This chapter covers the case study of Let Food Be Your Medicine (LFYM), a web- and mobile‑based intervention providing consumers with personalised nutrition advice. The case study includes an assessment of LFYM against the five best practice criteria, policy options to enhance performance and an assessment of its transferability to other OECD and EU27 countries.
Healthy Eating and Active Lifestyles
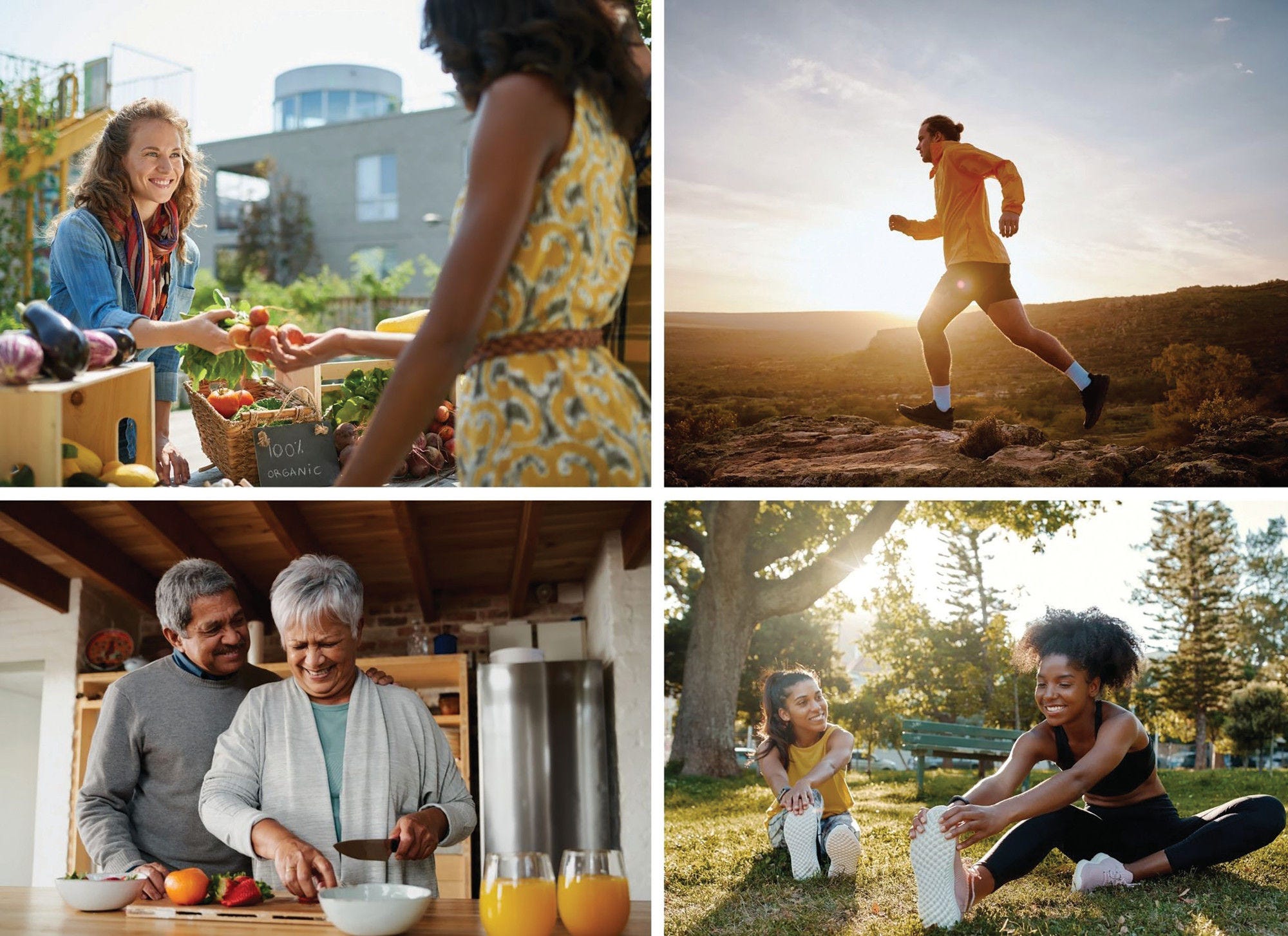
14. Let Food Be Your Medicine
Abstract
Let Food Be Your Medicine: Case study overview
Description: Let Food Be Your Medicine (LFYM) is a web- and mobile‑based intervention providing consumers with personalised nutrition (PN) advice. It is currently being piloted in Romania. Nutritional advice is based off a consumer’s dietary intake (and genetic tests, if provided) compared to recommended levels outlined by the European Food Safety Agency. The app is designed for both preventative and treatment purposes and can cost up between EUR 0‑5/month (USD PPP 0‑7.3), depending on application features.
Best practice assessment: LFYM is currently in its infancy therefore data to quantitatively assess the intervention against the OECD Framework was not possible. Based on evidence for similar digital PN interventions, LFYM is likely to perform well against most criteria (see Table 14.1).
