The digital revolution continues apace. Text-mining techniques are enabling fast-accelerating digital technologies to be identified. Measuring the development of artificial intelligence (AI) is challenging as the boundaries between AI and other innovations are blurred and change over time, but experimental work leveraging the expert community is leading to definitions of AI-related science and technologies. Patent databases are being used to identify the main fields in which AI is being applied, and web footprints are being leveraged to identify companies developing and using AI, as well as to examine their applications throughout the economy. In addition, bibliometric analysis is being used to reveal who precisely is leading in AI-related science. Thanks to sustained technological progress, growth in network capacity and decreasing costs of ICT products, more content can be accommodated on Internet infrastructure. Moreover, as bandwidth for data transmission increases, flows of information generated by digital technologies and digitally enabled activities are growing at an unprecedented pace and data centres are becoming a critical infrastructure underpinning the digital transformation.
Measuring the Digital Transformation
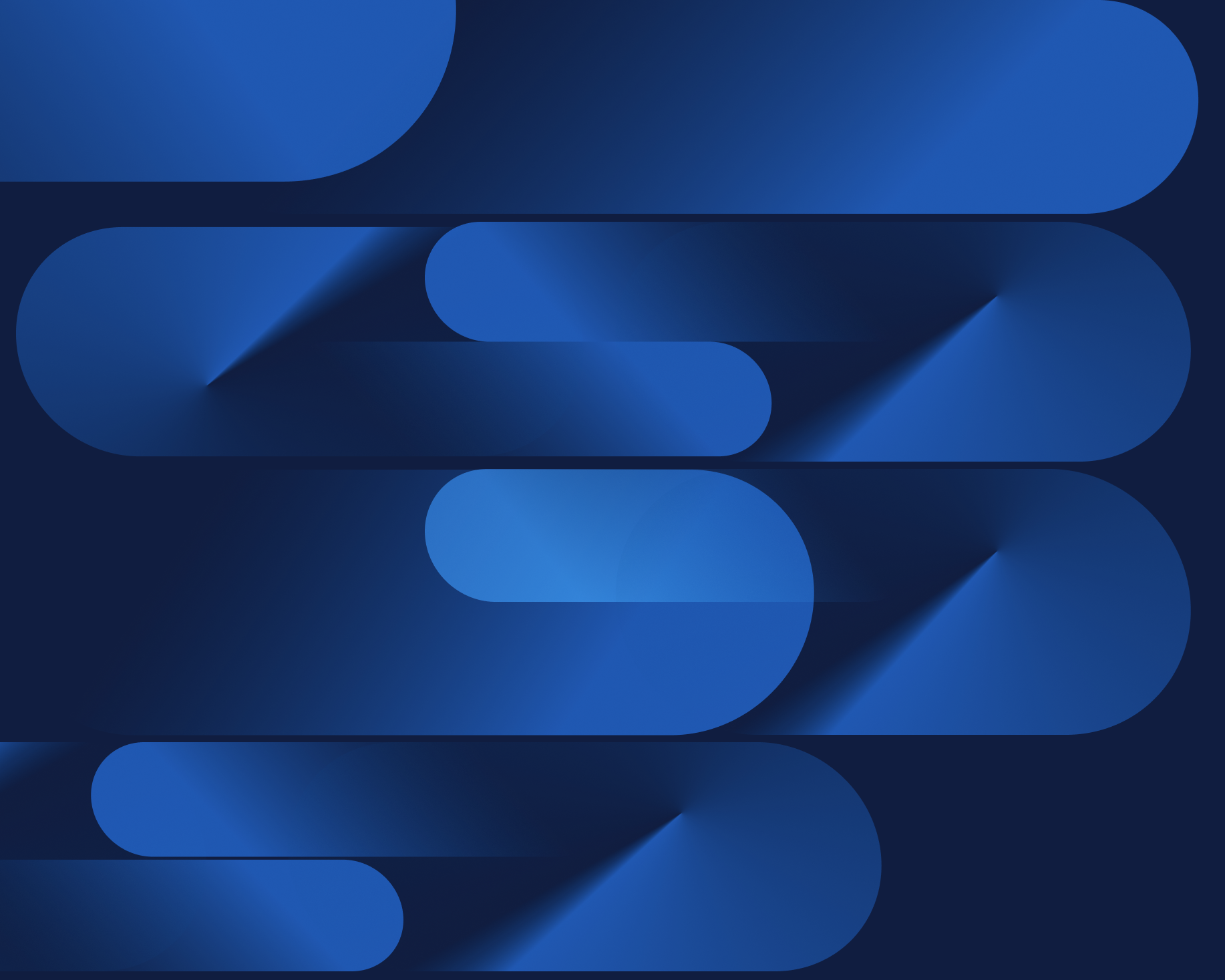
1.1. Technology trends
Abstract
At the digital frontier
Digital technology waves
AI technologies
AI-related applications
Mapping AI companies
AI companies in key sectors
The science behind AI
Scientific excellence in AI
Faster and cheaper
Network and content
The global data infrastructure
Data at the centre
Cloud and software
At the digital frontier
Five economies account for most developments at the digital technology frontier. Technologies take time to develop and mature and may follow different development and adoption paths. Technologies that have several applications may at some point experience accelerated development. Digital technologies are an example of fast-accelerating technologies.
ICT products such as mobile phones and computers are renowned for their complexity and modularity, their rapid obsolescence, and their reliance on a wide array of continuously evolving technologies (OECD, 2017). The OECD used a data-mining approach to monitor the extent to which different ICT fields emerge and develop, and to identify fast-accelerating technologies. Over 2013-16, five economies accounted for 72% to 98% of the top 25 fast-accelerating digital technologies. Japan and Korea contributed to the development of all ICT fields in which development accelerated during this period, together accounting for 7% to about 68% of all patenting activities in these ICT fields. The United States led the development of digital technologies related to aircraft traffic control (53%) and to algorithms based on biological models (43%) and mathematical models (39%). The People’s Republic of China (hereafter “China”) was among the top-five economies developing technologies in most fast-accelerating ICT fields, and was particularly active in control arrangements (31%) and wireless channel access, as well as in network and access restriction techniques (21%). A few European economies, namely Sweden, Germany and France, also featured among the top five players in emerging digital technologies.
1. Top players in emerging digital technologies, 2013-16

