over the last two decades, researchers and developers have made considerable progress in designing learning technologies to promote both engagement and learning. The aim of this chapter is to provide a broad overview of this emerging area and discuss some promising paths for the next decade or so. It will mainly focus on digital learning environments rather than traditional classroom settings, even though some of the developed technologies may soon be adapted for the classroom. At this stage, most of the digital learning technologies that have been developed to measure and promote sustained engagement have been tested in the context of research labs, but studies in real-world settings are gradually increasing. Thus far, results have been mixed, which is par for the course in such a nascent area.
Engagement is an easy concept to comprehend, but difficult to define. Therefore, after emphasising the importance of engagement for learning, the chapter discusses the challenges of scientifically defining engagement. Next, it provides an overview of current approaches to measuring engagement and then discusses how digital technologies and advances in computing methods and technologies afford rapid improvements in measuring it. With definition and measurement issues in place, the paper then shows different ways in which learning technologies have attempted to improve engagement and learning through proactive strategies and reactive interactions. These approaches, illustrated by examples and case studies, will give some ideas of what could soon become possible if not already widely used in digital learning environments. The paper concludes with a discussion of important next steps for this research programme and how it could transform education practice in the future.
The importance of engagement
The importance of engagement to learning has been recognised and investigated for decades. The research broadly supports the following general conclusion: a student who is engaged is primed to learn; a student who is disengaged is not. In particular, boredom, which is somewhat the antithesis of engagement, is not merely an unpleasant feeling. Boredom is associated with difficulties in maintaining attention (Danckert and Merrifield, 2018[1]; Eastwood et al., 2012[2]; Hunter and Eastwood, 2018[3]) and is consistently negatively correlated with learning outcomes (Pekrun et al., 2014[4]; Putwain et al., 2018[5]). For example, a recent meta-analysis on 29 studies (N = 19 052 students) found an overall significant negative effect r = -.24 of boredom on academic outcomes (Tze, Daniels and Klassen, 2016[6]). It is of course not necessary, and even unrealistic, to expect a student to be engaged 100% of the time; some amount of disengagement is likely to always occur and should not be problematic in small doses. However, sustained disengagement is associated with a host of negative outcomes including lower levels of achievement, lower self-efficacy, diminished interest in educational activities, partaking in high-risk behaviours, and most importantly, increased attrition and dropout from school (Baker et al., 2010[7]; Csikszentmihalyi, 1975[8]; Daniels et al., 2009[9]; Farrell et al., 1988[10]; Finn and Voelkl, 1993[11]; Griffiths et al., 2012[12]; Mann and Robinson, 2009[13]; Patrick, Skinner and Connell, 1993[14]; Pekrun et al., 2010[15]; Perkins and Hill, 1985[16]) (Wasson, 1981[17]). Put in a more positive light, being engaged in school is associated with several beneficial outcomes beyond academic achievement itself.
Much of the research on engagement has focused on traditional learning which occurs in the classroom and school settings. The Handbook of Research on Student Engagement (Christenson, Reschly and Wylie, 2012[18]) provides a detailed exposition of a range of issues pertaining to engagement in these learning contexts. However, with the advent of mobile devices, the Internet, and social media, much of learning occurs via digital media. This poses a challenge since it is particularly difficult to engage students when they interact with digital learning technologies, often in isolation. For example, Massive Open Online Courses (MOOCs) have achieved impressive outreach by opening up content to millions of learners worldwide. Yet, traditional MOOCs (xMOOCs), which mainly consist of watching videos, completing auto-graded assessments, and maybe participating in online discussions, have major problems with engagement and dropout (Yang et al., 2013[19]). Whereas a gifted human teacher or an expert tutor can design collaborative activities to increase engagement and can adapt the lesson when engagement appears to be waning, it is difficult for digital learning technologies to promote and sustain meaningful engagement for all learners. Even when a learning technology is successful at initially capturing students’ attention, they have little recourse when novelty fades, the student gets stuck, or boredom eventually sets in.
Designing digital learning experiences that promote both engagement and deep conceptual learning is a challenging task because it requires bridging the gap between learning and liking, which are often at odds. Herein lies the conundrum. On one hand, it is very easy to entertain students via puzzles, games, comics, and other “edutainment” gimmicks. Undoubtedly, students find these experiences to be very engaging, but it is unclear if they learn anything meaningful, especially at deeper levels of comprehension (Charsky, 2010[20]; Papert, 1998[21]). Further, approaches to increase interest by manipulating the learning materials (e.g. cover stories, very realistic images) can actually harm learning, presumably by detracting attention and cognitive resources away from the learning content (Rey, 2012[22]). On the other hand, several decades of research into the cognitive science of learning has yielded principles of effective learning, e.g. (Bransford, Brown and Cocking, 2000[23]; Karpicke and Blunt, 2011[24]; Roediger and Karpicke, 2006[25]) which can be implemented in learning technologies, such as intelligent tutoring systems (ITSs). However, despite the widely-documented learning benefits of intelligent tutoring systems e.g. (Steenbergen-Hu and Cooper, 2014[26]) and other technologies that implement deep learning principles (McNamara et al., 2006[27]), students find interacting with these technologies to be quite tedious (see (Baker et al., 2010[7]; Craig et al., 2008[28]) but also see (Rodrigo and Baker, 2011[29]) for an exception where intelligent tutoring systems were found to be more engaging than games). The central issue is that learning is hard because it takes considerable effort and practice to attain mastery (Ericsson, Krampe and Tesch-Römer, 1993[30]). It requires delaying gratification because it is much more rewarding (in the short term) to disengage from learning and engage in something more immediately rewarding, such as social media (Duckworth et al., 2019[31]). Of course, disengagement comes at a hefty price in the future as noted above.
Defining engagement
Unlike physical entities, such as temperature and mass, engagement in a conceptual entity (called a construct) that must be operationally (scientifically) defined. Consider, for example, four hypothetical middle-school students in a maths course.
Keisha attends her maths class each day, listens attentively, asks good questions, and completes her homework. If asked, she will say that maths is her least favourite subject, but she recognises that it is important for her future.
James frequently misses his maths class, tries to listen diligently when he does attend, but frequently catches himself zoning out. He completes about 50% of his maths homework and is ambivalent about maths and school in general.
Rafael attends every maths class, sits silently, never speaks, takes detailed notes, and always completes his homework. He enjoys maths but is really passionate about science since he wants to become a biologist.
Marcus is passionate about maths and plays maths games in his spare time. He attends every class but finds the material to be too easy, so he frequently gets bored, and attempts to find errors in what his teacher is saying as a way of passing the time. He tries to complete his maths homework every night but finds it too tedious and repetitive so he posts on social media instead.
Which of these students is engaged and which are not? Would a dictionary definition help? The Merriam-Webster dictionary defines engaged/engagement as “involved in activity” “greatly interested” and “emotional involvement or commitment.” However, as Eccles and Wang (2012[32]) note, these generic, definitions make the construct more accessible for policy makers and the educated lay person, but less useful for scientific research where precise definitions are of greater value, especially when it comes to elucidating cause and effect relationships.
Unfortunately, a scientific definition of engagement remains elusive. Reschly and Christenson (2012[33]) note that the term engagement has been used to describe diverse behaviours, thoughts, perceptions, feelings, and attitudes, and at the same time, diverse terms have been used to refer to similar constructs. Engagement has been considered to be a broad and complex construct pertaining to diverse aspects of the educational experience (e.g. showing up, completing homework, feelings of belonging, getting a good time) and across multiple time scales (e.g. momentary periods of interest, stable dispositions such as general disengagement with school, and life-altering outcomes like dropping out of school). Thus, it may be more fruitful to study specific aspects of this complex construct rather than striving for an all-encompassing, but overly generic definition.
Researchers generally agree that engagement is a multidimensional construct, although the number and nature of the dimensions are unclear (Figure 4.1). Fredricks, Blumenfeld and Paris (2004[34]) proposed three components of engagement in a highly influential article. Emotional engagement encompasses feelings and attitudes about the learning task or learning context, such as feelings of interest towards a particular subject, teacher (Renninger and Bachrach, 2015[35]), or general satisfaction with school. Behavioural engagement broadly refers to learners' participation in learning, including effort, persistence, and concentration. Cognitive engagement pertains to learners' investment in the learning task, such as how they allocate effort towards learning, and their understanding and mastery of the material.
Reeve and Tseng (2011[36]) recently suggested a fourth dimension: agentic engagement, characterised by learners proactively contributing to the learning process. Alternatively, Pekrun and Linnenbrink-Garcia (2012[37]) proposed a five-component model that includes cognitive (e.g. attention and memory processes), motivational (e.g. intrinsic and extrinsic motivation), behavioural (e.g. effort and persistence), social-behavioural (e.g. participating with peers), and cognitive-behavioural (e.g. strategy use and self-regulation) aspects of engagement.
Figure 4.1. Components of engagement
Source: D’Mello.
In addition to the components of engagement, the time course and the influence of contextual factors are also quite important. With respect to the time course, a fleeting interest in a topic that engages a student for a few minutes or a few hours is different from a student who sustains that engagement over months and years (Hidi and Renninger, 2006[38]). It is also widely acknowledged that the context in which an activity is situated has a major impact on the resultant patterns of engagement. Engagement is not an intrinsic property of individuals but emerges from interactions between individuals and their environments including their peers, teachers, family, and school structures (Christenson, Reschly and Wylie, 2012[18]).
Accordingly, Sinatra, Heddy and Lombardi (2015[39]) proposed a framework that integrates the components, time course, and contextual influences of engagement (Figure 4.2). The framework conceptualises engagement along a continuum, anchored by person-oriented perspectives at one extreme, context-oriented at the other, and person-in-context in between. Person-oriented perspectives focus on the cognitive, affective, and motivational states of the student at the moment of learning and are best captured with fine-grained physiological and behavioural measures (e.g. response patterns, facial expressions). The context-oriented perspective emphasises the environmental context as the analytic unit. Here, the focus is on macro-level structures like teachers, classrooms, schools, and the community, rather than the individual student. The intermediate-grain size, person-in-context perspective focuses on the interaction between student and context (e.g. how students interact with each other or with technology). This level of analysis would examine, for example, whether particular classroom activities (e.g. small group work) are more engaging than others (e.g. a lecture).
Figure 4.2. Time course of engagement and influence of context
Source: D’Mello.
When one focuses on digital learning, engagement can be operationally defined as a goal-directed state of active and focused involvement with a digital learning technology. This operationalisation aligns with the person-oriented level of analysis of Sinatra et al. (2015) in that the focus is on the state (not trait) of the affective, behavioural, and cognitive components of engagement across short time scales ranging from seconds to minutes. In most cases, boredom, drowsiness, and excessive zoning out are signs of disengagement whereas interest, curiosity, and the so-called “flow experience,” where a learner is so engrossed in an activity that time and space disappear (Csikszentmihalyi, 1990[40]), would signal engagement. However, in some cases, the specific mental states (and their levels) associated with engagement vary based on the affordances of the learning technology. For example, despite being a negative emotion, frustration while playing a difficult educational game might indicate that the student is engaged as it is part and parcel of the “hard fun” of learning from games (Gee, 2003[41]), whereas frustration during a simple vocabulary learning task might signal something other than engaged learning.