Table 14.1. OECD best practice assessment of LFYM
Criteria |
Assessment |
---|---|
Effectiveness |
PN interventions have found to be effective in improving dietary habits including a reduction in fat intake and high-energy snacks, and an increase in fruit and vegetable consumption |
Efficiency |
Economic evaluations of PN interventions conclude they are generally cost-effective |
Equity |
Interventions requiring users to be digitally literate and have access to the internet or smartphone can potentially exclude disadvantage groups such as the elderly |
Evidence‑base |
The quality of evidence to evaluate LFYM is not available as the intervention is in its infancy The evidence to support personalised nutrition interventions more broadly is strong in areas such the study design and data collection methods |
Extent of coverage |
Mobile apps have an uptake of approx. 2% and a dropout rate of up to 20% with certain groups more prone to exiting |
Enhancement options: to enhance effectiveness and efficiency, targeted online forums to discuss progress with those facing similar health issues could be established alongside frequent motivational messages. In addition, users could be given the option of uploading information on other risk factors such as tobacco consumption. To enhance equity, options to improve access to vulnerable groups are encouraged to boost uptake, for example through targeted promotion. To enhance the evidence‑base, future evaluations using objective measures of body-mass index (BMI) are important given they are considered a final outcome and therefore of key interest to policy makers. Finally, to enhance extent of coverage, LFYM administrators are encouraged to actively promote how data will be used and stored to alleviate privacy concerns.
Transferability: a contextual review of Romania, where LFYM is being piloted, and OECD and non-OECD European countries, indicates the intervention is broadly transferable. For example, compared to Romania, most countries have higher levels of internet use and access among the public and health profession. However, before determining transferability, it is important to collect new types of information including regulation regarding the collection and use of genetic information.
Conclusion: LFYM is in its infancy therefore an in-depth assessment of the intervention against the OECD Framework was not possible, However, given the impact of similar PN digital interventions on health outcomes, LFYM can be considered a “promising” best practice intervention that may be broadly transferable. To further enhance implementation, LFYM administrators could consider options outlined in this case study such as frequent motivational messages.
Intervention description
The majority of interventions to change dietary behaviours are normally applied homogenously across different population groups (e.g. “eat five servings of fruits and vegetables a day”), and, have had limited success (Ordovas et al., 2018[1]; Marsaux et al., 2015[2]; Fallaize et al., 2013[3]). However, in recent years, there has been a growth in personalised nutrition (PN) which uses “individual specific information, founded in evidence‑based science, to promote dietary behaviour change resulting in better health outcomes” (Adams et al., 2019[4]). The rise in the use of PN is a result of (Ordovas et al., 2018[1]; Forster et al., 2016[5]):
A better understanding of how diets impact overall health, including at the individual level
Advances in technology that enable people to record and measure their dietary intake with relative ease
Developments in data analytics enabling information to be readily converted into useful information.
Let Food be Your Medicine (hereafter, LFYM) is a privately run mobile‑ and web-based online tool designed to provide personalised nutritional advice for both prevention and treatment purposes. To operate the tool, users upload information regarding:
Diet: information on diet is collected using the Food4Me food frequency questionnaire covering 157 food items divided into 11 groups (e.g. “meat and fish”, “dairy products”, and “sweets and snacks”). Against each food time, users are asked to report how often the item was consumed using a nine‑point scale ranging from “never” to “6+ a day”, as well as portion size based on a series of pictures (Forster et al., 2014[6]).
Genetic information: participants have the option to purchase a nutrigenetic test, which includes a swab tests. Results from the swab are then analysed with results provide online (Nutricare, 2020[7]). Alternatively, genetic information can be collected from a blood or urine sample.
Medical history: users have the opportunity to directly upload information regarding medical conditions.
Calories, body composition and physical activity: users have the option of uploading information from wearable and other digital devices to track performance. Key examples including physical activity and calorie trackers, as well data from smart scales.1
Using this information, the tool compares nutritional intake with recommended levels provided by the European Food Safety Agency (see Figure 14.1 for example output). Findings from the comparative assessment are used to develop recommendations on how to address any identified nutritional deficiencies or above standard nutrient amounts through a change in diet (i.e. what foods to eat more/less of) and/or supplements (NutriCare, 2019[8]).2 Recommendations provided by the tool are supported by clinical studies published within the academic literature (NutriCare, 2019[8]). Alternatively, upon receiving results, users can book an appointment with their health care provider or nutritionist to discuss changes to their diet.
LFYM is currently undergoing a testing phase where it has been made available to 300 people in Romania. Once publically available, the standard version will be made available for free and the premium version at a cost between EUR 1‑5/month (USD PPP 1.5‑7.3) for individuals and EUR 5‑10/month (USD PPP 7.3‑14.6) for nutritionists (NutriCare, 2019[8]).
Figure 14.1. Diet assessment example
OECD Best Practices Framework assessment
Data from the LFYM isn’t available given the intervention is currently in its testing phase. For this reason, the OECD Best Practice Identification Framework (OECD Framework) was used to assess digital PN interventions more broadly (see Box 14.1 for a high-level assessment of digital PN interventions). Consequently, results from the assessment should only be taken as an indication of how LFYM may perform once rolled-out nationally. Further details on the OECD Framework can be found in Annex A.