Source: OECD, STI Micro-data Lab: Intellectual Property Database, http://oe.cd/ipstats, January 2019. See 1. StatLink contains more data.
1. Data refer to IP5 families by filing date and the applicant’s residence, using fractional counts. Patent “bursts” correspond to periods characterised by the sudden and persistent increase in the number of patents filed by International Patent Classification (IPC) classes. Top patent bursts are identified by comparing the filing patterns of all IPC classes. The intensity of a patent burst refers to the relative strength of the observed increase in filing patterns. Only IPC classes featuring a positive burst intensity from 2010 are included. Data for 2015 and 2016 are incomplete.
Descriptions of IPC groups are available at: http://web2.wipo.int/classifications/ipc/ipcpub.
Identifying acceleration in technological development
Patents protect novel technological inventions. Patent data can thus help investigate a number of policy-relevant issues related to innovation and technological development. A data-mining approach called “DETECTS” (see Dernis et al., 2016) exploits information contained in patents to identify technologies in which development increases sharply compared to previous levels and to the development of other technologies, and maps the time it takes for such dynamics to unfold. A technology field is said to accelerate when a substantial increase in the number of patents filed in that field is observed. Monitoring fields in which accelerations of technological developments occur is important for policy making, as developments tend to persist in these areas over the short and medium term. Furthermore, information contained in patents about the technologies themselves and the geographical location of patent owners and inventors enables the identification of economies leading such technology developments, and can shed light on the generation of new technological trajectories arising from the cross-fertilisation of different technologies (e.g. ICT and environmental technologies).
Digital technology waves
The general-purpose nature of digital technologies increases their development speed and sustains their acceleration over time as they find new areas of application. Analysis focusing on ICT-related fields over the period 2005-15 reveals the sequence of technological developments occurring during that decade, the extent to which some ICT fields saw development accelerate and the length of the period during which such accelerations were sustained. In the mid-2000s, activities burgeoned in the field of data-processing equipment, whereas the late 2000s saw accelerations in semi-conductor and wireless communications. Since 2012, inventions patented in the top-five Intellectual Property offices worldwide (IP5) and related to digital data transfer experienced a persistent acceleration of unprecedented intensity, at an average growth rate of 19% a year. During the last part of the period considered, domains linked to organic materials devices, image analysis, connection, transmission, or security management experienced accelerated development. Compared to those observed at the beginning of the period, recent accelerations in the pace of development seem to last longer and consist of a higher number of inventions.
2. Intensity and development speed in ICT-related technologies, 2005-15