Measuring engagement
The effectiveness of any approach to improve engagement depends on the validity of the measurement of engagement. So how has engagement been traditionally measured? Figure 4.3 provides an overview of the various measurement approaches.
Traditional measurement approaches
The most widely used measures of engagement in both traditional and digital education are self-report questionnaires (Fredricks and McColskey, 2012[42]; Greene, 2015[43]; Henrie, Halverson and Graham, 2015[44]). Here, students are asked to endorse items such as “When I am in class, I listen very carefully” (an item for behavioural engagement) or “I enjoy learning new things in class” (an item for emotional engagement). Although relatively inexpensive, easy to administer, and generally reliable, questionnaires have well-known limitations (Duckworth and Yeager, 2015[45]; Krosnick, 1999[46]). For instance, when endorsing items, respondents must compare the target (e.g. a student rating himself or herself) to some implicit standard, and standards may vary from respondent to respondent. To one student, “I am engaged in my maths class” may be exemplified by doing five hours of homework each day; for others, the same statement may be exemplified by simply showing up for class. Thus, biases that arise from heterogeneous frames of reference reduce the validity of self-report questionnaires (Heine et al., 2002[47]). Social desirability bias is another important limitation (Krosnick, 1999[46]), both when respondents aim to appear admirable to others and also when they inflate responses to preserve their own self-esteem. Likewise, memory recall limitations and acquiescence bias can influence self-report questionnaires (Podsakoff et al., 2003[48]).
Several non-questionnaire engagement measures have also been developed. Examples include experience-sampling methods (ESM) (Csikszentmihalyi and Larson, 1987[49]), day reconstruction (Kahneman et al., 2004[50]), and interviews (Turner and Meyer, 2000[51]). However, because they still rely on self-reports, they are subject to some of the same biases as questionnaires. For example, ESM is subject to social desirability biases but not to memory recall biases.
Observational methods are an attractive alternative to self-reports because they are more objective (Nystrand and Gamoran, 1991[52]; Pianta, Hamre and Allen, 2012[53]; Renninger and Bachrach, 2015[35]; Ryu and Lombardi, 2015[54]; Volpe et al., 2005[55]). Unfortunately, these methods entail considerable human effort, which poses a significant challenge for repeated long-term measurement at scale. Further, observations cannot be conducted in some learning contexts, such as students’ homes. Some of the limitations may be addressed by combining automated data collection with semi-automated or manual data coding. For example, the Electronically Activated Recorder (EAR) randomly samples audio clips in naturalistic environments (Mehl et al., 2001[56]). Data collection with the EAR is efficient and cost-effective; however, the data still need to be transcribed and coded by humans, which increases cost and reduces scalability. Similarly, engagement can be coded from videos by researchers (Lehman et al., 2008[57]) or even teachers (D’Mello et al., 2008[58]), but video coding is a labour- and time-intensive effort. To address this limitation, there have also been some attempts to automatically analyse classroom video to infer aspects of student engagement e.g. (Aung, Ramakrishnan and Whitehill, 2018[59]; Bidwell and Fuchs, 2011[60]; Klein and Celik, 2017[61]; Raca, Kidzinski and Dillenbourg, 2015[62]), though research is still in its infancy partly due to the difficulty of recording classroom video and related privacy concerns.
Finally, engagement can be adduced from academic and behaviour records, such as homework completion, absences, achievement test scores, time spent on a digital learning platform, and so on (Arnold and Pistilli, 2012[63]; Lehr, Sinclair and Christenson, 2004[64]; Skinner and Belmont, 1993[65]), but these measures are limited in what they can reveal about the cognitive and affective components of engagement. For example, in the technology world, engagement is often equated with usage, and is quantified as the number of logins, clicks, and so on. This characterisation, which only captures a few overt behaviours, is clearly insufficient because it focuses on only one dimension (behavioural) of a multidimensional construct (see above).
An automated digital measurement approach
Scientific advances on understanding engagement and interventions to increase it are limited by measurement approaches that are either costly or have known biases and limitations. Improving engagement in the context of digital learning is first and foremost a measurement and theoretical challenge that advances in sensors and digital technologies can help address. Among the most policy-, practice-, research-, and design-relevant finding from recent reports on engagement and other similar factors (Atkins-Burnett et al., 2012[66]; Shechtman et al., 2013[67]) is the overuse of and overreliance on student self-report measures, which limits current findings and theory even when produced by rigorous research designs and large sample sizes by the education research, design, policy, and practice communities. In the past and currently, student self-report data informs theory and programme interventions, which use student self-report measures to evaluate programmes and influence theory. Advanced digital technologies and data analytics methods allow one to break the cycle and advance our understanding of engagement systematically and significantly by going beyond the use of student self-report measures only. Reliable, valid, fair, and efficient measures collected from multiple sources and analysed by trained researchers applying methods and techniques appropriately will make the entire value of research studies and programme evaluation more productive.
D’Mello, Dieterle and Duckworth (2017[68]) recently proposed the advanced, analytic, automated (AAA) approach to measure engagement as an alternative method especially suited for interactions with digital learning technologies. This AAA-approach focuses on a person-oriented operationalisation of engagement as the momentary affective and cognitive states that arise throughout the learning process (i.e., it is consistent with the working definition provided above). AAA-based measures have several advantages over counterparts. For one, they are automated, which indicates that they can be applied widely and at scale. Second, they are more consistent because computers provide the measurements, thereby partially obviating reference, social desirability, acquiescence, and other biases associated with self- and observer- reports. These measures are also unaffected by momentary lapses in attention or by fatigue, as can occur with humans. They vastly reduce time and effort, which is a limitation of ESM, day reconstruction, video coding, and human observations.
Figure 4.3. Major categories of measures of engagement with examples (last row)
Source: D’Mello.
The core idea of the AAA-approach (Figure 4.4) is to train machines to infer latent mental states associated with engagement (e.g. concentration, interest) from machine-readable signals and from aspects of the environmental context. The approach uses machine learning which requires training data to learn a computational model (computer programme) that can be applied on data collected in the future (unseen data). Accordingly, the AAA measurement approach, which begins with collection of training data.
Step 1 consists of recording signals (video, physiology, log files, etc.) as students complete a learning activity within a particular learning context (Step 1a), followed by computing higher-order representations (called features) from the signals (Step 1b). A video is an example signal, from which features such as smiles, nods, frowns, etc., can be automatically extracted using computer vision techniques (Valstar et al., 2012[69]). Patterns of students’ interactions (i.e., click-streams) with learning technologies provide another powerful signal into student engagement (Baker and Ocumpaugh, 2015[70]).
In Step 2, annotations of mental states reflecting various components of engagement are obtained from the students themselves, from external observers, or via some other method (see (Porayska-Pomsta et al., 2013[71]) for a review). As an example, students can be asked to self-report their level of interest every five minutes (Sabourin and Lester, 2014[72]) or a trained human observer can watch the ensuing interaction and code students’ perceived affect in five-minute intervals (Ocumpaugh, Baker and Rodrigo, 2015[73]). The features and annotations need to be temporally synchronised so that there is an association among the two. For example, the number of mouse clicks and smiles in each five-minute interval can be aligned to the self- or observer- reports of engagement in the same interval.
Step 3 in the AAA measurement approach involves supervised learning (a machine learning subfield), which learns to associate features (extracted from signals recorded by sensors as noted above) with temporally synchronised annotations of mental states (e.g. from self-reports or observer judgments) collected at multiple points in a learning session and/or from multiple students ideally in varied contexts. The output of supervised learning is a computational model (or a computer programme) which produces computer-generated annotations, which replaces the human-provided annotations. As summarised below:
1. features + human-provided annotations → computational model
2. features + computational model → computer-generated annotations
Figure 4.4. Overview of the AAA-approach.
In Step 4, the computer-generated annotations are compared to the human-provided annotations to validate the model. The aim is for computer-generated annotations to match the human-provided annotations (accuracy) when applied to new or unseen data, for example, from a different set of students (generalisability). Once the model has been sufficiently validated, it can be deployed. Based on the sensor-collected data at some future time and/or from a new set of students, the model automatically generates estimates (annotations) of engagement. Figure 4.4 presents an overview of the approach.
It is important to consider privacy concerns for measures that use biometric signals (e.g. an image of a face, an audio sample). Some sensors can also inadvertently record sensitive information, as in the “WebcamGate” scandal where school authorities in a U.S. district remotely accessed students’ school-issued laptops to take pictures of students in their own homes, their chat logs, and information on the websites they visited without informing students or parents (Martin, 2010[74]). An effective strategy to protect privacy is to only retain non-identifiable features from the signals while discarding the signals themselves as done in (Bosch and D’Mello, 2019[75]). In addition to privacy, there are important concerns pertaining to bias and fairness of the underlying models so it is important that training data is representative of different subgroups and the models demonstrate equivalent performance across subgroups (REFS) (Gardner, Brooks and Baker, 2019[76]; Jensen, Hutt and D’Mello, 2019[77]). There are also ethical concerns with respect to how the measures are used. Their use for teacher or student evaluation is not recommended since the measures are imperfect and engagement is influenced by factors out of the control of teachers and students. Continual use is also not recommended as this can result in students rightly feeling monitored by these technologies – a particular concern for historically marginalised student populations. As we elaborate in the next section, these measures are best used in efforts to improve learning technologies, either by passively measuring periods of disengagement for retrospective review and refinement e.g. (Miller et al., 2014[78]) or by dynamically re-engaging disengaged students (D’Mello et al., 2016[79]; De Falco, Baker and D’Mello, 2014[80]). Their usage should be limited and ideally based on opt-in consent by students and caregivers.
Examples of engagement measures with the advanced, automated, analytic approach
D’Mello, Dieterle and Duckworth (2017[68]) provide examples of 15 studies that have utilised the AAA-approach to measure engagement across a range of learning technologies (e.g. intelligent tutoring system, educational game), learning domains (e.g. algebra, biology), student populations, operationalisations of engagement, methods used to obtain human annotations, supervised classification methods, and validation methods. They organised their review based on the sensor used for measurement. Sensor-free measures analyse digital traces recorded in log files e.g. (Bixler and D’Mello, 2013[81]; Gobert, Baker and Wixon, 2015[82]; Hutt, Grafsgaard and D’Mello, 2019[83]; Pardos et al., 2013[84]), while sensor-based measures use physical sensors. The latter can be further categorised as sensor-light if they use sensors that are readily available in contemporary digital devices, such as webcams and microphones e.g. (Bosch and D’Mello, 2019[75]; Bosch et al., 2016[85]; Forbes-Riley and Litman, 2011[86]; Monkaresi et al., 2017[87]; Pham and Wang, 2015[88]; Whitehill et al., 2014[89]) or sensor-heavy if they require non-standard sensors like eye trackers e.g. (Bixler and D’Mello, 2016[90]; Conati, Aleven and Mitrovic, 2013[91]; Hutt et al., 2019[92]), pressure pads e.g. (D’Mello and Graesser, 2009[93]; Mota and Picard, 2003[94]), and physiological sensors e.g. (Blanchard et al., 2014[95]; Dindar et al., 2017[96]; Mills et al., 2017[97]). Currently, sensor-free approaches are the most viable for learning technologies, but sensor-light approaches are gaining prominence and are expected to be major contenders in the next decade. Some research has also combined the two e.g. (Bosch et al., 2015[98]; D’Mello and Graesser, 2010[99]; Grafsgaard et al., 2014[100]; Kapoor and Picard, 2005[101]). Some examples of AAA-based engagement measures are listed below.