Box 14.1. Best practice assessment overview: Personalised nutrition interventions
Effectiveness
Let Food Be Your Medicine is in its testing phase, therefore no data on outcomes is currently available
Analysis of similar digital interventions highlight the potential gains associated with personalised nutrition advice, such as an increase in fruit and vegetable consumption
Efficiency
Economic evaluations of personalised nutrition interventions conclude they are generally cost-effective. For instance, OECD modelling work estimated mobile phone apps targeting diet and exercise will lead to labour cost savings of USD 4 PPP per capita (EUR 3.64), per year in Romania (the country where LFYM is being implemented)
Equity
Once the intervention is rolled-out nationally, it will be made available to everyone with a smartphone or the internet at a monthly cost
Evidence shows mHealth can widen existing health inequalities by restricting access to lower socio‑economic groups, older populations and those living in rural areas
Evidence‑base
The quality of evidence to evaluate LFYM is not available as the intervention is in its infancy
The evidence to support personalised nutrition interventions more broadly is strong in areas such the study design and data collection methods
Extent of coverage
Based on OECD analysis, participation rates for mobile phone diet and exercise apps are approximately 2.2%
Analysis of similar digital PN interventions indicate the dropout rate is around 20%
Effectiveness
Mobile apps and PN interventions have found to be effective in improving dietary habits including a reduction in fat intake and high-energy snacks, and an increase in fruit and vegetable consumption
Smartphone penetration is growing across the world. As of 2017, 80% of the population in high-income countries owned a smartphone with this figure increasing to 82% for low and middle‑income countries (Deloitte, 2017[9]). Mobile phone apps therefore present a promising opportunity to promote healthy lifestyles on a large scale. For example, modelling work by OECD estimated mobile apps targeting diet and physical activity over the period 2020‑2050 will lead to an additional 3.38 life years gained per 100 000 people in Romania (where LFYM is implemented), which was second highest amongst the 36 countries analysed (OECD, 2019[10]). In terms of specific diseases, over the same time period, the model estimates mobile apps could avoid 760 CVDs and 121 diabetes cases in Romania (OECD, 2019[10]).
Advances in technology (e.g. machine learning) partnered with the growing use of smartphone has led to a growth in the use of PN advice. The evidence on PN dates back many years with Kroeze et al., in (2006[11]), summarising key findings from a systematic review of computer-tailored interventions targeted physical activity and dietary behaviour. Their analysis revealed that of the 26 randomised control trials (RCTs) regarding PN, 20 showed statistically significant, positive effects, in particular regarding a reduction in fat intake (Kroeze, Werkman and Brug, 2006[11]). More recently, Forster and colleagues (2016[5]) reviewed the evidence on the impact of PN tools delivered online (web-based), via mobiles as well as through wearable sensor technologies. In total, results from seven RCTs were summarised, four of which recorded significant effects associated with PN:
Sternfeld et al. (2009[12]) found individuals participating in a 16-week tailored healthy eating and physical exercise plan offered online led to a reduction in consumption of trans-fats and saturated fats, and an increase in fruit and vegetable consumption, when compared to the control group. Specifically, four-months post the intervention, the intervention group consumed 0.29 and 0.75 grammes less of trans-fats and saturated fats, and 0.35 additional cups of fruits and vegetables per day (Sternfeld et al., 2009[12]). Individuals, who, at baseline, did not meet recommended dietary targets, saw the greatest improvement.
Springvloet et al. (2015[13]) evaluated the short- and medium-term efficacy of a web-based programme offering tailored nutrition education based on self-reported consumption of fruits, vegetables, high-energy snacks and fat intake. Results from the study found when compared to the provision of generic nutrition information, those who received PN reduced their fat intake and high-energy snacks by more (‑0.28 fat points and ‑0.20 high-energy snacks per day, four-months post the intervention) (Springvloet et al., 2015[13]).
Maes et al. (2011[14]) evaluated the impact of Food-O-Meter, an online tool offering tailored nutritional advice to those aged between 12‑17 years. The study reported modest findings, with fat intake falling for those who are overweight in the intervention group compared to those in the control group.
Ambeba et al. (2015[15]) examined the impact of daily-feedback-messages (DFM) to changes in dietary intake using a two-year RCT involving obese participants. Findings from the RCT indicate those who receive DFM, compared to those who do not, reduce their mean percentage energy intake (‑22.8% versus ‑14%, respectively) and fat intake (‑10.4% versus ‑4.7%, respectively) after 24-months.
Forster et al. (2016[5]) also included the Food4Me study, which represents the most comprehensive PN RCT, to date (Celis-Morales et al., 2017[16]). The Food4Me “proof of principle” study was an internet-based 6-month RCT to assess the impact of PN compared to standard population advice. Participants in the study were divided into one of four groups: group 1) general dietary advice based on European population guidelines; group 2) PN based on individual dietary intake data; group 3) PN based on individual dietary intake and phenotypic data;3 and group 4) PN based on individual dietary intake, and phenotypic and genotypic data (Celis-Morales et al., 2017[16]).
Results from the study found those who received PN (i.e. groups 2‑4) saw improvements in salt and folate intake when compared to group 1. Specifically, the difference in mean change of salt intake for group 2‑4 and group 1 was 0.65 grammes/day, and 29.6µg/day regarding folate consumption (Celis-Morales et al., 2017[16]). The impact of the intervention on other indicators such as fruit and vegetable consumption, waist circumference and BMI also showed positive effects, however, these were not statistically significant (Celis-Morales et al., 2017[16]).
Effectiveness information outlined in this section is largely based on interventions offering PN using self-reported food frequency questionnaires (FFQs). Conversely, LFYM uses genetic information as well as FFQs, therefore, the effectiveness of the LFYM may differ.