Source: OECD, STI Micro-data Lab: Intellectual Property Database, http://oe.cd/ipstats, January 2019. See 1. StatLink contains more data.
1. Patent “bursts” correspond to periods characterised by a sudden and persistent increase in the number of patents filed in ICT-related technologies. Top patent bursts are identified by comparing the filing patterns of all other technologies. The intensity of a patent burst refers to the relative strength of the observed increase in filing patterns. Data refer to IP5 patent families, by filing date, using fractional counts. Patents in ICT are identified using the list of IPC codes in Inaba and Squicciarini (2017). Only the top 25 ICT-related patent classes featuring a positive burst intensity from 2005 are included. Data for 2015 and 2016 are truncated.
Descriptions of IPC groups are available at: http://web2.wipo.int/classifications/ipc/ipcpub.
Descriptions of IPC groups are available at: http://web2.wipo.int/classifications/ipc/ipcpub.
How to read this figure
A larger bubble indicates a greater intensity of acceleration (i.e. the pace at which the technology accelerates), and the different shades indicate different technologies that start to accelerate at the same time. The X axis indicates the year in which technologies start to accelerate, and the Y axis displays the number of years during which technologies continued to burst. For example, acceleration in the development of patented technologies related to data-processing equipment (on the left) was first observed in 2005 (X axis), and lasted for four years (Y axis), until the end of 2009. Bubbles located along the diagonal line on the right-hand side of the figure represent technologies that were still developing at an accelerated pace at the end of the sample period. Among ICT technologies that began to accelerate in 2012 are those related to digital data transfer, organic materials devices, image analysis and connection management. While developments in these fields were characterised by a varying number of patents – with digital data transfer accounting for the highest amount – inventive activities in all fields continued to occur at an accelerated pace up to the end of 2015.
AI technologies
AI-related inventions have accelerated since 2010 and continue to grow at a much faster pace than is observed on average across all patent domains. Artificial intelligence (AI) is a term used to describe machines performing human-like cognitive functions (e.g. learning, understanding, reasoning, or interacting). It has the potential to revolutionise production as well as to contribute to tackling global challenges related to health, transport, and the environment (OECD, 2017). AI developments began in the 1950s, when pioneers in computing, mathematics, psychology, and statistics set out to solve some concrete problems in order to make machines that can “think” (Turing, 1950). These included playing games, classifying images, and understanding natural language. Technologies developed by AI researchers became extremely valuable in themselves, as well as for many other purposes. One such technology is machine learning (ML), a relatively recent development in the history of AI technologies that uses a statistical approach to identify patterns in large datasets. ML and other AI-related developments, coupled with technologies such as Big data analytics and cloud computing, are strengthening the potential impact of AI (OECD, 2019a).
The multifaceted nature of AI and its rapid evolution over time make it challenging to clearly identify and measure AI-related technological developments. An experimental three-pronged approach is pursued here, whereby patent classification codes, key words obtained from an analysis of AI-related scientific publications, and a combination of the two, have been used to search patent documents to identify AI-related inventions protected through patents. About one-third of AI patents were identified using patent classification codes only, whereas the bulk of such inventions was detected through the joint use of codes and keywords.
Data on inventions protected in the top-five Intellectual Property offices (IP5) worldwide show that the development of AI-related technologies continued at a sustained pace over the period 1990-2016. The number of AI-related patents grew more than tenfold during the period considered, a much faster pace than that observed on average across all patent domains. As measured by the patent data, technological developments in AI have accelerated since 2010.
3. Technology developments in artificial intelligence, 1990-2016

Source: OECD, STI Micro-data Lab: Intellectual Property Database, http://oe.cd/ipstats January 2019. See 1.
1. Patents related to AI are identified using an experimental combination of International Patent Classification (IPC) codes and keyword searches extracted from patent documents. Data refer to IP5 patent families by earliest filing date and the applicant’s residence, using fractional counts. IP5 patent families are patents filed in at least two offices worldwide, one of which is one of the five largest IP offices: the European Patent Office (EPO), the Japan Patent Office (JPO), the Korean Intellectual Property Office (KIPO), the US Patent and Trademark Office (USPTO) and the National Intellectual Property Administration of People’s Republic of China (NIPA). Data for 2015 and 2016 are estimates based on available data for those years.
How can AI-related developments in science and technology be tracked?
Artificial Intelligence refers to a machine-based system that is capable of influencing the environment by making recommendations, predictions or decisions for a given set of objectives. It does so by utilising machine and/or human-based inputs to: i) perceive real and/or virtual environments; ii) abstract such perceptions into models manually or automatically; and iii) use model interpretations to formulate options for outcomes (OECD, 2019b). Measuring the development of AI is challenging as the boundaries between AI and other innovations are blurred and change over time. Experimental work by the OECD and the Max Planck Institute for Innovation and Competition (MPI) relies on a three-pronged approach aimed at measuring AI developments in: science, as captured in scientific publications; technological developments, as proxied by patents; and software, in particular open source software. The approach entails identifying documents (publications, patents and software) which are unambiguously AI-related, using expert advice and assessing the similarity of other documents to those unambiguously considered as AI-related. The patent-based approach initially developed by the OECD and MPI has been further refined through work carried out under the aegis of the OECD-led Intellectual Property (IP) Statistics Task Force, and benefitting in particular from the advice of experts and patent examiners from IP Australia, the Canadian Intellectual Property Office (CIPO), the European Patent Office (EPO), the Israel Patent Office (ILPO), the Italian Patent and Trademark Office (UIBM), the National Institute for Industrial Property of Chile (INAPI), the United Kingdom Intellectual Property Office (UK IPO), and the United States Patent and Trademark Office (USPTO).
AI-related applications
AI-related innovations are being applied to a wide range of fields. An examination of the technology fields on which AI-related patents rely shows that AI technologies are naturally rooted in computer technologies, and are frequently associated with developments in audiovisual technologies, IT methods, and medical technologies. Among the variety of technological areas most often combined with AI are pattern recognition, image analysis, and speech recognition. Specific types of algorithm (e.g. for biological models, knowledge-based systems, and machine learning) accounted for about one-fifth of AI-related developments during the period 2012-16. Image analysis, biological model algorithms and other computational models are among the areas showing an increasing reliance on AI-related components.
4. Top fields of application of AI-related technologies, 2012-16

Source: OECD, STI Micro-data Lab: Intellectual Property Database, http://oe.cd/ipstats January 2019. See 1. StatLink contains more data.
1. Patents related to AI are identified using an experimental combination of International Patent Classification (IPC) codes and keyword searches extracted from patent documents. Data refer to IP5 patent families by earliest filing date and technology fields, using fractional counts. IP5 patent families are patents filed in at least two offices worldwide, one of which is one of the five largest IP offices: the European Patent Office (EPO), the Japan Patent Office (JPO), the Korean Intellectual Property Office (KIPO), the US Patent and Trademark Office (USPTO) and the National Intellectual Property Administration of People’s Republic of China (NIPA). Patents are allocated to application fields on the basis of their International Patent Classification (IPC) codes, following the concordance provided by WIPO (2013), revised in 2018. Data for 2015 and 2016 are incomplete.
5. Top technologies combined with artificial intelligence, by field of application, 2012-16