Interaction patterns to detect disengagement from task goals
Gobert, Baker and Wixon (2015[82]) developed an AAA-based measure for Inq-ITS, a computer-based learning environment with intelligent tutoring system to help students develop scientific inquiry skills. They focused on detecting if learners were disengaged from the task goal (DTG), defined as “engaging with the task, but in a fashion unrelated to the learning task’s design goals or incentive structure” (p. 48). They collected training data from 144 middle-school U.S. students who used Inq-ITS as part of their science classes. Two humans coded disengagement from the task goal from human-readable excerpts (called clips) of Inq-ITS log files. Features used for supervised classification included the total number of actions, time between actions, duration of the longest pause, and number of simulations run. The researchers obtained moderate accuracies (about 41% above guessing) in discriminating disengagement from the task goal from clips with no disengagement from the task goal, highlighting the utility of the approach.
Eye-gaze to detect mind-wandering (zoning out)
Hutt et al. (2019[92]) used commercial off-the-shelf (COTS) eye trackers to track mind-wandering when high-school students completed tutorial sessions with a biology intelligent tutoring system called GuruTutor (Olney et al., 2012[102]) in their regular biology classroom (Figure 4.5). They asked students to self-report mind-wandering by responding to a question asking whether they were thinking about the learning context or something else entirely throughout the learning session. They computed eye gaze features (e.g. number of fixation, fixation durations, blinks) in 30-second windows preceding each probe and trained supervised classifiers to discriminate between positive and negative probe responses from the gaze features. The models were very successful in detecting mind-wandering, with accuracies more than double that of chance (guessing). Importantly, the models’ predictions of mind-wandering negatively correlated with learning outcomes similar to self-reported mind-wandering. The researcher embedded the models into GuruTutor to provide real-time mind-wandering estimates for evaluation and to drive interventions (see reactive approach below).
Figure 4.5. Using consumer-grade eye tracker (left) to monitor visual attention while students interact with Guru (right) in classrooms
Facial features, body moments, and interaction patterns to detect affect
Bosch et al. (2016[85]) developed an AAA-based measure of engagement while students played an educational game called Physics Playground (Shute, Ventura and Kim, 2013[103]); the game is described in more detail in the next section. They collected training data from 137 8th and 9th grade U.S. students in two 55-minute sessions across two days. Trained observers performed live annotations of boredom, engaged concentration, confusion, frustration, and delight using an observation protocol (Ocumpaugh, Baker and Rodrigo, 2015[73]), which were synchronised with videos of students’ faces and upper bodies. The researchers extracted body movement and various facial expressions (e.g. smiles) from the videos and combined them with interaction features (e.g. number of restarts) extracted from the game log files. Supervised learning models trained to discriminate each affective state from the others (e.g. boredom vs. confusion, frustration, engaged concentration, and delight) yielded moderate accuracies (about 37% improvement over chance).
A sensor-free approach to measuring engagement during online learning
The above three examples highlight a variety of AAA-based engagement measures. However, these and all extant measures have been developed using data from a small number of students collected over one or two class sessions. As such, they serve as a proof-of-concept of the overall idea, but may not be sufficiently robust for real-world use. In contrast, Hutt, Grafsgaard and D’Mello (2019[83]) used the AAA-approach to develop a sensor-free measure of student engagement with an eye for scalability to tens of thousands of diverse students across extended time frames of an entire school year and beyond. The research was conducted in the context of Algebra Nation (Figure 4.6), an online maths learning platform that supports over 150 000 students studying Algebra 1, Algebra 2, and Geometry. For each topic, students can watch a video lecture from one of several human tutors. They can also use the Test Yourself quiz feature, which randomly selects 10 questions aligned with state standards. Students can review feedback on their answers or watch solution videos. Lastly, students can get more help through the Discussion Wall where they can interact with other students and study experts hired by Algebra Nation. Students can earn karma points by answering questions posted by other students.
The researchers collected a large-scale dataset of 69 174 students who used Algebra Nation as part of their regular maths classes for a semester. They used experience sampling to collect 133 966 self-reports (on a 1 to 5 scale) of 18 mental states (e.g. boredom, confusion, mind-wandering, curiosity, interest) related to engagement. They computed generic activity features (e.g. viewing a video, pausing a video taking a quiz) extracted from Algebra Nation log files; these features do not require specialised sensors and are domain- and (to a certain extent) system-independent. In all, they counted the occurrence of 22 such features in 5-minute windows prior to a self-report survey. They trained supervised learning models to predict each affective state from the features. Prediction accuracies, quantified with Spearman’s rho (a correlation coefficient ranging from -1 to 1), were modest and ranged from .08 (for surprise) to .34 (for happiness), with a mean of .25.
The researchers tested the generalisability of the engagement models in several ways. First, they showed that the models trained on Algebra students generalised to a different data set of Geometry students (n = 28 458) on the same platform. They also studied the models’ generalisability to clusters of students based on typical platform use and demographic features. They found that models trained on one group performed similarly well when tested on the other groups, although there was a small advantage of training individual subpopulation models compared to a general (all-population) model.
These results show the promise of scaling up sensor-free AAA-based engagement detection on the largest and most heterogeneous student sample to date using generic activity features that are not specific to a particular domain or system. The models have been embedded in the Algebra Learning platform, where they are being used as a component of a personalised system that recommends activities to students based on their ability and level of engagement (from the current models). The study is ongoing so the effectiveness of the approach awaits further research developments.
Figure 4.6. Sample video (left) in the Algebra Nation platform along with a self-report engagement questionnaire (right)
Improving engagement
Learning technologies have traditionally mainly focused on promoting knowledge and skills. The unstated assumption underlying this approach is that cognition is all that matters, or at the very least, what really matters. Accordingly, emotion and motivation have been relegated to secondary design considerations, if considered at all. This was a reasonable assumption in the early days of learning technologies given that the dominant learning theories of the time put a premium on knowledge and skill acquisition e.g. (Anderson, 1982[105]; Brown and VanLehn, 1980[106]; Sleeman and Brown, 1982[107]). The benefit of hindsight tells us that this assumption is problematic since students need to be engaged to learn and it is very difficult to engage students with these traditional learning technologies. For example, intelligent tutoring systems (ITSs), which emulate one-on-one instruction of human-human tutoring, are effective in promoting learning for meta-analyses, see (Ma et al., 2014[108]; Steenbergen-Hu and Cooper, 2013[109]; Steenbergen-Hu and Cooper, 2014[26]), yet students experience high levels of boredom while learning from intelligent tutoring systems (Craig et al., 2004[110]; D’Mello, 2013[111]; Hawkins, Heffernan and Baker, 2013[112]). Further, the basic functioning of the human cognitive system makes it difficult to sustain attention – a core component of engagement – even when one is motivated to do so. For example, students experience lapses in attention in the form of “zone outs” around 30% of the time during learning from technology (D’Mello, 2019[113]). Whereas some amount of zoning out and other forms of disengagement are normal occurrences in learning, higher amounts of zoning out is negatively related with learning outcomes (Randall, Oswald and Beier, 2014[114]; Risko et al., 2013[115]).
Can we design learning environments that are more sustainably engaging and thus enhance students’ learning? It is only within the past two decades that researchers have made a concentrated effort to design for engagement (del Soldato and du Boulay, 1995[116]). Researchers have converged upon two main strategies to improve engagement in learning technologies: designs that promote engagement from the ground-up (proactive) or embedding mechanisms to monitor engagement in real-time and dynamically intervene when disengagement occurs or is imminent (reactive). It is also possible to combine the two approaches.
Proactive approaches
Proactive digital learning technologies are specifically designed to promote engagement and learning. Such systems aspire to increase the likelihood that the learner will experience cognitive and affective states which are generally positively associated with engagement (e.g. interest, curiosity, deep reasoning, critical thinking, being alert), while decreasing those which are usually negatively associated with engagement (e.g. boredom, zone outs, shallow processing).
It is important to distinguish between subtle or light-touch approaches that elicit mild engagement versus attempts to deeply engage students. For example, gamification – including elements of games into traditional learning technologies via points, challenges, badges, leaderboards and the like (Gibson et al., 2015[117]; Kapp, 2012[118]), see Box 4.1 as an example – can mildly increase engagement in the short term, but is unlikely to sustain deep engagement over extended time frames. Students might also resent the so-called “chocolate-covered broccoli” feel to some gamification attempts. Another subtle approach is emotional design, which involves altering digital learning materials to induce mild positive affect (e.g. adding facial anthropomorphisms to non-human graphical elements and/or adding pleasant colours in digital media) (Plass et al., 2014[119]; Um et al., 2012[120]). A recent meta-analysis (Brom, Stárková and D’Mello, 2018[121]) indicated that emotional design was surprisingly effective in increasing learning while also enhancing engagement as measured by intrinsic motivation, liking/enjoyment, and positive affect.
Box 4.1. Encouraging good behaviour and social learning with video games in Canada and the United States
Fans of the Harry Potter series understand the significance of earning points for student behaviour. Teachers at Hogwarts school of witchcraft and wizardry award and take away points to reward or punish students for their behaviour. Students can earn points for their House by doing good deeds, answering questions correctly, and of course by winning Quidditch matches. By doing the opposite, students may lose points (and disappoint their fellow students in the same House). At the end of the school year, the House with most points wins the prestigious House Cup.
Real life may lack the savvy magic tricks of Hogwarts’ teachers, but Québec- and New York-based company Classcraft tried to replicate engagement in learning with similar methods. Inspired by video games such as World of Warcraft, its cloud-based platform for secondary education was described as a “role-playing game for classroom management” (Sanchez, Young and Jouneau-Sion, 2016[122]).
Classcraft aims to help students learn about appropriate behaviour, like doing homework and not being late for class. The game is not related to any specific subject; the duration of a game can last from just one class to an entire school year. Classcraft is foremost a web application for use in class, although students can download the application on their mobile phones for use outside class too. Unlike most video games, Classcraft does not offer a 3D game world. Instead it offers a form of augmented reality: by adding a digital layer to the real world, the game creates new interactions between teachers and students.