Efficiency
Economic evaluations of digital PN interventions found they are generally cost-effective
The economic impact of mobile apps promoting healthy lifestyles (through improved diet and physical activity) was recently modelled by OECD (2019[10]). Overall, the analysis concluded mHealth apps are efficient given they reduce labour costs such as absenteeism and presenteeism. For example, in Romania, it is estimated mHealth apps will lead to labour cost savings of USD 4 PPP per capita (EUR 3.64) annually (OECD, 2019[10]).
Regarding PN interventions specifically, a number of studies evaluated efficiency exist, however, few specifically refer to interventions offering tailored dietary advice based on genetic information (Laddu and Hauser, 2019[17]). Dalziel and Segal (2007[18]) evaluated the economic performance of 10 nutrition interventions, two of which offered PN advice (“Intensive Lifestyle Change to Prevent Diabetes”, considered to have “high-quality evidence” and “Nurse Counselling in GP [General Practice]” with “intermediate quality evidence”). Results from their analysis found both interventions were cost-effective, specifically, Intensive Lifestyle Change to Prevent Diabetes was highly cost-effective with a cost per QALY of USD 1 410 (or EUR 1 2834). Results for Nurse Counselling in GP were expressed as an incremental cost-effectiveness ratio with one additional percentage point of participants eating more than five servings of fruits and vegetables per day costing USD 4 300 (or EUR 3 914). More recently, a systematic review of the cost-effectiveness of personalised interventions with a nutrition component found of the 49 studies analysed, over 70% were cost-effective (Milanne Galekop, 2019[19]).
Equity
Interventions requiring users to be digitally literate and have access to the internet or smartphone can potentially exclude disadvantage groups such as the elderly
LFYM is offered online or via a mobile‑based app at a cost of between EUR 1‑5 (USD PPP 1.5‑7.3) per month (a pared-down version offering standard as opposed to personalised advice is available for free). When the programme is rolled-out nationally, it will be available to everyone with access to a smartphone or the internet.
Previous analyses of mHealth users indicate they are popular among younger, higher-educated populations (Bol, Helberger and Weert, 2018[20]; Azzopardi-Muscat and Sørensen, 2019[21]), therefore, they potentially exacerbate existing health inequities by restricting access to groups in society that would benefit most (Oliveira Hashiguchi, 2020[22]; Jarke, 2018[23]). For example, research undertaken by OECD estimated adults in the highest income quartile are 50% more likely to use the internet to research health information, compared to adults in the lowest income quartile (OECD, 2019[24]). Other groups less likely to use digital health interventions include older populations and those living in rural areas due to factors such as cost (e.g. pay for a smartphone), lower e‑health literacy skills and limited broadband access (Bol, Helberger and Weert, 2018[20]; Azzopardi-Muscat and Sørensen, 2019[21]; Oliveira Hashiguchi, 2020[22]).
Evidence‑base
Evidence to support personalised nutrition interventions are generally strong in terms of study design and data collection methods
The impact of LFYM has not been evaluated as the intervention is in its infancy. Therefore an assessment of the quality of evidence used to evaluate LFYM is not possible. Instead, the evidence‑based section assesses the quality of the Food4Me Study, which represents the most comprehensive research into PN to date (see Table 14.2) (Celis-Morales et al., 2017[16]).
The Food4Me proof-of-principle study utilised a four-arm, web-based RCT across seven European countries to evaluate the impact of different levels of PN on a range of health outcomes. Specifically, the 1 607 eligible participants were randomly allocated into one of the four groups: 1) general dietary advice based on European population guidelines; 2) PN based on individual dietary intake data; 3) PN based on individual dietary intake and phenotypic data;5 or 4) PN based on individual dietary intake, and phenotypic and genotypic data (Celis-Morales et al., 2017[16]).
Using the Quality Assessment Tool for Quantitative Studies, the Food4Me study is rated as having a strong study design (i.e. RCT) and data collection methods (i.e. the Food Frequency Questionnaire was used to measure to dietary intake which was the primary outcome). However, the study was not blinded and seemingly did not adequately control for potential confounders (e.g. socio‑economic status), therefore the study was rated as “weak” in these areas (Effective Public Health Practice Project, 1998[25]).
Table 14.2. Evidence‑based assessment, LFYM
Assessment category |
Question |
Score |
---|---|---|
Selection bias |
Are the individuals selected to participate in the study likely to be representative of the target population? |
Very likely |
What percentage of selected individuals agreed to participate? |
N/A |
|
Selection bias score: Moderate |
||
Study design |
Indicate the study design |
RCT |
Was the study described as randomised? |
36 123 |
|
Was the method of randomisation described? |
36 123 |
|
Was the method of randomisation appropriate? |
36 123 |
|
Study design score: Strong |
||
Confounders |
Were there important differences between groups prior to the intervention? |
1.65 |
What percentage of potential confounders were controlled for? |
Can’t tell |
|
Confounders score: Weak |
||
Blinding |
Was the outcome assessor aware of the intervention or exposure status of participants? |
36 123 |
Were the study participants aware of the research question? |
36 123 |
|
Blinding score: Weak |
||
Data collection methods |
Were data collection tools shown to be valid? |
36 123 |
Were data collection tools shown to be reliable? |
36 123 |
|
Data collection methods score: Strong |
||
Withdrawals and dropouts |
Were withdrawals and dropouts reported in terms of numbers and/or reasons per group? |
36 123 |
Indicate the percentage of participants who completed the study? |
60‑79% |
|
Withdrawals and dropouts score: Moderate |
Source: Effective Public Health Practice Project (1998[25]), “Quality assessment tool for quantitative studies”, https://www.nccmt.ca/knowledge‑repositories/search/14.