Source: OECD, STI Micro-data Lab: Intellectual Property Database, http://oe.cd/ipstats January 2019. See 1. StatLink contains more data.
1. Patents related to AI are identified using an experimental combination of International Patent Classification (IPC) codes and keyword searches extracted from patent documents. Data refer to IP5 patent families by earliest filing date and IPC codes, using fractional counts. IP5 patent families are patents filed in at least two offices worldwide, one of which is one of the five largest IP offices: the European Patent Office (EPO), the Japan Patent Office (JPO), the Korean Intellectual Property Office (KIPO), the US Patent and Trademark Office (USPTO) and the National Intellectual Property Administration of People’s Republic of China (NIPA). Patents are allocated to application fields on the basis of their International Patent Classification (IPC) codes, following the concordance provided by WIPO (2013), revised in 2018. Data for 2015 and 2016 are incomplete.
Mapping AI companies
AI companies in different industries are developing and applying a variety of AI-related technologies. Artificial intelligence (AI) is permeating all sectors of the economy. However, little is known about the specific types of AI technologies and approaches being used in each sector, or the purposes for which they are being developed.
In 2018, there were about 6 000 AI-related companies in the United Kingdom alone, according to data from Glass AI, a company that interprets open web text (i.e. sentences and paragraphs) at scale. About 2 800 of these companies make explicit mention of AI activities on their website. These companies appear to combine different AI-related technologies and approaches, depending on their field of application or area of activity. For instance, about 400 companies focus on deep learning, and rely on automation-related technologies and, to a lesser extent, data analytics. About 300 companies currently advancing the use of AI in robotics, the Internet of Things (IoT) and virtual reality (VR) are concentrating on automation and, to a lesser extent, natural language processing. About 250 AI companies are directing their attention to analytics coupled with recognition-related technologies with a view to developing e-commerce-related AI technologies. A similar number of companies rely on different combinations of the same technologies for data mining and business solution-related developments.
6. AI-related companies in the United Kingdom, by focus of activity, 2018

Source: OECD calculations based on Glass.ai data, January 2019. See 1. StatLink contains more data.
1. PC1 and PC2 are calculated on the basis of the Jensen-Shannon divergence and using a principal components algorithm. The number of companies are based on companies mostly active in a given topic, using simple counts.
Mapping AI technologies and applications through topic modelling
Glass AI identifies AI companies by searching for AI-related keywords across an organisation’s website, including in sections such as “News”, “About us” and so on. The bubbles in the chart and the names on the axis are the outcome of a topic modelling exercise. This was performed on the business descriptions of 2 800 companies in the Glass sample that explicitly state on their website that they are active in AI. Based on the Latent Dirichlet Allocation algorithm and Gibbs sampling, the topic modelling exercise grouped companies into nine broad themes (“Topics”). Topics were identified on the basis of the frequency and combination of words contained in the business description of the companies, and their probability of belonging to one or more of the topics (with the sum of probabilities equal to 1). The topics represented on the axis were labelled on the basis of principal component analysis and the frequency with which words featured together in a given topic. The size of the bubbles mirrors the number of companies active in any of the topics identified.
AI companies in key sectors
Companies engaging in AI-related activities belong to a wide range of sectors. More insights about the types of AI technologies that these companies are developing and applying can be gained by focusing on a few key sectors of the UK economy. In particular, Financial services, Professional services, and ICT manufacturing and service activities accounted for 22.7% of total employment (7.3 million persons, up from 6.0 million in 2010) and for 53% of investment (i.e. gross fixed capital formation, GFCF) in ICT equipment in 2017.
Of the 2 800 UK companies in the Glass AI sample which stated that they were actively pursuing AI-related activities, 829 appear to operate in ICT manufacturing and services activities, 693 in Professional services activities and 162 in Financial and insurance activities, representing 60% of the sample. The other 40% is distributed across ten sectors ranging from agriculture to real estate and construction. Some of these companies are developing and using several types of AI-related technologies, whereas others appear to be focused on a specific area. In addition, different technologies appear to be developed to relatively different extents. UK AI-active companies in ICT manufacturing and services are focusing their efforts on technologies related to language processing, business solutions, and deep learning. Companies in Professional services are especially concerned with language processing, image recognition and robotics, Internet of Things (IoT), and virtual reality-related technologies. Finally, Finance and insurance companies appear to be especially active in autonomous vehicles-related technologies, deep learning, robotics, IoT, and virtual reality.
7. AI-related technologies developed by UK companies, by sector, 2018
Assigning firms to sectors
The companies in the Glass AI sample have been assigned to industrial sectors on the basis of a semantic analysis of the text provided on their websites. Financial services correspond to sector 64-66 “Financial and insurance activities” (i.e. section K) of ISIC Rev.4; Professional services correspond to sectors 69-75, 78 and 80-82 of ISIC Rev.4; and ICT manufacturing and service activities correspond to sectors 26, 61 and 62-63 of ISIC Rev.4 classification.
The science behind AI
Scientific advances related to AI are not confined to computer sciences. Research in the field of artificial intelligence (AI) has aimed for decades to allow machines to perform human-like cognitive functions. Breakthroughs in computational power, the availability of data, and algorithms have raised the capabilities of AI, with its performance increasingly resembling that of humans in some narrow fields. Such advances enabled IBM’s Deep Blue computer to beat world chess champion Garry Kasparov in 1997 and have allowed computers to distinguish between objects and text in images and videos with growing accuracy (OECD, 2017). Over the last two decades, different scientific domains have contributed to advances in AI. Text mining of keywords shows that computer science is the scientific field making the largest contribution to AI-related science: it accounts for slightly more than one-third of all AI-related documents published between 1996 and 2016. More than a quarter of all AI-related scientific publications and conference proceedings have appeared in engineering outlets and close to 10% in mathematics. About 25% of the science involving AI (drawing on it or contributing to its general advancement) occurs in a wide array of other scientific disciplines, including physics and astronomy, earth and planetary sciences, materials science, medicine and environmental science, among others. This demonstrates the pervasiveness of this new data-driven paradigm.
8. The science behind AI, 1996-2016