Students play in teams of 4 to 6 students. At the beginning of the game, they each get to choose different avatars (healers, mages, warriors) with their own strengths and weaknesses. Their goal is to gain experience points and prevent their avatars from losing health points. By acting according to the criteria for appropriate behaviour, they earn points which they can for example use to upgrade their avatar or to help their teammates. Doing the opposite can of course also lead to losing points, which may affect the entire team. Teachers operate as “game masters”: they distribute or remove points, and they can create certain random events in the game or certain dynamics in real life (like asking to fulfil specific tasks) which affect all students. Updates in the game appear in real-time in every player’s application. Interaction with real life is at the core of the model: winning or losing virtual points can lead to teacher actions in real life, including praise, rewards, and perhaps even reprimands. Because the game also connects the health points of one student with other students in the same team, students are encouraged to work together. The use of Classcraft – as well as comparable software programmes for classroom management – may have a positive impact on student engagement, collaboration, and even on the school climate (Edweek).
Promoting deep sustained engagement requires a fundamental reconceptualisation of the learning experience. The Interactive-Constructive-Active-Passive (ICAP) framework (Chi and Wylie, 2014[123]) provides a useful starting point. ICAP proposes four levels of engagement and learning based on the level of interactivity afforded by the learning activity. The levels, in decreasing order of expected engagement and learning, are Interactive > Constructive > Active > and Passive (Figure 4.7). An example of passive activity would include viewing a lecture or video with no overt behaviour, whereas taking verbatim notes without adding new ideas or organisation would be considered active. Generating a summary or self-explanation of lecture content by adding new ideas or reorganising old ideas would qualify as constructive. Interactive activities including some form of interaction or dialogue that accompany a constructive activity, for example, reciprocal peer tutoring, where students take turns tutoring each other. According to ICAP, one strategy to promote both engagement and learning is to incorporate more constructive and interactive experiences in learning technologies.
Figure 4.7. Organisation of activities with respect to expected engagement and learning (from left to higher) as per the ICAP framework
Source: D’Mello.
Educational games are one example of such learning technologies (Gee, 2003[41]). Well-designed educational games can enhance engagement and learning by turning work into play by minimising boredom, optimising engagement/flow, presenting challenges that are achievable with thought, creativity, and effort, and by engineering delight and pleasant surprises (Lepper and Henderlong, 2000[124]; Plass, Homer and Kinzer, 2015[125]; Ritterfeld, Cody and Vorderer, 2009[126]). Designing educational games can be quite challenging because game designers must balance a trade-off between game environments that are engaging but tangential to learning, and environments that promote deep learning but fail to foster engagement (Johnson and Mayer, 2010[127]). Well-designed games balance these goals by incorporating theoretically-grounded principles (problem solving, rules/constraints, challenge, control, ongoing feedback, and sensory stimulation see (Shute et al., 2014[128])) that make them intrinsically motivating and thus engaging; (Fullerton, Swain and Hoffman, 2008[129]; Malone and Lepper, 1987[130]; Shute, Rieber and Van Eck, 2011[131]).
Well-designed games also directly embed meaningful learning experiences throughout gameplay. As such, playing well-designed games has been shown to be positively related to various learning competencies and outcomes such as visual-spatial abilities and attention (Green and Bavelier, 2012[132]; Green and Bavelier, 2007[133]; Shute, Ventura and Ke, 2015[134]), college grades (Skoric, Teo and Neo, 2009[135]) (Ventura, Shute and Kim, 2012[136]), persistence (Ventura, Shute and Zhao, 2013[137]), creativity (Jackson et al., 2012[138]), and civic engagement (Ferguson and Garza, 2011[139]), as well as valuable academic content and skills (e.g. (Coller and Scott, 2009[140]; DeRouin-Jessen, 2008[141]); for a review, see (Tobias and Fletcher, 2011[142]; Wilson et al., 2009[143]; Young et al., 2012[144]).
Two examples: Physics Playground and Crystal Island
As an example, consider Physics Playground (Shute, Ventura and Kim, 2013[103]), a highly engaging educational game for learning Newton’s laws of force and motion, linear momentum, energy, and torque (Figure 4.8). The game obeys the basic rules of physics via a formal simulation of a virtual physics “world” and dynamically responds to players’ interactions with the game. The primary goal is for players to guide a green ball to a red balloon, resulting in “solving” the level, and to do so, players must create agents – ramps, pendulums, levers, and springboards – that “come to life” on the screen. Game elements in Physics Playground include realism (accomplished via detailed formal simulation of a virtual physics “world”), ongoing feedback, interactive problem solving, and adaptive challenges. The game also gives players the freedom to try/fail by experimenting with a variety of solutions. Physics Playground is successful at increasing both engagement and learning by integrating physics concepts with authentic gameplay. Box 4.2 shows that the game also provides a good environment to research the relations between students’ emotions and learning internationally.
Figure 4.8. Example of a problem and pendulum solution in Physics Playground
Game-based learning can be further enhanced by increasing the immersion and realism of the experience. In traditional classroom settings, project-based learning (PBL) provides students or student teams with authentic, personally-meaningful, real-world problems over an extended period of time (Blumenfeld et al., 1991[145]). For example, student teams might take on the challenge of improving the water quality in their community; this requires an understanding of the water system, pollutants, purification, policy, chemistry, and so on. Thus, in addition to keeping students engaged through choice, realism, and collaboration, well-designed PBL curricula can help students acquire content knowledge along with real-world skills (e.g. inquiry, collaboration, scientific reasoning; see (Schneider et al., 2020[146])). Unfortunately, implementing these learning experiences require extensive curricula, access to physical resources, and human scaffolding, and are difficult to realise via digital technologies alone. To address this challenge, researchers have explored the use of augmented and virtual reality to develop immersive digital learning experiences for students. Immersion is defined as the subjective perception of experiencing reality – akin to a suspension of disbelief – and is hypothesised to increase engagement and learning (Dede, 2009[147]). Examples include River City (Dede, 2009[147]), Quest Atlantis (Barab et al., 2005[148]), and Crystal Island (Sabourin and Lester, 2014[72]), which is discussed briefly below.
Crystal Island (Rowe et al., 2009[149]; Spires et al., 2011[150]) is an immersive educational game that capitalises on the principle of narrativity, which posits that learners benefit most from educational games that weave a narrative theme into the game experience. In Crystal Island, the learner takes on the role of a protagonist named Alex who arrives on the island. Alex discovers that members of a research team are falling ill and is charged with identifying the source of an infectious disease. Alex proceeds with the investigation by visiting various areas of the island (dining hall, lab, inferminary, dorm), by interviewing other islanders, and by manipulating objects in the world. Through the generation of questions and hypotheses, and the collection and analysis of data, learners make gradual steps towards diagnosing the cause of the disease. Thus, Crystal Island embeds learning of microbiology knowledge and development of critical thinking and inquiry learning skills in an engaging narrative platform.
Evidence from learners’ interactions with Crystal Island indicate that it is highly engaging and motivating and can improve learning outcomes (Rowe et al., 2009[149]; Rowe et al., 2010[151]). For example, Sabourin and Lester (2014[72]) conducted a study in which 450 8th grade students interacted with Crystal Island for 55 minutes. Students self-reported one of seven affective states (anxious, bored, confused, curious, excited, focused, and frustrated) at five-minute intervals during gameplay. The researchers found that self-reports of boredom (8%) were considerably lower than reports of excitement (13%) and curiosity (19%). Confusion (16%) and frustration (16%) were also quite frequent, suggesting that the game was deeply engaging students as these affective states are frequent in deep learning activities (D’Mello and Graesser, 2012[152]). In addition, students demonstrated significant learning gains of about 30%, assessed as the percent improvement of post-test over pre-test scores.
Box 4.2. Using digital environments to research learning emotions in the Philippines
Physics Playground was used in the Philippines to analyse the positive or negative relationships of different affective states to learning, including frustration, the affective state expressing annoyance and dissatisfaction. Using a standardised human-based codifying system for students’ emotions while playing the game, and a different task (puzzle) than the one described in the main text, Banawan, Rodrigo and Andres (2015[153]) found that, after engaged concentration (79% of observed affective states), frustration (8%) was the second most experienced state among the 8 that were monitored, and the only one that had a statistical significant relationship with achievement – a negative relationship. The research shows that frustration does not behave like confusion, which can be positively related to achievement once solved. It also highlights the contextual nature of engagement, as Filipino students did not experience the same levels of enjoyment as the U.S. students using Physics Playground. This shows the importance of designing digital learning environments that remain sensitive to possibly different ways to deal with failure in learning and designing the right scaffoldings to help students cope with their negative feelings.
Recent advances in scalable augmented and virtual reality technologies have further closed the gap between the virtual and physical world (for a review, see (Martín-Gutiérrez et al., 2017[154])), especially in terms of immersion and realism (Psotka, 1995[155]). Despite the enthusiasm for these technologies, and some initial promising results (e.g. (Ibáñez et al., 2014[156])), there has yet to be rigorous research studies evaluating their learning effectiveness, particularly when it comes to conceptual rather than procedural or rote learning.
Reactive approaches
Consider the following hypothetical scenarios to motivate the reactive approach to improving engagement: “Imagine you are helping your niece prepare for an upcoming examination in evolutionary biology. Things started off quite well, but after a while, you realise that her mind is a million miles away. Although the plan is for the two of you to collaboratively model genetic frequency shifts in populations, you notice that her attention has drifted to unrelated thoughts of lunch, the football game, or an upcoming vacation. You might try to momentarily reorient her attention by asking a probing question. However, if her attentional focus continues to wane, you realise that you must adapt your instruction to better engage her by altering the course of the learning session. You shift the initiative from a collaborative discussion to a student-centred perspective by asking her to develop a strategy for tracking genetic changes in populations. This works and she appears to tackle this task with a renewed gusto and the session progresses quite smoothly. However, sometime later, you notice that she actually appears to be nodding off as you delve into the fundamentals of allele frequencies. So, you suggest switching topics or even taking a break, thereby giving her an opportunity to recharge.”
The example above provides a sense of what a reactive agent – a human in this case – can accomplish. Reactive approaches to increasing engagement focus on automatically detecting student engagement and dynamically responding when engagement appears to be declining, or providing motivating feedback when engagement is high (D’Mello and Graesser, 2015[157]). These approaches assume that engagement is a fluid, dynamical process that will wax and wane as the learning session progresses. Despite the best intentions of the technology designer to deliver an engaging experience, there will be individual differences in the extent to which a learning technology successfully engages a learner. Further, even if a learner is fully engaged at the onset of learning, engagement will eventually decrease over time as novelty fades and fatigue increases.