Extent of coverage
Mobile apps have an uptake of approximately 2% and a dropout rate of up to 20% with certain groups more prone to exiting
At this stage, the extent of coverage of LFYM is unknown. Estimates of potential participation and dropout rates therefore rely on previous studies of similar interventions.
Research undertaken by OECD revealed around 2.2% of the adult population (15‑64 years) use mobile apps to improve their health (Goryakin et al., 2017[26]). Regarding dropout rates, previous research from the Food4Me study in Europe revealed a dropout rate of approximately 20%, depending on user demographics (e.g. the Food4Me study reported female and participants with obesity were 38% and 125% more likely to dropout, respectively) (Livingstone et al., 2017[27]).
Based on the research summarised above, LFYM could expect a participation rate of approximately 2% and a dropout rate of 20%, depending on the population group.
Policy options to enhance performance
LFYM is an evidence‑based PN intervention aimed at preventing as well as addressing current health problems using latest available technology. The intervention is in its infancy having only been trialled with 300 participants based in Romania. Therefore, data on best practice indicators such as effectiveness, efficiency and equity are not yet available. For this reason, policy options to enhance implementation have been developed based on a “gap analysis” comparing facilitators and barriers to success of similar interventions compared to the design of LFYM.
Enhancing effectiveness
A review of digital PN and other lifestyle interventions revealed a number of key success factors for enhancing effectiveness, many of which are already adopted by LFYM. These include a range of behavioural change techniques and social support mechanisms (see Box 14.2 for further details). Based on a gap analysis comparing these factors against the design of LFYM, several options to enhance effectiveness could be considered:
A new feature being launched in 2021 allowing users to interact with one another online6 is highly encouraged given the proven benefits associated with people feeling supported and part of a community (Hwang et al., 2010[28]). Since a wide range of users will access the online forum (i.e. with different health issues and goals), to maximise the potential of an forum/discussion group, a feature allowing users to more readily connect with others “in the same boat” may be beneficial (e.g. a filtering system allowing users to identify others aiming to lose weight).
The types of information uploaded by participants into the app/web-based portal could be extended to include other risk factors such as alcohol and tobacco consumption (data on physical activity is already allowed through wearable technologies). By self-monitoring consumption of alcohol and tobacco, participants may be more motivated to reduce consumption. However, there are risks associated with uploading personal information, which must be considered. This topic is discussed in further detail under “Enhancing extent of coverage”.
Users who upload information from their wearable device (e.g. smart scales, and calorie and physical activity trackers) can track their performance in meeting specific goals on a frequent basis. To further motivate users, consideration could be given to providing users with either tailored or generic messages to maintain motivation, including to those who do not have access to wearable devices. For example, providing email or text messages to users when they reach a milestone towards achieving their set goal or when they have recorded improvement in their behaviour such as a consistent increase in daily physical activity. The literature indicates messaging should be frequent and be updated regularly, however, it is important that users have the option to reduce messaging as it may cause fatigue (Livingstone et al., 2017[27]).
Box 14.2. Factors impacting digital PN and other lifestyle intervention effectiveness
A summary of evidence regarding PN and other lifestyle interventions revealed the following key success factors:
Content that is frequently “refreshed” limits the possibility of participants becoming desensitised from key messages (Forster et al., 2016[5])
Interventions targeting multiple lifestyle behaviours – e.g. diet, physical activity, tobacco/alcohol consumption – tend to be more successful in changing health outcomes when compared to those targeting a single lifestyle factor (Browne et al., 2019[29]; Schulz et al., 2014[30])
Participants who receive social support (e.g. through an online community group) are more likely to engage with digital lifestyle interventions (Jane et al., 2018[31]; Sharpe, Karasouli and Meyer, 2017[32])
Frequent interaction, such as through daily messages, can help maintain participant motivation levels (Livingstone et al., 2017[27]; Ambeba et al., 2015[15]; Sharpe, Karasouli and Meyer, 2017[32])
Behaviour change techniques such as goal setting, planning, self-monitoring, and feedback are associated with better health outcomes (Browne et al., 2019[29]; Janssen et al., 2013[33]; Forster et al., 2016[5]; Celis-Morales, Lara and Mathers, 2015[34])
The online tool used to access information should be user friendly and therefore easy to navigate, further, it should include easy-to‑understand instructions on how to impute data used to develop PN recommendations to minimise error (Adams et al., 2019[4]).
Higher levels of population health literacy (HL) will enhance the effectiveness of mHealth apps, such as LFYM. HL refers to an “individual’s knowledge, motivation and skills to access, understand, evaluate and apply health information” (Moreira, 2018[35]). When people are health literate they are more likely to act on health information they receive, take greater responsibility for their own health, as well as engage in shared decision-making. Example strategies to boost HL have been outlined in a recent OECD report and include specific actions such as encouraging HL in schools and workplaces, as well as broader efforts to better measure and monitor changes in HL levels (Moreira, 2018[35]).
Improving digital HL, for users and health professionals, will also enhance the effectiveness of LFYM. For health professionals, this can be achieved by: developing digital health competency frameworks; developing concrete guidelines on how to integrate digital health topics into educational and training; and integrating digital skills into continuous professional development programs (OECD, 2019[24]).