Source: OECD calculations based on Scopus Custom Data, Elsevier, Version 1.2018, January 2019. See 1.
1. Fields are based on the SCOPUS 2-digit All Science Journals Classification (ASJC).
This is an experimental indicator. AI-related documents (among Scopus-indexed articles, reviews and conference proceedings) are identified using a list of keywords to search the abstracts, titles and keywords of scientific documents. These keywords are selected on the basis of high co-occurrence patterns with terms frequently used in journals classified as AI by Elsevier. Only those documents with two or more keywords were considered AI documents. For more details, see the forthcoming working paper at: https://doi.org/10.1787/18151965.
Identifying AI-related science
The bibliometric analysis shown here is based on data from Elsevier’s Scopus®, a large abstract and citation database of peer-reviewed literature, which includes scientific journals, books and conference proceedings. The latter are particularly important in the case of emerging fields, helping to provide a more timely picture as developments are often discussed at peer-reviewed conferences prior to being published in other literature. The indicators shown here are experimental. AI-related documents (among Scopus-indexed articles, reviews and conference proceedings) are identified using a list of keywords searched for in the abstracts, titles and author-provided keywords of scientific documents. These keywords have been selected on the basis of high co-occurrence patterns with terms frequently used in journals classified as AI-focused by Elsevier. As some selected keywords may be used in non-AI settings, only those documents with two or more keywords were considered as being AI-related in order to prevent the inclusion of documents too weakly related to AI. More precise indicators could be obtained through text mining analysis of full text, but this is currently impossible for a comprehensive and representative set of scientific publications. Full text analysis could potentially allow a better distinction between AI use in science and the development of knowledge about AI itself.
Scientific excellence in AI
China produces the most AI-related scientific publications and is improving their quality. Scientific publishing related to AI has experienced a remarkable expansion over the 2006-2016 period. Since 2006, the annual volume of AI-related publications has grown by 150%, compared to 50% for the overall body of indexed scientific publications. China became the main producer of AI-related scientific publications as far back as 2006, and in 2016 had a global share of 27%. In turn, the shares accounted for by the EU28 and the United States declined over the same period to 19% and 12%, respectively. The fast growth of AI-related publishing in India (11% of the world total in 2016) has contributed to the growing share accounted for by other economies. As in other areas, different AI-related scientific publications have different levels of citation “impact”, so it can be misleading to count all publications equally. The EU28 and the United States are still responsible for the greatest shares of highly cited AI-related publications (i.e. those featuring among the world’s top 10% most cited publications). Their shares, however, declined between 2006 and 2016, from 29% to 25% for the EU28 and from 31% to 21% for the United States. China, India, Iran and Malaysia all more than doubled their share of top-cited AI publications over the past decade.
9. Trends in scientific publishing related to artificial intelligence, 2006-16
10. Top-cited scientific publications related to AI, 2006 and 2016

Source: OECD calculations based on Scopus Custom Data, Elsevier, Version 1.2018 and 2018 Scimago Journal Rank from the Scopus journal title list (accessed March 2018), January 2019. See 1. StatLink contains more data.
1. This is an experimental indicator. AI-related documents (among Scopus-indexed articles, reviews and conference proceedings) are identified using a list of keywords to search the abstracts, titles and keywords of scientific documents. These keywords are selected on the basis of high co-occurrence patterns with terms frequently used in journals classified as AI by Elsevier. Only those documents with two or more keywords were considered AI-related documents. For more details, see the forthcoming working paper at: https://doi.org/10.1787/18151965.
“Top-cited publications” are the 10% most-cited papers normalised by publication journal scientific field(s) and type of document (articles, reviews and conference proceedings). The Scimago Journal Rank indicator is used to rank documents with identical numbers of citations within each class. This measure is a proxy indicator of research excellence. Estimates are based on fractional counts of documents by authors affiliated to institutions in each economy. Documents published in multidisciplinary/generic journals are allocated on a fractional basis to the ASJC codes of citing and cited papers.
Faster and cheaper
Thanks to sustained technological progress, ICT products have become much cheaper and more powerful over time. These trends, coupled with the continuous widening of network availability, functionalities, applications and content have played a major role in promoting the use of ICT products. From 2000 to 2018, while consumer prices increased about 45% on average in the OECD area, the prices of communication-related products (i.e. excluding IT and media) decreased by more than 20%. Price dynamics vary between ICT goods and services. In the Euro area and the United States, for which detailed indices can be computed, prices decreased by 10% to 25% for telecommunication services and fell 80% or more for ICT goods.
The capabilities of digital products are rapidly evolving. Since the 1970s, the number of transistors per chip – a traditional way of considering improvements in computing power – has followed “Moore’s law” by roughly doubling capacity every two years. This has been accompanied by concurrent miniaturisation: the length of the “transistor gate” is now around 7 nm, 1 500 times smaller than in the early 1970s, resulting in increased processing speed and improved energy efficiency. Storage capacity has also increased enormously, with the commercial price per Gigabyte diminishing from about USD 10 in 2000 to below USD 0.3 in 2018.
11. Consumer price indices, all products and ICT goods and services, OECD, Euro area and United States, 2000-18