Reactive approaches are more sophisticated than proactive approaches. For one, the form of dynamic adaptivity exemplified above requires the ability to continually monitor engagement, to detect when engagement is waning, and to adapt instruction to address periods of disengagement. The technology could focus on a specific component of engagement, or could measure the construct more holistically. Engagement measurement can be achieved using the AAA-based approach discussed previously. A reactive learning technology must then alter its pedagogical/motivational strategies in response to the sensed engagement. It has multiple paths to pursue. It could do nothing if the learner is engaged and is on a positive learning trajectory. It could attempt to reorient attention if it detects that the learner is zoned out or distracted (D’Mello, 2016[158]). Hints and just-in-time explanations can be provided when confusion or frustration is detected (Forbes-Riley and Litman, 2011[86]). The system could provide choice, encourage breaks, or adjust the levels of challenge when it detects that a student is bored. It can also provide supportive messages which aim to motivate students to persevere in the learning (D’Mello and Graesser, 2012[159]; DeFalco et al., 2018[160]). If the technology is embodied in some form, such as with an animated pedagogical agent, it can utilise various social cues to boost engagement, for example by mirroring facial expressions and gestures (Burleson and Picard, 2007[161]) or looking unhappy when a disengaged behaviour is detected (Baker et al., 2006[162]). Examples of reactive learning technologies that implement some of these strategies are discussed below.
Responding to students’ inattention with Gaze Tutor
D’Mello et al. (2012[163]) respond to behavioural engagement during multimedia learning. The interface consists of an animated conversational agent that provides explanations on biology concepts with synthesised speech that is synchronised with annotated image (Figure 4.9). The system uses an eye tracker to track when a student is not attending to the important parts of the interface (i.e., the tutor or image). Gaze Tutor simply assumes that learners are disengaged when their gaze is not on the tutor or image for at least five consecutive seconds. When this occurs, it attempts to reengage learners with “witty” statements that directs them to reorient their attention towards the agent or the image (e.g. “I'm over here you know” and “Snap out of it. Let's keep going.”) Preliminary results suggest that these gaze-sensitive statements are successful in reorienting attention and improve learning outcomes.
Figure 4.9. GazeTutor interface with animated agent (0), image panel (1), and input box (2). Blank screen areas on the bottom are not displayed
Responding to students’ uncertainty with UNC-ITSPOKE
Forbes-Riley and Litman (2011[86]) was designed to examine whether automatic responses to learner uncertainty could improve learning outcomes while students engage with a spoken conversational intelligent tutoring system for physics. Uncertainty is related to confusion, an associated state that aligns with both the cognitive and affective components of engagement (D’Mello and Graesser, 2014[164]). UNC-ITSPOKE automatically detects learners’ certainty/uncertainty (from speech and language using an AAA-approach) in addition to the correctness/incorrectness of a response. It provides an explanation-based sub dialogue when the student is correct but uncertain about a response as this suggests a metacognitive failure. A validation study indicated that this form of adaptivity achieved slightly (but not significantly) higher learning outcomes compared to control conditions.
Responding to students’ mind-wandering with eye-mind reader
D’Mello et al. (2017[165]) and Mills et al. (2020[166]) respond to instances of mind-wandering (zone outs) during computerised reading (Figure 4.10). It uses an eye-gaze-based AAA-approach to detect mind-wandering (Faber, Bixler and D’Mello, 2018[167]) on a page-by-page basis (a page is a screen of text), and dynamically responds with comprehension assessments and re-reading opportunities. One initial intervention strategy consists of asking rote comprehension questions on the page where mind-wandering is detected and providing opportunities to re-read if the question is answered incorrectly. A validation study (D’Mello et al., 2017[165]) indicated that this intervention had the intended effect of reducing comprehension deficits attributable to mind-wandering in specific cases. However, since the interpolated questions were rote questions, this encouraged keyword spotting and a general shallow-level processing style. To address this, Mills et al. (2020[166]) replaced the rote multiple-choice questions with deeper-level questions that required learners to generate self-explanations in natural language. These were automatically scored and the system responded with feedback and provided opportunities to re-read and revise the explanations. Results suggest a positive effect for the intervention strategy compared to equivalent controls on retention (i.e. learning assessments completed a week later).
Figure 4.10. Eye tracking during reading. Filled circles display fixations (where eyes are focused) and lines display saccades (rapid eye movements between fixations)
Reactive technologies in the classroom
Most research on reactive learning technologies has mainly been done in in the lab, but this line of work is gradually moving to classrooms. Aslan et al. (2019[168]) developed Student Engagement Analytics Technology (SEAT) to assist teachers with the task of monitoring and responding to student real-time behavioural and emotional engagement. Here, the engagement measures provide assessments of student engagement to the teacher, who then can decide if and how to intervene. This research was done with students in Turkey who used a self-paced maths educational platform as part of their classes. An AAA-based engagement measure monitored facial features extracted from webcam video, patterns of interaction with the educational platform, and browser URL logs. It combined behavioural (on- or off-task) and emotional (bored, satisfied, confused) engagement assessments to yield an overall engagement score.
The engagement measure was then embedded in the SEAT interface which provided colour-coded (green, yellow, red) estimates of student engagement to teachers in real-time (Figure 4.11). Teachers used these data to intervene with individual students and their primary intervention strategies included verbal warnings, positive reinforcement, scaffolding (e.g. explaining the question, providing a hint), and close monitoring (watching the student’s screen to ensure they were on-task).
The researchers tested SEAT in a 16-week research study using both qualitative and quantitative methods. Interviews indicated both teachers and students had positive experiences using SEAT. Teachers were proactive about using the interface and reported that it made it easier to monitor the needs of individual students in a large class. Students also reported receiving individual attention when they needed it and felt that they were more engaged with the learning session. Finally, students in a SEAT-enabled class had higher (but not significant) learning gains compared to a parallel class of students without SEAT. However, this result should be considered preliminary since the study used a quasi-experimental design and only 37 students were tested across the two classes.
Figure 4.11. SEAT interface displaying the overall class-view (left) and student-specific view (right).
Conclusions and future directions
Research on engagement during learning has exploded in past years. Not only is the science of engagement in education advancing, but so are technology designs that make engagement a central design feature. Shared among the scientific research and technology design communities is a perspective that recognises engagement as an outcome worthy of consideration in its own right. There is a risk, however, that each proceeds independently, thereby missing obvious benefits of the two progressing in tandem.
To illustrate, the last two decades have produced a flurry of theoretical and empirical research aimed at defining engagement, identifying its causes and effects, and devising interventions to increase it (Christenson, Reschly and Wylie, 2012[18]). Unfortunately, the science of measuring engagement has not kept up; most researchers exclusively rely on self-report questionnaires as the sole measure of engagement (Fredricks and McColskey, 2012[42]), partly because self-reports are convenient and familiar, and perhaps because accessing more advanced technologies is more costly and intimidating. At the same time, in many countries, the computer is becoming an indispensable component in everyday learning (see (Vincent-Lancrin et al., 2019[169]). The increased bandwidth of available information in digital learning contexts also suggests that it may be unwise to exclusively rely on 20th-century methods to measure engagement in 21st-century digital learning contexts. Scientific research on engagement would benefit by considering digital learning experiences and incorporating associated methods into study designs.
On the flip side, the influx of technology in classrooms and the rise of online learning technologies produce huge volumes of data, which in principle, should lead to transformative advances in the measurement and facilitation of engagement. Unfortunately, this has not been the case yet. Despite the impressive volume, much of the data generated by these technologies is not very rich in depth, resulting in shallow measures of engagement that focus on very basic behavioural patterns, such as the number of logins, video plays, and so on. Further, despite many benefits, the proliferation of online learning has several side effects, most troublesome being a return to a transmission mode of education with its ineffectual passive learning strategies and lack of meaningful collaboration, which is one of the most engaging and productive ways to learn (Dillenbourg, 1999[170]; Stahl, Koschmann and Suthers, 2006[171]). Educational technology will benefit by incorporating principles from the scientific research on engagement into technology design.
Where will we be in the next 10 to 15 years? A pessimistic view is that research will proceed as usual, with scientific research on engagement chugging along in one direction and educational technology research proceeding in another, with rarely the two meeting. A more optimistic view is for the two to work hand in hand, resulting in learning technologies that meaningfully engage students and the emergence of more research on engagement with digital learning technologies. In this future, learning games – one of the best known experiences to foster deep engagement (as reviewed above) – also incorporate strategies such as scaffolds and learning supports that foster deep conceptual learning while also keeping learners engaged and motivated. And reactive-based approaches to improve engagement, which dynamically respond based on sensed engagement, will be deployed more readily as sensors become increasingly ubiquitous, wearable, and cost-effective; rigorous safeguards to protect privacy are established; efforts are made to ensure that the AI models underlying the technologies are unbiased and fair; and interdisciplinary collaborations between education and computer science researchers increase.
Importantly, these technologies should be developed with ethics, equity, and justice as core design considerations, not design after-thoughts, and technology designers seriously answer the question of what intelligent technologies should do rather than what they can do. There should be careful thought given to what data is collected and for how long it is stored, if at all, and end users (students, parents, teachers) should have the final say with respect to whether and when engagement is assessed. In this future, the ideas and research outlined in this paper will leave the confines of the research lab and small-scale studies in the real world to become a core component of digital learning that benefits millions of students worldwide. Here, opt-in measurement of engagement aimed for formative and non-evaluative purposes (while protecting privacy of students) coupled with intelligent strategies to enhance engagement will be essential elements of digital learning technologies. A future of efficient, effective, and engaging digital learning awaits us all.
References
[104] Algebra Nation (n.d.), Algebra Nation, https://loveforquotes.com/t/algebra-nation (accessed on 29 April 2021).
[105] Anderson, J. (1982), “Acquisition of cognitive skill”, Psychological Review, Vol. 89/4, pp. 369-406.
[63] Arnold, K. and M. Pistilli (2012), Course signals at Purdue: Using learning analytics to increase student success.
[168] Aslan, S. et al. (2019), “Investigating the Impact of a Real-time, Multimodal Student Engagement Analytics Technology in Authentic Classrooms”, in Proceedings of the ACM CHI Conference on Human Factors in Computing Systems (CHI 2019)., ACM, New York.
[66] Atkins-Burnett, S. et al. (2012), Landscape analysis of non-cognitive measures.
[59] Aung, A., A. Ramakrishnan and J. Whitehill (2018), Who Are They Looking At? Automatic Eye Gaze Following for Classroom Observation Video Analysis, Paper presented at the International Conference on Educational Data Mining (EDM).
[162] Baker, R. et al. (2006), Adapting to when students game an intelligent tutoring system, Paper presented at the Intelligent Tutoring Systems Conference.
[7] Baker, R. et al. (2010), “Better to be frustrated than bored: The incidence, persistence, and impact of learners’ cognitive–affective states during interactions with three different computer-based learning environments”, International Journal of Human-Computer Studies, Vol. 68/4, pp. 223-241.
[70] Baker, R. and J. Ocumpaugh (2015), “Interaction-based affect detection in educational software”, in Calvo, R. et al. (eds.), The Oxford Handbook of Affective Computing, Oxford University Press, New York.
[153] Banawan, M., M. Rodrigo and J. Andres (2015), An investigation of frustration among students using physics playground. In Doctoral Student Consortium (DSC), Proceedings of the 23rd International Conference on Computers in Education.
[148] Barab, S. et al. (2005), “Making learning fun: Quest Atlantis, a game without guns”, Educational technology research and development, Vol. 53/1, pp. 86-107.