Enhancing efficiency
Efficiency is calculated by obtaining information on effectiveness and expressing it in relation to inputs used. Therefore policies to boost effectiveness without significant increases in costs will have a positive impact on efficiency.
Enhancing equity
Overarching policies aimed at reducing barriers to access for disadvantaged groups, such as improving access to broadband, fall outside the scope of responsibilities of LFYM administrators. Nevertheless, policies that could be considered include: targeted promotion campaigns; provision of detailed, tailored, advice on how to use the app and its benefits to disadvantaged groups; as well as piloting LFYM in low-SES communities.
Given NCDs disproportionality affect lower-SES groups, ensuring access to digital PN interventions should be of interest to relevant policy makers given this group stands to benefit most.
Enhancing the evidence‑base
Studies to evaluate the impact of digital PN interventions, to date, have largely relied on intermediate outcomes7 such as changes in fruit and vegetable consumption and fat intake (see assessment of “Effectiveness”). Given LFYM plans to collect data on a final outcome measure – specifically, objective measures of BMI using smart scales – findings from a future evaluation of the intervention should be of high interest to policy makers. However, it is important when evaluating BMI data (and any other outcomes) to control for a range of socio‑economic demographic variables such as education, employment and income, given users of PN are typically wealthier (for example, only a small subset of the population can afford to purchase a smart scale) (Adams et al., 2019[4]). Controlling for gender, age and ethnicity will also be of high interest. Failing to control for individual characteristics may lead to bias results by presenting findings for a specific group as opposed to the wider population.
The ability to control for individual characterises will ultimately depend on the data collection process. For this reason, LFYM administrators could consider additional socio-demographic and health-related questions when signing up to the intervention.
Details on outcome indicators of interest to LFYM and how to collect this data is provided in Box 14.3.
Further details on how to undertake an evaluation can be found in OECD’s Guidebook on Best Practices in Public Health (OECD, 2022[36]).
Box 14.3. Indicators to measure outcomes
Potential indicators to measure the impact of LFYM are listed in Table 14.3, which includes both final and intermediate outcomes. Outcome indicators should be collected at different points in time, in particularly before and after the intervention. However, given LFYM is an ongoing intervention, collection of data during specific stages of the intervention may be more appropriate (e.g. once a year):
Start: Collect data when activities start to take place to get an accurate baseline – for both the intervention and the control group (given a control group is available)
During: Collect data throughout the intervention period instead of only at the end, to understand when the intervention is effective and observe trends such as participation levels
After: Collect data at the end of the intervention and, ideally, some time after, to observe the longer-term impact and determine whether there is a lasting effect once the intervention has ended (this may be possible if participants who drop out of LFYM agree to have their data collected at specific follow-up points in time).
Indicators considered high-priority have been highlighted in Table 14.3. These indicators are universally recognised thereby making it easier to compare the impact of similar interventions implemented in different contexts (e.g. countries).
Table 14.3. Recommended final and intermediate outcome measures
Indicators |
|
---|---|
Final outcomes |
Change in body-mass index (= weight / height2)) Relative reduction in raised blood pressure Change in waist circumference Change in waist-to-hip ratio Change in perceived health status (% of participants in good or very good health) |
Intermediate outcomes |
Percentage of users who consume recommended daily amount of fruits and vegetables (i.e. 5 portions) Percentage of users consuming fruit at least once per day Percentage of users consuming vegetables at least once per day Percentage of population whose free sugar intake is less than 10% of total calorie intake Percentage of the population who consume less than 5grams of salt per day Percentage of the population whose saturated fatty acid intake is less than 10% of total calorie intake (less than 1% for trans fatty acids) Percentage adults (18+) reporting doing at 150min of moderate‑intensity physical activity in a week OR 75min or vigorous-intensity (or a combination of the two)* Percentage population who engage in performance enhancing physical activity at least once a week (aerobic and/or muscle strengthening) Number of steps taken per day Self-efficacy for healthy eating** Self-efficacy for physical activity |
Note: Indicators which are bolded and italicised are considered to be of high priority. *An indicator for physical activity has been included given LFYM offers users the option to upload activity data from wearable technologies. **Self-efficacy refers to an individual’s belief they are capable of changing a certain behaviour (in this example, healthy eating and/or physical activity) – this type of data is collected through self-reported questionnaires (WHO, n.d.[37]).
Enhancing extent of coverage
Policies to enhance the extent of coverage are outlined according to individuals and health professionals.
Individuals
A key component of the LFYM intervention is the use of genetic testing to provide tailored nutritional advice. However, potential participants may be wary of uploading genetic information to a digital device operated by a private company due to potential risks (for example, concerns regarding how data may “be used and abused” by different stakeholders such as insurers and employers) (see Box 14.4 for further details) (Stewart-Knox et al., 2009[38]). A survey of 6 000 individuals across eight European countries found just 28% of participants were willing to undertake genetic testing for PN purposes, with rates lower for younger, healthier individuals (Stewart-Knox et al., 2009[38]). Further, less than a quarter (22%) of participants would not be willing to have a genetic test. For this reason, it is recommended that LFYM administrators provide extensive information and actively promote how genetic data is used and stored in order to alleviate concerns (e.g. keeping abreast of developments in the standard required to collect, protect, share and use genetic data will be important – such as the Future of Privacy Forum) (Adams et al., 2019[4]).