Source: OECD Consumer Price Indices (CPIs) Database; Eurostat, Harmonised Index of Consumer Prices (HICP) Statistics and United States Bureau of Labor Statistics, CPI-All Urban Consumers (Current Series), January 2019. See 1. StatLink contains more data.
1. For the OECD, the following data are not included in calculations: Canada (whole series), Iceland, Mexico and the United States (until 2002), Turkey (until 2004) and New Zealand (until 2007).
National indices are used for Finland and the United States until 2009, Poland until 2005, the United Kingdom until 2004 and Hungary until 2006.
Data for 2018 are limited to October.
For the Euro area, data refer to Belgium, France, Germany, Italy, the Netherlands and Spain (representing 90% of the total Euro area) until 2014.
For the United States, data for ICT equipment include Internet services until 2008. Country and item weights were used for aggregations and estimations.
12. Computing power and cost of storage, 1970-2018 and 1982-2018

Source: OECD based on Wikipedia, “Transistor count”, www.wikipedia.org/wiki/Transistor_count; “A history of storage cost”, www.mkomo.com/cost-per-gigabyte; “Disk drive prices 1955-2018”, www.jcmit.net/diskprice.htm, January 2019. See 1.
1. The transistor count is the number of semiconductor devices on an integrated circuit (IC). Transistor count is the most common measure of IC complexity, although there are caveats. For instance, the majority of transistors are contained in the cache memories in modern microprocessors, which consist mostly of the same memory cell circuits replicated many times.
The process node (also called the technology node, process technology or simply node) refers to a specific process in semiconductor manufacturing. The size of the elements of the structure of a chip are measured in nanometres.
Network and content
Infrastructure capacity is increasing, as is content. Mobile connectivity has undergone major improvements starting with the launch of 3G at the beginning of the millennium and followed by the introduction of 4G in the early 2010s. As a result, most OECD countries currently enjoy broad coverage, and 5G is now in the early stages of roll out. Wired connections have also become more widespread while deploying faster technologies such as fibre. According to commercial sources (Akamai and M-Lab), the average (fixed and mobile combined) global Internet connection speed increased from 2 Mbps to more than 9.1 Mbps between 2011 and 2018. Meanwhile, the total number of websites grew from about 100 million in 2006 to more than 1.6 billion in 2018, according to Netcraft. The number and growth of top-level domains (TLDs) associated to websites provides an indication of the increased content hosted by the Internet. TLDs grew from just above 90 million in 2005 to 280 million in 2014, and reached close to 350 million in the third quarter of 2018. By that time, the .com generic domain (gTLD) had reached 135 million, followed by the China (.cn) country domain (ccTLD), the volume of which doubled in four years to reach 23 million.
13. The increasing capacity of Internet infrastructure, 2005-18

Note: These sources give only a partial perspective on developments in Internet speed. Please see pages 3.3 and 3.7 on the strengths and limitations of such sources.
Source: OECD based on Akamai, MLAB, Netcraft and Internet live stats, January 2019. See 1.
1. Speed data for 2018 refer to the period June 2017 to May 2018.
Top-level domains data for 2018 are limited to October 2018.
14. The increasing content hosted on the Internet, 2018