[60] Bidwell, J. and H. Fuchs (2011), “Classroom analytics: Measuring student engagement with automated gaze tracking”, Behav Res Methods, Vol. 49, p. 113.
[90] Bixler, R. and S. D’Mello (2016), “Automatic gaze-based user-independent detection of mind wandering during computerized reading”, User Modeling & User-Adapted Interaction, Vol. 26, pp. 33-68.
[81] Bixler, R. and S. D’Mello (2013), “Detecting engagement and boredom during writing with keystroke analysis, task appraisals, and stable traits”, in Proceedings of the 2013 International Conference on Intelligent User Interfaces (IUI 2013), ACM, New York, NY.
[95] Blanchard, N. et al. (2014), “Automated physiological-based detection of mind wandering during learning”, in Trausan-Matu, S. et al. (eds.), Proceedings of the 12th International Conference on Intelligent Tutoring Systems (ITS 2014), Springer International Publishing, Switzerland.
[145] Blumenfeld, P. et al. (1991), “Motivating project-based learning: Sustaining the doing, supporting the learning”, Educational Psychologist, Vol. 26/3-4, pp. 369-398.
[98] Bosch, N. et al. (2015), “Accuracy vs. Availability Heuristic in Multimodal Affect Detection in the Wild”, in Proceedings of the 17th ACM International Conference on Multimodal Interaction (ICMI 2015), ACM, New York, NY.
[85] Bosch, N. et al. (2016), “Using video to automatically detect learner affect in computer-enabled classrooms”, ACM Transactions on Interactive Intelligent Systems, Vol. 6/2, pp. 17.1-17.31.
[75] Bosch, N. and S. D’Mello (2019), “Automatic Detection of Mind Wandering from Video in the Lab and in the Classroom”, IEEE Transactions on Affective Computing, https://doi.org/10.1109/TAFFC.2019.2908837.
[23] Bransford, J., A. Brown and R. Cocking (2000), How people learn, National Academy Press, Washington, DC.
[121] Brom, C., T. Stárková and S. D’Mello (2018), “How effective is emotional design? A meta-analysis on facial anthropomorphisms and pleasant colors during multimedia learning”, Educational Research Review, Vol. 25, pp. 100-119.
[106] Brown, J. and K. VanLehn (1980), “Repair theory: A generative theory of bugs in procedural skills”, Cognitive Science, Vol. 4/379-426,, https://doi.org/10.1016/S0364-0213(80)80010-3.
[161] Burleson, W. and R. Picard (2007), “Evidence for gender specific approaches to the development of emotionally intelligent learning companions”, IEEE Intelligent Systems, Vol. 22/4, pp. 62-69.
[20] Charsky, D. (2010), “From edutainment to serious games: A change in the use of game characteristics”, Games and culture, Vol. 5/2, pp. 177-198.
[123] Chi, M. and R. Wylie (2014), “The ICAP framework: Linking cognitive engagement to active learning outcomes”, Educational Psychologist, Vol. 49/4, pp. 219-243.
[18] Christenson, S., A. Reschly and C. Wylie (2012), Handbook of research on student engagement, Springer, New York.
[140] Coller, B. and M. Scott (2009), “Effectiveness of using a video game to teach a course in mechanical engineering”, Computers & Education, Vol. 53/3, pp. 900-912.
[91] Conati, C., V. Aleven and A. Mitrovic (2013), “Eye-Tracking for Student Modelling in Intelligent Tutoring Systems”, in Sottilare, R. et al. (eds.), Design Recommendations for Intelligent Tutoring Systems - Volume 1: Learner Modeling, Army Research Laboratory, Orlando, FL.
[28] Craig, S. et al. (2008), “Emote aloud during learning with AutoTutor: Applying the Facial Action Coding System to cognitive–affective states during learning”, Cognition & Emotion, Vol. 22/5, pp. 777-788, https://doi.org/10.1080/02699930701516759.
[110] Craig, S. et al. (2004), “Affect and learning: An exploratory look into the role of affect in learning”, Journal of Educational Media, Vol. 29, pp. 241-250, https://doi.org/10.1080/1358165042000283101.
[40] Csikszentmihalyi, M. (1990), Flow: The psychology of optimal experience, Harper and Row, New York.
[8] Csikszentmihalyi, M. (1975), Beyond boredom and anxiety, Jossey-Bass, San Francisco, CA.
[49] Csikszentmihalyi, M. and R. Larson (1987), “Validity and reliability of the experience-sampling method”, The Journal of nervous and mental disease, Vol. 175/9, pp. 526-536.
[1] Danckert, J. and C. Merrifield (2018), “Boredom, sustained attention and the default mode network”, Experimental Brain Research, Vol. 236/9, pp. 2507-2518.
[9] Daniels, L. et al. (2009), “A longitudinal analysis of achievement goals: From affective antecedents to emotional effects and achievement outcomes”, Journal of Educational Psychology, Vol. 101/4, pp. 948-963, https://doi.org/10.1037/a0016096.
[80] De Falco, J., R. Baker and S. D’Mello (2014), “Addressing behavioral disengagement in online learning”, in Sottilare, R. et al. (eds.), Design Recommendations for Adaptive Intelligent Tutoring Systems: Adaptive Instructional Strategies (Volume 2), Army Research Laboratory, Orlando, FL.
[147] Dede, C. (2009), “Immersive interfaces for engagement and learning”, Science, Vol. 323/5910, pp. 66-69.
[160] DeFalco, J. et al. (2018), “Detecting and addressing frustration in a serious game for military training”, International Journal of Artificial Intelligence in Education, Vol. 28/2, pp. 152-193.
[116] del Soldato, T. and B. du Boulay (1995), “Implementation of motivational tactics in tutoring systems”, International Journal of Artificial Intelligence in Education, Vol. 6, pp. 337-378.
[141] DeRouin-Jessen, R. (2008), Game on: The Impact of Game Features in Computer-Based Training.
[170] Dillenbourg, P. (1999), “What do you mean by collaborative learning”, in Dillenbourg, P. (ed.), Collaborative-learning: Cognitive and computational approaches.
[96] Dindar, M. et al. (2017), “Matching self-reports with electrodermal activity data: Investigating temporal changes in self-regulated learning”, Education and Information Technologies, pp. 1-18.
[113] D’Mello, S. (2019), “What do we think about when we learn?”, in Millis, K. et al. (eds.), Deep Comprehension: Multi-Disciplinary Approaches to Understanding, Enhancing, and Measuring Comprehension, Routledge, New York, NY.
[158] D’Mello, S. (2016), “Giving Eyesight to the Blind: Towards attention-aware AIED”, International Journal of Artificial Intelligence in Education, Vol. 26/2, pp. 645-659.
[111] D’Mello, S. (2013), “A selective meta-analysis on the relative incidence of discrete affective states during learning with technology”, Journal of Educational Psychology, Vol. 105/4, pp. 1082-1099.
[68] D’Mello, S., E. Dieterle and A. Duckworth (2017), “Advanced, Analytic, Automated (AAA) Measurement of Engagement during Learning”, Educational Psychologist, Vol. 52/2, pp. 104-123.
[157] D’Mello, S. and A. Graesser (2015), “Feeling, thinking, and computing with affect-aware learning technologies”, in Calvo, R. et al. (eds.), The Oxford Handbook of Affective Computing, Oxford University Press, New York.
[164] D’Mello, S. and A. Graesser (2014), “Confusion”, in Pekrun, R. and L. Linnenbrink-Garcia (eds.), International handbook of emotions in education, Routledge, New York, NY.
[159] D’Mello, S. and A. Graesser (2012), “AutoTutor and Affective AutoTutor: Learning by talking with cognitively and emotionally intelligent computers that talk back”, ACM Transactions on Interactive Intelligent Systems, Vol. 2/4, pp. 23:2-23:39.
[152] D’Mello, S. and A. Graesser (2012), “Dynamics of affective states during complex learning”, Learning and Instruction, Vol. 22/2, pp. 145-157, https://doi.org/10.1016/j.learninstruc.2011.10.001.
[99] D’Mello, S. and A. Graesser (2010), “Multimodal semi-automated affect detection from conversational cues, gross body language, and facial features”, User Modeling and User-adapted Interaction, Vol. 20/2, pp. 147-187.
[93] D’Mello, S. and A. Graesser (2009), “Automatic detection of learners’ affect from gross body language”, Applied Artificial Intelligence, Vol. 23/2, pp. 123 - 150, http://www.informaworld.com/10.1080/08839510802631745.
[79] D’Mello, S. et al. (2016), “Attending to attention: Detecting and combating mind wandering during computerized reading”, in Extended Abstracts of the ACM SIGCHI Conference on Human Factors in Computing Systems (CHI 2016), ACM, New York.
[165] D’Mello, S. et al. (2017), “Zone out no more: Mitigating mind wandering during computerized reading”, in Hu, X. et al. (eds.), Proceedings of the 10th International Conference on Educational Data Mining, International Educational Data Mining Society.
[163] D’Mello, S. et al. (2012), “Gaze tutor: A gaze-reactive intelligent tutoring system”, International Journal of Human-Computer Studies, Vol. 70/5, pp. 377-398.
[58] D’Mello, S. et al. (2008), “Self versus teacher judgments of learner emotions during a tutoring session with AutoTutor”, in Woolf, B. et al. (eds.), Proceedings of the 9th international conference on Intelligent Tutoring Systems, Springer-Verlag, Berlin, Heidelberg, http://dx.doi.org/10.1007/978-3-540-69132-7_6.
[31] Duckworth, A. et al. (2019), “Self-control and academic achievement”, Annual Review of Psychology, Vol. 70, pp. 373-399.
[45] Duckworth, A. and D. Yeager (2015), “Measurement matters: Assessing personal qualities other than cognitive ability for educational purposes.”, Educational Researcher, Vol. 44/4, pp. 237-251.
[2] Eastwood, J. et al. (2012), “The unengaged mind: Defining boredom in terms of attention”, Perspectives on Psychological Science, Vol. 7/5, pp. 482-495.
[32] Eccles, J. and M. Wang (2012), “Part I commentary: So what is student engagement anyway?”, in Christenson, S., A. Reschly and C. Wylie (eds.), Handbook of research on student engagement, Springer, New York.
[30] Ericsson, K., R. Krampe and C. Tesch-Römer (1993), “The role of deliberate practice in the acquisition of expert performance”, Psychological Review, Vol. 100/3, p. 363.
[167] Faber, M., R. Bixler and S. D’Mello (2018), “An automated behavioral measure of mind wandering during computerized reading”, Behavior Research Methods, Vol. 50/1, pp. 134-150.
[10] Farrell, E. et al. (1988), “Giving voice to high-school students: Pressure and boredom, ya know what I’m sayin’?”, American Educational Research Journal, Vol. 25/4, pp. 489-502.
[139] Ferguson, C. and A. Garza (2011), “Call of (civic) duty: Action games and civic behavior in a large sample of youth”, Computers in Human behavior, Vol. 27/2, pp. 770-775.