Once the intervention is rolled-out and sufficient data on participant demographics is available, an analysis of participation rates across population groups is encouraged to identify key trends (e.g. higher dropout in certain population groups). Further analysis into key participation trends may be necessary, for example, through stakeholder interviews and surveys, to identify why certain groups are less engaged than others.
Box 14.4. Risks associated with sharing personal health information
Drawing upon two recent OECD reports, key risks associated with uploading personal information are summarised below, with a specific focus on genetic information (OECD, 2019[24]; OECD, 2020[39]). These risks should be considered when rolling out interventions such as LFYM.
Data security threats: health care data has a high economic value, for this reason, it is prone to leakages and hacks.
Monetisation of health data: monetisation of health data is an area of ethical concern, for example, custodians of data may use this information to develop products and/or license access to data to third parties.
Interpretation of results: the use of direct-to-consumer genetic testing to develop tailored treatments (or lifestyle behaviour recommendations) risks presenting individuals with information: they do not understand; is difficult to interpret; or which requires further contextual information. Low levels of understanding can therefore lead to unnecessary emotional distress.
Understanding of how data is used: there is a risk people upload personal health information without realising how their data will be used – for example, due to lengthy terms and conditions and disclaimers. As a result, there is a risk will people upload information for purposes they are not comfortable with.
Health professionals (including dieticians)
A recent study by Chen et al. (2017[40]) examining the use of mHealth apps amongst dieticians in Australia, New Zealand and the United Kingdom revealed 62% of respondents use mHealth apps within their practice. Dieticians not using mHealth apps highlighted the following factors for this choice: 1) no access to a smartphone at work (51% indicated this as a barrier); lack of infrastructure in their practice, such as poor Wi-Fi (42%); and lack of time with patients to recommend apps (26%) (Chen et al., 2017[40]).
Responsibility for addressing these barriers lies with higher-level policy makers (e.g. state/national level), however, barriers that could be addressed by LFYM include “lack of awareness of the best apps to recommend” and “apps [being] too hard to use” (Chen et al., 2017[40]). For example, LFYM administrators could engage with dietetic associations in countries it hopes to launch in as a way to promote the app and its uses. LFYM could also use this opportunity to offer dieticians a free trial in which to get used to the app, which would also provide an opportunity for LFYM administrators to gather feedback on the app’s usability.
Transferability
This section explores the transferability of LFYM from Romania to other OECD and non-OECD EU countries and is broken into three components: 1) an examination of previous transfers; 2) a transferability assessment using publically available data; and 3) additional considerations for policy makers interested in transferring LFYM.
Previous transfers
The LFYM mHealth intervention has, to date, not been transferred to other countries or regions outside Romania, however, mHealth healthy lifestyle apps are common in most developed countries. For example, the WHO as part of its “Be He@althy, Be Mobile” initiative has developed a series of handbooks to help policy makers implement mHealth interventions (WHO, 2021[41]).
Transferability assessment
The following section outlines the methodological framework to assess transferability and results from the assessment.
Methodological framework
Details on the methodological framework to assess transferability can be found in Annex A.
Indicators from publically available datasets to assess the transferability of LFYM are listed in Table 14.4. Please note, the assessment is intentionally high level given the availability of public data covering OECD and non-OECD European countries.
Table 14.4. Indicators to assess the transferability of LFYM
Indicator |
Reasoning |
Interpretation |
---|---|---|
Population context |
||
% of individuals using the internet for seeking health information in the last 3 months |
LFYM is more likely to be successful in a population comfortable seeking health information online |
🡹 value = more transferable |
ICT Development Index* |
LFYM is more likely to be successful in digitally advanced countries |
🡹 value = more transferable |
Sector specific context (digital health sector) |
||
Legislation exists to protect the privacy of personally identifiable data of individuals, irrespective of whether it is in paper or digital format |
mHealth apps work in settings where users feel their personal data is safe. Therefore, LFYM is more likely to be successful in countries in countries with legislation to protect patient data. |
“ 36 123 ” = more transferable |
eHealth composite index of adoption amongst GPs** |
LFYM users can share results from the app with their GP, therefore, LFYM is more likely to be successful in countries where GPs are comfortable using eHealth technologies |
🡹 value = more transferable |
Entity providing incentives and guidance for mHealth apps |
LFYM is more likely to be successful in countries where there is an entity responsible for promoting mHealth apps |
“ 36 123 ” = more transferable |
Political context |
||
A national eHealth policy or strategy exists |
LFYM is more likely to be successful if the government is supportive of eHealth |
“ 36 123 ” = more transferable |
Operational strategy/action plan/policy to reduce unhealthy eating |
LFYM will be more successful in countries which prioritise unhealthy eating |
“ 36 123 ” = more transferable |
Economic context |
||
Gross national income per capita (purchasing power parity, international dollars) |
Users have to pay out-of-pocket for LFYM, therefore, LFYM is more likely to be successful in more wealthy countries |
🡹 value = more transferable |
* The ICT development index represents a country’s information and communication technology capability. It is a composite indicator reflecting ICT readiness, intensity and impact (ITU, 2020[42]). **The eHealth composite index of adoption amongst GPs is made up of adoption in regards to electronic health records, telehealth, personal health records and health information exchange (European Commission, 2018[43]). Source: ITU (2020[42]), “The ICT Development Index (IDI): conceptual framework and methodology”, https://www.itu.int/en/ITU-D/Statistics/Pages/publications/mis/methodology.aspx; WHO (2015[44]), “Atlas of eHealth country profiles: The use of eHealth in support of universal health coverage”, https://www.afro.who.int/publications/atlas-ehealth-country-profiles-use‑ehealth-support-universal-health-coverage; WHO (n.d.[45]), “Global Health Observatory”, https://www.who.int/data/gho; World Bank (2017[46]), “GNI per capita, PPP (constant 2017 international $)”, https://data.worldbank.org/indicator/NY.GNP.PCAP.PP.KD; European Commission (2018[43]), “Benchmarking Deployment of eHealth among General Practitioners (2018)”, https://op.europa.eu/en/publication-detail/-/publication/d1286ce7‑5c05‑11e9‑9c52‑01aa75ed71a1.