Note: Top-level domain (TLD) refers to the last segment of a domain name or the part that follows immediately after the “dot” symbol. TLDs are mainly classified into two categories: generic (g) and country-specific (cc) TLDs. In recent years, new gTLDs have been added to the older (“legacy”) .com, .org, .net, .gov, .biz and .edu. TLDs.
Source: OECD based on Council of European National Top-level Domains Registries (CENTR), Verisign, Domaintools.com and national authorities, January 2019. See 1. StatLink contains more data.
1. Data sources are the following:
For Australia, China and Tokelau, data come from Verisign: www.verisign.com/en_GB/domain-names/dnib/index.xhtml.
For Europe and Canada: https://stats.centr.org; for Spain: www.dominios.es/dominios; for Japan: https://jprs.co.jp/en/stat; for India: https://registry.in; for the United States: www.about.us/resources/statistics; for Korea, Poland and South Africa: http://research.domaintools.com/statistics/tld-counts.
For all gTLDs except .biz: https://stats.centr.org; for .biz: www.statdns.com.
For all 2005 TLDs: www.oecd.org/sti/ieconomy/37730629.pdf.
Data for “others” are based on OECD estimates by difference.
The global data infrastructure
Capacity for data transmission is increasing everywhere, including developing economies. Cross-border data flows enable businesses to effectively co-ordinate their, supply, production, sales, after-sales, and research and development processes in global markets. At an inter-continental level, the bulk of data is transferred via sub-marine cables, making them a useful indicator of the volume of cross-border data flows. The Submarine Cable Map is an online resource provided and regularly updated by TeleGeography. According to these data, there were around 448 submarine cables in service in 2018, with a total length of roughly 1.2 million kilometres (Krisetya, Lairson and Mauldin, 2018a). Meanwhile, global Internet bandwidth reached 393 Tbps (Terabytes per second) in 2018, two-thirds of which has been deployed since 2014. Africa experienced the most rapid growth, with a compound annual rate of 45% between 2014 and 2018 (Krisetya, Lairson and Mauldin, 2018b). In 2018, 126 Tbps of capacity was inter-regional and 265 Tbps connected countries within each of the major world regions (see the Global Internet Map 2018).
15. Global Internet Map, 2018

Source: Global Internet Map 2018, TeleGeography 2018, downloaded 17 January 2019.
How to read this map
The map depicts international Internet bandwidth between metropolitan areas. This includes one route for each country where the route’s bandwidth is 2 Gbps or greater and top routes in countries with greater bandwidth. The circular area of each regions’ projection is proportional to the bandwidth connected to cities within that region. Internet bandwidth data only includes capacity from providers that operate international bandwidth links. Private network IP links are excluded. Data are from mid-2018.
Data at the centre
Data ownership is concentrating as the volume of data continues to rise, but its overall value remains unknown. International bandwidth usage is increasingly shifting towards content providers such as Amazon, Google, Facebook and Microsoft among others. Over the past few years, their share of international bandwidth usage has risen significantly, reaching 40% in 2017, on par with traditional Internet backbone providers (Mauldin, 2017). To ensure ever-increasing demand for their services, content providers themselves have become large players in the development of global data infrastructure, for example through the construction of submarine cables and data centres.
Data centres - servers that can be used exclusively by a firm (private cloud) or rented on demand from cloud service providers - enable the storage of data, as well as remote computing via the Internet (cloud computing). The growing importance of data analytics – the analysis of Big data coming from ubiquitously networked end-user devices and the Internet of Things (IoT) – has added to the value and growth of data centres. CISCO (2018), estimates that Global Internet Protocol (IP) traffic in 2021 will be double that of 2018, approaching 400 Exabytes/month (1 EB equals 1 0006 bytes) in 2022; and that global traffic from data centres in 2021 will also almost double, to more than 20 Zettabytes (1 ZB equals 10007 bytes). Big data is expected to represent about 3 ZB of traffic within data centres, having increased almost five-fold since 2016 (Cisco, 2018).
Data flows, including across borders, can take place within businesses, between businesses (B2B), between businesses and consumers (B2C) and between machines (M2M). CISCO estimates that, in 2022, mobile networks will contribute 20% of global IP traffic, more than twice their share in 2017. Correspondingly, 41% of global traffic will originate from smartphones, up from 18% in 2017. M2M traffic is expected to grow from 3.8 EB to 25.4 EB per month and from 3.1% to 6.4% of global IP traffic. Internet video services function as the key driver of global traffic growth, accounting for about three-quarters of consumer IP traffic (itself more than four-fifths of global traffic). According to CISCO, this share will approach 82% in 2022, even without accounting for managed-IP traffic corresponding to video-on-demand. However, it is highly unlikely that video traffic explains the majority of value created from data flows. Indeed, many productive uses of data flows, such as the co-ordination of global value chains or cloud computing, may generate relatively little data traffic.
16. Global data centre traffic, by type and Consumer Internet Protocol (IP) traffic, by sub-segment, 2015-22