[11] Finn, J. and K. Voelkl (1993), “School characteristics related to student engagement”, The Journal of Negro Education, Vol. 62/3, pp. 249-268.
[86] Forbes-Riley, K. and D. Litman (2011), “Benefits and challenges of real-time uncertainty detection and adaptation in a spoken dialogue computer tutor”, Speech Communication, Vol. 53/9-10, pp. 1115-1136, https://doi.org/10.1016/j.specom.2011.02.006.
[34] Fredricks, J., P. Blumenfeld and A. Paris (2004), “School engagement: Potential of the concept, state of the evidence”, Review of Educational Research, Vol. 74/1, pp. 59-109.
[42] Fredricks, J. and W. McColskey (2012), “The measurement of student engagement: A comparative analysis of various methods and student self-report instruments”, in Christenson, S., A. Reschly and C. Wylie (eds.), Handbook of research on student engagement, Springer, New York.
[129] Fullerton, T., C. Swain and S. Hoffman (2008), Game design workshop: a playcentric approach to creating innovative games, Morgan Kaufmann, Burlington, MA.
[76] Gardner, J., C. Brooks and R. Baker (2019), Evaluating the fairness of predictive student models through slicing analysis., Paper presented at the Proceedings of the 9th International Conference on Learning Analytics & Knowledge.
[41] Gee, J. (2003), What video games have to teach us about learning and literacy, Palgrave Macmillan, New York.
[117] Gibson, D. et al. (2015), “Digital badges in education”, Education and Information Technologies, Vol. 20/2, pp. 403-410.
[82] Gobert, J., R. Baker and M. Wixon (2015), “Operationalizing and detecting disengagement within online science microworlds”, Educational Psychologist, Vol. 50/1, pp. 43-57.
[100] Grafsgaard, J. et al. (2014), “Predicting learning and affect from multimodal data streams in task-oriented tutorial dialogue”, in Stamper, J. et al. (eds.), Proceedings of the 7th International Conference on Educational Data Mining, International Educational Data Mining Society.
[132] Green, C. and D. Bavelier (2012), “Learning, attentional control, and action video games”, Current Biology, Vol. 22/6, pp. R197-R206.
[133] Green, C. and D. Bavelier (2007), “Action-video-game experience alters the spatial resolution of vision”, Psychological Science, Vol. 18/1, pp. 88-94.
[43] Greene, B. (2015), “Measuring cognitive engagement with self-report scales: Reflections from over 20 years of research”, Educational Psychologist, Vol. 50/1, pp. 1-17.
[12] Griffiths, A. et al. (2012), “The relations of adolescent student engagement with troubling and high-risk behaviors”, in Christenson, S., A. Reschly and C. Wylie (eds.), Handbook of research on student engagement, Springer, New York.
[112] Hawkins, W., N. Heffernan and R. Baker (2013), “Which is more responsible for boredom in intelligent tutoring systems: students (trait) or problems (state)?”, in Nijholt, A., S. D’Mello and M. Pantic (eds.), Proceedings of the 5th International Conference on Affective Computing and Intelligent Interaction (ACII 2013), IEEE, Washington, DC.
[47] Heine, S. et al. (2002), “What’s wrong with cross-cultural comparisons of subjective Likert scales?: The reference-group effect”, Journal of Personality and Social Psychology, Vol. 82/6, pp. 903-918.
[44] Henrie, C., L. Halverson and C. Graham (2015), “Measuring student engagement in technology-mediated learning: A review”, Computers & Education, Vol. 90, pp. 36-53.
[38] Hidi, S. and K. Renninger (2006), “The four-phase model of interest development”, Educational Psychologist, Vol. 41/2, pp. 111-127, https://doi.org/10.1207/s15326985ep4102_4.
[3] Hunter, A. and J. Eastwood (2018), “Does state boredom cause failures of attention? Examining the relations between trait boredom, state boredom, and sustained attention”, Experimental Brain Research, Vol. 236/9, pp. 2483-2492.
[83] Hutt, S., J. Grafsgaard and S. D’Mello (2019), “Time to Scale: Generalizable Affect Detection for Tens of Thousands of Students across An Entire Schoolyear”, in Proceedings of the ACM CHI Conference on Human Factors in Computing Systems (CHI 2019), ACM, New York.
[92] Hutt, S. et al. (2019), “Automated Gaze-Based Mind Wandering Detection during Computerized Learning in Classrooms”, User Modeling & User-Adapted Interaction.
[156] Ibáñez, M. et al. (2014), “Experimenting with electromagnetism using augmented reality: Impact on flow student experience and educational effectiveness”, Computers & Education, Vol. 71, pp. 1-13.
[138] Jackson, L. et al. (2012), “Information technology use and creativity: Findings from the Children and Technology Project”, Computers in Human behavior, Vol. 28/2, pp. 370-376.
[77] Jensen, E., S. Hutt and S. D’Mello (2019), Generalizability of Sensor-Free Affect Detection Models in a Longitudinal Dataset of Tens of Thousands of Students, Proceedings of the 2019 Educational Data Mining Conference (EDM 2019). International Educational Data Mining Society.
[127] Johnson, C. and R. Mayer (2010), “Applying the self-explanation principle to multimedia learning in a computer-based game-like environment”, Computers in Human behavior, Vol. 26/6, pp. 1246-1252.
[50] Kahneman, D. et al. (2004), “A survey method for characterizing daily life experience: The day reconstruction method”, Science, Vol. 306/5702, pp. 1776-1780.
[101] Kapoor, A. and R. Picard (2005), “Multimodal affect recognition in learning environments”, in Proceedings of the 13th annual ACM international conference on Multimedia, ACM, New York, http://doi.acm.org/10.1145/1101149.1101300.
[118] Kapp, K. (2012), The gamification of learning and instruction, Wiley San Francisco.
[24] Karpicke, J. and J. Blunt (2011), “Retrieval practice produces more learning than elaborative studying with concept mapping”, Science, Vol. 331/6018, pp. 772-775.
[61] Klein, R. and T. Celik (2017), The Wits Intelligent Teaching System: Detecting student engagement during lectures using convolutional neural networks, IEEE.
[46] Krosnick, J. (1999), “Survey research”, Annual Review of Psychology, Vol. 50/1, pp. 537-567.
[57] Lehman, B. et al. (2008), “What Are You Feeling? Investigating Student Affective States During Expert Human Tutoring Sessions”, in Intelligent Tutoring Systems, Lecture Notes in Computer Science, Springer Berlin Heidelberg, Berlin, Heidelberg, https://doi.org/10.1007/978-3-540-69132-7_10.
[64] Lehr, C., M. Sinclair and S. Christenson (2004), “Addressing student engagement and truancy prevention during the elementary school years: A replication study of the check & connect model”, Journal of education for students placed at risk, Vol. 9/3, pp. 279-301.
[124] Lepper, M. and J. Henderlong (2000), “Turning“ play” into“ work” and“ work” into“ play”: 25 years of research on intrinsic versus extrinsic motivation”, in Sansone, C. and J. Harackiewicz (eds.), Intrinsic and extrinsic motivation: The search for optimal motivation and performance, Academic Press, San Diego, CA.
[130] Malone, T. and M. Lepper (1987), “Making learning fun: A taxonomy of intrinsic motivations for learning”, in Snow, R. and M. Farr (eds.), Aptitude, learning, and instruction III: Cognitive and affective process analyses, Erlbaum, Hilsdale, NJ.
[13] Mann, S. and A. Robinson (2009), “Boredom in the lecture theatre: an investigation into the contributors, moderators and outcomes of boredom amongst university students”, British Educational Research Journal, Vol. 35/2, pp. 243-258, https://doi.org/10.1080/01411920802042911.
[154] Martín-Gutiérrez, J. et al. (2017), “Virtual technologies trends in education”, EURASIA Journal of Mathematics Science and Technology Education, Vol. 13/2, pp. 469-486.
[74] Martin, J. (2010), Lower Merion district’s laptop saga ends with $610,000 settlement, http://articles.philly.com/2010-10-12/news/24981536_1_laptop-students-district-several-million-dollars.
[108] Ma, W. et al. (2014), “Intelligent tutoring systems and learning outcomes: A meta-analysis”, Journal of Educational Psychology, Vol. 106/4, pp. 901-918.
[27] McNamara, D. et al. (2006), “Improving adolescent students’ reading comprehension with iSTART”, Journal of Educational Computing Research, Vol. 34/147-171.
[56] Mehl, M. et al. (2001), “The Electronically Activated Recorder (EAR): A device for sampling naturalistic daily activities and conversations”, Behavior Research Methods, Instruments, & Computers, Vol. 33/4, pp. 517-523.
[78] Miller, W. et al. (2014), Boredom Across Activities, and Across the Year, within Reasoning Mind, Paper presented at the Workshop on Data Mining for Educational Assessment and Feedback (ASSESS 2014), New York, NY.
[97] Mills, C. et al. (2017), “Put Your Thinking Cap On: Detecting Cognitive Load using EEG during Learning”, in Molenaar, I., O. X. and S. Dawson (eds.), Proceedings of the 7th International Learning Analytics and Knowledge Conference (LAK’17), ACM, New York, NY.
[166] Mills, C. et al. (2020), “Eye-Mind Reader: An Intelligent Reading Interface that Promotes Long-term Comprehension by Detecting and Responding to Mind Wandering”, Human-Computer Interaction, https://doi.org/10.1080/07370024.2020.1716762.
[87] Monkaresi, H. et al. (2017), “Automated detection of engagement using video-based estimation of facial expressions and heart rate”, IEEE Transactions on Affective Computing, Vol. 8/1, pp. 15-28.
[94] Mota, S. and R. Picard (2003), Automated posture analysis for detecting learner’s interest level.
[52] Nystrand, M. and A. Gamoran (1991), “Instructional discourse, student engagement, and literature achievement”, Research in the Teaching of English, Vol. 25/3, pp. 261-290.
[73] Ocumpaugh, J., R. Baker and M. Rodrigo (2015), Baker Rodrigo Ocumpaugh Monitoring Protocol (BROMP) 2.0 Technical and Training Manual.
[102] Olney, A. et al. (2012), “Guru: A computer tutor that models expert human tutors”, in Cerri, S. et al. (eds.), Proceedings of the 11th International Conference on Intelligent Tutoring Systems, Springer-Verlag, Berlin/Heidelberg.
[21] Papert, S. (1998), “Does easy do it? Children, games, and learning”, Game Developer, Vol. 5/6, p. 88.
[84] Pardos, Z. et al. (2013), “Affective states and state tests: Investigating how affect throughout the school year predicts end of year learning outcomes”, in Suthers, D. et al. (eds.), Proceedings of the 3rd International Conference on Learning Analytics and Knowledge, ACM, New York, NY.
[14] Patrick, B., E. Skinner and J. Connell (1993), “What motivates children’s behavior and emotion? Joint effects of perceived control and autonomy in the academic domain.”, Journal of Personality and Social Psychology, Vol. 65/4, pp. 781-791, https://doi.org/10.1037/0022-3514.65.4.781.
[15] Pekrun, R. et al. (2010), “Boredom in achievement settings: Exploring control–value antecedents and performance outcomes of a neglected emotion”, Journal of Educational Psychology, Vol. 102/3, pp. 531-549, https://doi.org/10.1037/a0019243.
[4] Pekrun, R. et al. (2014), “Boredom and academic achievement: Testing a model of reciprocal causation”, Journal of Educational Psychology, Vol. 106/3, pp. 696-710.
[37] Pekrun, R. and L. Linnenbrink-Garcia (2012), “Academic emotions and student engagement”, in Christenson, S., A. Reschly and C. Wylie (eds.), Handbook of research on student engagement, Springer, New York.
[16] Perkins, R. and A. Hill (1985), “Cognitive and affective aspects of boredom”, British Journal of Psychology, Vol. 76/May, pp. 221-234.
[88] Pham, P. and J. Wang (2015), “AttentiveLearner: improving mobile MOOC learning via implicit heart rate tracking”, in International Conference on Artificial Intelligence in Education, Springer, Berlin Heidelberg.
[53] Pianta, R., B. Hamre and J. Allen (2012), “Teacher-student relationships and engagement: Conceptualizing, measuring, and improving the capacity of classroom interactions”, in Christenson, S., A. Reschly and C. Wylie (eds.), Handbook of research on student engagement, Springer, New York, NY.
[119] Plass, J. et al. (2014), “Emotional design in multimedia learning: Effects of shape and color on affect and learning”, Learning and Instruction, Vol. 29, pp. 128-140.
[125] Plass, J., B. Homer and C. Kinzer (2015), “Foundations of game-based learning”, Educational Psychologist, Vol. 50/4, pp. 258-283.
[48] Podsakoff, P. et al. (2003), “Common method biases in behavioral research: A critical review of the literature and recommended remedies”, Journal of Applied Psychology, Vol. 88/5, pp. 879-903.
[71] Porayska-Pomsta, K. et al. (2013), “Knowledge elicitation methods for affect modelling in education”, International Journal of Artificial Intelligence in Education, Vol. 22, pp. 107-140.
[155] Psotka, J. (1995), “Immersive training systems: Virtual reality and education and training”, Instructional science, Vol. 23/5-6, pp. 405-431.
[5] Putwain, D. et al. (2018), “Reciprocal relations between students’ academic enjoyment, boredom, and achievement over time”, Learning and Instruction, Vol. 54, pp. 73-81.
[62] Raca, M., L. Kidzinski and P. Dillenbourg (2015), “Translating head motion into attention-towards processing of student’s body language”, in Proceedings of the 8th International Conference on Educational Data Mining, International Educational Data Mining Society.
[114] Randall, J., F. Oswald and M. Beier (2014), “Mind-wandering, cognition, and performance: A theory-driven meta-analysis of attention regulation”, Psychological Bulletin, Vol. 140/6, pp. 1411-1431.
[36] Reeve, J. and C. Tseng (2011), “Agency as a fourth aspect of students’ engagement during learning activities”, Contemporary Educational Psychology, Vol. 36/4, pp. 257-267.
[35] Renninger, K. and J. Bachrach (2015), “Studying triggers for interest and engagement using observational methods”, Educational Psychologist, Vol. 50/1, pp. 58-69.
[33] Reschly, A. and S. Christenson (2012), “Jingle, jangle, and conceptual haziness: Evolution and future directions of the engagement construct”, in S. Christenson, A. Reschly and C. Wylie (eds.), Handbook of research on student engagement, Springer, Berlin.
[22] Rey, G. (2012), “A review of research and a meta-analysis of the seductive detail effect”, Educational Research Review, Vol. 7/3, pp. 216-237.
[115] Risko, E. et al. (2013), “Everyday attention: mind wandering and computer use during lectures”, Computers & Education, Vol. 68/1, pp. 275-283.
[126] Ritterfeld, U., M. Cody and P. Vorderer (2009), Serious games: Mechanisms and effects, Routledge, New York and London.
[29] Rodrigo, M. and R. Baker (2011), “Comparing Learners’ Affect While Using an Intelligent Tutor and an Educational Game”, Research and Practice in Technology Enhanced Learning, Vol. 6, pp. 43-66.
[25] Roediger, H. and J. Karpicke (2006), “Test-enhanced learning - Taking memory tests improves long-term retention”, Psychological Science, Vol. 17/3, pp. 249-255.
[149] Rowe, J. et al. (2009), Crystal island: A narrative-centered learning environment for eighth grade microbiology, Paper presented at the Workshop on Intelligent Educational Games at the 14th International Conference on Artificial Intelligence in Education, Brighton, UK.
[151] Rowe, J. et al. (2010), “Integrating learning and engagement in narrative-centered learning environments”, in Kay, J. and V. Aleven (eds.), Proceedings of the 10th International Conference on Intelligent Tutoring Systems, Springer, Berlin / Heidelberg.
[54] Ryu, S. and D. Lombardi (2015), “Coding classroom interactions for collective and individual engagement”, Educational Psychologist, Vol. 50/1, pp. 70-83.
[72] Sabourin, J. and J. Lester (2014), “Affect and Engagement in Game-Based Learning Environments”, IEEE Transactions on Affective Computing, Vol. 5/1, pp. 45-56.
[122] Sanchez, E., S. Young and C. Jouneau-Sion (2016), “Classcraft: from gamification to ludicization of classroom management”, Education and Information Technologies, Vol. 22/2, pp. 497-513, https://doi.org/10.1007/s10639-016-9489-6.
[146] Schneider, B. et al. (2020), Learning Science-the Value of Crafting Engagement in Science Environments, New Haven. CT: Yale University Press.
[67] Shechtman, N. et al. (2013), Promoting grit, tenacity, and perseverance: Critical factors for success in the 21st century.
[131] Shute, V., L. Rieber and R. Van Eck (2011), “Games... and... learning”, in Reiser, R. and J. Dempsey (eds.), Trends and issues in instructional design and technology, Pearson Education, Inc.
[134] Shute, V., M. Ventura and F. Ke (2015), “The power of play: The effects of Portal 2 and Lumosity on cognitive and noncognitive skills”, Computers & Education, Vol. 80, pp. 58-67.
[103] Shute, V., M. Ventura and Y. Kim (2013), “Assessment and learning of qualitative physics in Newton’s playground”, The Journal of Educational Research, Vol. 106/6, pp. 423-430.
[128] Shute, V. et al. (2014), “Video games and learning”, in Tierney, W. et al. (eds.), Postsecondary play: The role of games and social media in higher education, John Hopkins Press, Baltimore, MD.
[39] Sinatra, G., B. Heddy and D. Lombardi (2015), “The challenges of defining and measuring student engagement in science”, Educational Psychologist, Vol. 50/1, pp. 1-13.
[65] Skinner, E. and M. Belmont (1993), “Motivation in the classroom: Reciprocal effects of teacher behavior and student engagement across the school year”, Journal of Educational Psychology, Vol. 85/4, pp. 571-581.
[135] Skoric, M., L. Teo and R. Neo (2009), “Children and video games: addiction, engagement, and scholastic achievement”, Cyberpsychology & behavior, Vol. 12/5, pp. 567-572.
[107] Sleeman, D. and J. Brown (1982), Intelligent tutoring systems, Academic Press, New York.
[150] Spires, H. et al. (2011), “Problem solving and game-based learning: Effects of middle grade students’ hypothesis testing strategies on learning outcomes”, Journal of Educational Computing Research, Vol. 44/4, pp. 453-472.
[171] Stahl, G., T. Koschmann and D. Suthers (2006), “Computer-supported collaborative learning: An historical perspective”, in Sawyer, K. (ed.), Cambridge handbook of the learning sciences, Cambridge University Press, New York, NY.
[26] Steenbergen-Hu, S. and H. Cooper (2014), “A meta-analysis of the effectiveness of intelligent tutoring systems on college students’ academic learning”, Journal of Educational Psychology, Vol. 106/2, pp. 331-347.
[109] Steenbergen-Hu, S. and H. Cooper (2013), “A meta-analysis of the effectiveness of intelligent tutoring systems on K–12 students’ mathematical learning”, Journal of Educational Psychology, Vol. 105/4, pp. 970-987.
[142] Tobias, S. and J. Fletcher (2011), Computer games and instruction, Information Age Publishers, Charlotte, NC.
[51] Turner, J. and D. Meyer (2000), “Studying and understanding the instructional contexts of classrooms: Using our past to forge our future”, Educational Psychologist, Vol. 35/2, pp. 69-85.
[6] Tze, V., L. Daniels and R. Klassen (2016), “Evaluating the relationship between boredom and academic outcomes: a meta-analysis”, Educational Psychology Review, Vol. 28/1, pp. 119-144.
[120] Um, E. et al. (2012), “Emotional design in multimedia learning”, Journal of Educational Psychology, Vol. 104/2, pp. 485-498.
[69] Valstar, M. et al. (2012), “Meta-analysis of the first facial expression recognition challenge”, IEEE Transactions on Systems, Man, and Cybernetics, Part B: Cybernetics, Vol. 42/4, pp. 966-979.
[136] Ventura, M., V. Shute and Y. Kim (2012), “Video gameplay, personality and academic performance”, Computers & Education, Vol. 58/4, pp. 1260-1266.
[137] Ventura, M., V. Shute and W. Zhao (2013), “The relationship between video game use and a performance-based measure of persistence”, Computers & Education, Vol. 60/1, pp. 52-58, https://doi.org/10.1016/j.compedu.2012.07.003.
[169] Vincent-Lancrin, S. et al. (2019), Measuring Innovation in Education 2019: What Has Changed in the Classroom?, Educational Research and Innovation, OECD Publishing, Paris, https://dx.doi.org/10.1787/9789264311671-en.
[55] Volpe, R. et al. (2005), “Observing students in classroom settings: A review of seven coding schemes”, School Psychology Review, Vol. 34/4, pp. 454-474.
[17] Wasson, A. (1981), “Susceptibility to Boredom and Deviant-Behavior at School”, Psychological Reports, Vol. 48/3, pp. 901-902.
[89] Whitehill, J. et al. (2014), “The faces of engagement: Automatic recognition of student engagement from facial expressions”, IEEE Transactions on Affective Computing, Vol. 5/1, pp. 86 - 98.
[143] Wilson, K. et al. (2009), “Relationships between game attributes and learning outcomes review and research proposals”, Simulation & gaming, Vol. 40/2, pp. 217-266.
[19] Yang, D. et al. (2013), Turn on, tune in, drop out: Anticipating student dropouts in massive open online courses, Paper presented at the Proceedings of the 2013 NIPS Data-Driven Education Workshop.
[144] Young, M. et al. (2012), “Our Princess Is in Another Castle: A Review of Trends in Serious Gaming for Education”, Review of Educational Research, Vol. 82/1, pp. 61-89.