Note: “To data centre” refers to traffic flowing from one data centre to another, for example, moving data between clouds, or copying content to multiple data centres as part of a content distribution network. “To user” refers to traffic that flows from the data centre to end users through, for example, streaming video to a mobile device or PC. “Within data centres” refers to traffic that remains within a data centre, for example, moving data from a development environment to a production environment within a data centre, or writing data to a storage array.
Source: OECD calculations based on Cisco Global Cloud Index 2016-2021 and Cisco Visual Networking Index 2017-2022, January 2019. See 1. StatLink contains more data.
1. Cisco Global Cloud Index 2016-21 is available at: www.cisco.com/c/en/us/solutions/collateral/service-provider/global-cloud-index-gci/white-paper-c11-738085.html.
Cisco Visual Networking Index 2017-22 is available at: www.cisco.com/c/en/us/solutions/service-provider/visual-networking-index-vni/index.html#complete-forecast.
How data travels through the Internet
The Internet is a global network of computers, each with its own Internet Protocol (IP) address (an identifier of a device on the Internet). When a file is sent from a computer in Country A to a recipient in Country B it is first broken down into different “packets”. These are like little parcels of information marked with the IP address of the sender, that of the recipient, and a code identifying the sequence in which the packets are to be reassembled at the destination. Once the packets are ready, they leave the origin computer, crossing different networks and taking different routes to their destination. Routers, the traffic wardens of the Internet, guide the packets across networks, ensuring that, at each step, they take the shortest or least congested route. Once the packets arrive at their destination, the computer assembles these according to their pre-specified sequence. If a packet is missing, a signal is sent for that packet to be re-sent. When flowing between two countries, packets take different routes often crossing multiple third countries. The ultimate origin and destination of data flows is often a technical issue. For example, firms use mirror sites, which replicate webpages in different countries, to increase the speed of data transfers. In some instances, what might seem to be a domestic transfer, involves a cross-border flow (Casalini and López González, 2019).
Cloud and software
The growth of cloud services has gone hand-in-hand with the diffusion of high-speed fibre broadband, the rise in quality of key storage and data processing technologies, and the availability of software tools. For firms with a high-speed broadband connection, the ability to access cloud services using a “pay on demand” function became possible with the advent of Elastic Computing Cloud, introduced by Amazon Web Services (AWS) in 2006. From 2010, adoption began to rise quickly due to the increased number of cloud providers (e.g. Google, IBM, Microsoft, and Oracle) followed by an associated decline in the price of such services (DeStefano, Kneller and Timmis, 2018). Cloud services mark a paradigm shift in ICT provision, allowing businesses and individuals to access on-demand IT services over a network, without the need to make large up-front investment in physical ICT capital. Information on companies’ use of cloud computing services is still difficult to isolate in official data, at least on a basis that is comparable across countries. The OECD Inter-Country Input-Output (ICIO) table (2018 update), however, enables the identification of an industry’s purchases of information services produced by companies operating in “Computer programming, consultancy and related activities” and “information services” industries which include producers of cloud computing services.
Industry-level purchases of ICT service intermediates, as a share of value added in each industry, grew more between 2005 and 2015, than software investment and investment in ICT equipment. The differentials in growth rates suggest a change in the pace of “IT outsourcing” over the decade, i.e. the growth rate in ICT intermediate services was driven mainly by purchases from foreign suppliers. By reducing or avoiding the large fixed costs associated with investment in new ICT equipment, purchases of IT services allow companies to reduce the costs of experimenting with new technologies, scaling up, and adapting technology use to the business cycle. These effects are likely to be more prominent for small, young and credit-constrained firms.
17. ICT investment and expenditure in ICT intermediate services, 2005-15

Note: Intensities are calculated by dividing investment and expenditure by the industry-country (deflated) value added. Year-on-year growth rates in industry-country values are averaged over 33 OECD countries and industries, while accounting for the unbalanced nature of the sample. As intensities are calculated as flows of investment or intermediate consumption divided by the industry’s value added, the reported growth rates are not driven by the growth of production in the industries themselves. As all values are separately deflated, growth rates do not reflect price changes in the period either.
Source: OECD calculations based on Intan-Invest data, www.intan-invest.net; EUKLEMS, www.euklems.net; OECD Inter-Country Input-Output (ICIO) Database, http://oe.cd/icio; OECD Annual National Accounts (SNA) Database, www.oecd.org/std/na; OECD Productivity Database, www.oecd.org/std/productivity-stats and OECD Structural Analysis (STAN) Database http://oe.cd/stan, December 2018. See 1. StatLink contains more data.
1. Nominal investment in ICT tangible capital and software capital by industry and country is sourced from the OECD Annual National Accounts (SNA) Database. ICT investment estimates for Germany and Spain are provisional and sourced from EUKLEMS. Purchases of intermediate ICT services in current prices are sourced from the OECD Inter-Country Input-Output (ICIO) Database. The industry origins of intermediate ICT services are those produced by ISIC Rev.4 Division 62 (Computer programming, consultancy and related activities) and 63 (Information service activities). Both domestically produced and imported intermediates are included. Purchases by all sectors in the economy are considered. Current price investment in ICT tangible capital are deflated using hedonic price series from the OECD Productivity Database. Current price investment in software and databases is deflated using the software deflators developed by Corrado et al. (2012), extrapolated using software hedonic deflators from the OECD Productivity Database, where necessary. Purchases of ICT intermediate services are deflated by the output prices of the ICT-service producing industry in the country. When such output deflators are not available, the G7 un-weighted average deflator for the same industry is applied. Value added figures at the industry-country are sourced from the OECD Structural Analysis (STAN) Database, and complemented with information from the OECD SNA Database when missing. They are deflated using industry-specific deflators from the STAN Database or GDP deflators from the SNA Database when the former are missing.
Intensities are calculated by dividing deflated investment and expenditure by the industry-country value added. Year-on-year growth rates in industry-country values are calculated, then averaged over all countries and industries, accounting for the unbalanced nature of the sample. Growth rates are calculated at the disaggregation level reported in the ICIO. The following countries are included: Australia, Austria, Belgium, Canada, Chile, Czech Republic, Denmark, Estonia, Finland, France, Germany, Greece, Hungary, Ireland, Iceland, Israel, Italy, Japan, Korea, Luxembourg, Latvia, Mexico, the Netherlands, Norway, New Zealand, Poland, Portugal, Slovak Republic, Slovenia, Spain, Sweden, the United Kingdom and the United States.