Results
LFYM is broadly transferable across OECD and non-OECD European countries based on publically available data (Table 14.5). Compared to Romania (the owner setting), most countries have higher internet use and access as measured by the proportion of people accessing health information online, eHealth adoption amongst GPs and the ICT development index – for example, 33% of people in Romania seek health information online compared to an average of 54% in other countries. Further, most countries have legislation to protect digital patient data, as well as eHealth and unhealthy eating plans indicating political support for the intervention (64% and 90%, respectively).
Table 14.5. Transferability assessment by country, LFYM (OECD and non-OECD European countries)
A darker shade indicates Let Food Be Your Medicine may be more suitable for transferral in that particular country
% individuals seeking health information online |
ICT Development Index value |
Legislation to protect digital patient data |
eHealth adoption amongst GPs |
Entity promoting mHealth apps |
National eHealth policy |
Unhealthy eating action plan |
GNI per capita (PPP Int $) |
|
---|---|---|---|---|---|---|---|---|
Romania |
33 |
5.9 |
36 123 |
1.65 |
1.65 |
36 123 |
36 123 |
29 549 |
Australia |
42 |
8.2 |
2.05 |
2.05 |
2.05 |
36 123 |
36 123 |
48 007 |
Austria |
53 |
Yes |
36 123 |
1.91 |
1.65 |
1.65 |
36 123 |
56 304 |
Belgium |
49 |
1.65 |
36 123 |
2.07 |
1.65 |
36 123 |
36 123 |
52 562 |
Bulgaria |
34 |
6.4 |
36 123 |
1.81 |
1.65 |
36 123 |
36 123 |
22 883 |
Canada |
59 |
7.6 |
36 123 |
2.05 |
2.05 |
36 123 |
36 123 |
48 384 |
Chile |
27 |
6.1 |
36 123 |
2.05 |
2.05 |
36 123 |
36 123 |
24 131 |
Colombia |
41 |
5 |
36 123 |
2.05 |
2.05 |
2.05 |
36 123 |
14 163 |
Costa Rica |
44 |
6 |
36 123 |
2.05 |
2.05 |
36 123 |
36 123 |
19 094 |
Croatia |
53 |
6.8 |
36 123 |
2.18 |
36 123 |
36 123 |
36 123 |
28 388 |
Cyprus |
58 |
6.3 |
2.05 |
1.93 |
1.65 |
36 123 |
1.65 |
38 207 |
Czech Republic |
56 |
7.2 |
36 123 |
2.06 |
36 123 |
1.65 |
36 123 |
38 326 |
Denmark |
67 |
8.8 |
36 123 |
2.86 |
1.65 |
36 123 |
36 123 |
57 449 |
Estonia |
60 |
8 |
36 123 |
2.79 |
36 123 |
36 123 |
36 123 |
36 123 |
76 |
76 |
47 065 |
36 123 |
2.05 |
2.05 |
36 123 |
36 123 |
|
50 |
50 |
8 |
2.05 |
2.05 |
2.05 |
2.05 |
36 123 |
47 065 |
66 |
66 |
47 065 |
2.05 |
1.65 |
2.05 |
2.05 |
36 123 |
|
50 |
50 |
1.65 |
36 123 |
1.65 |
1.65 |
36 123 |
1.65 |
|
60 |
60 |
1.65 |
36 123 |
1.65 |
1.65 |
1.65 |
36 123 |
|
65 |
65 |
1.65 |
36 123 |
2.05 |
1.65 |
36 123 |
36 123 |
|
57 |
57 |
1.65 |
36 123 |
#N/A |
2.05 |
36 123 |
36 123 |
|
50 |
50 |
1.65 |
36 123 |
2.05 |
#N/A |
1.65 |
36 123 |
|
35 |
35 |
1.65 |
36 123 |
Yes |
1.65 |
36 123 |
36 123 |
|
Yes |
2.05 |
1.65 |
36 123 |
2.05 |
2.05 |
36 123 |
36 123 |
|
48 |
48 |
1.65 |
36 123 |
Yes |
1.65 |
36 123 |
36 123 |
|
61 |
61 |
7 |
36 123 |
1.65 |
36 123 |
36 123 |
36 123 |
35 989 |
58 |
58 |
1.65 |
36 123 |
Yes |
1.65 |
36 123 |
36 123 |
|
59 |
59 |
Yes |
36 123 |
2.05 |
36 123 |
1.65 |
36 123 |
|
50 |
50 |
Yes |
36 123 |
2.05 |
2.05 |
2.05 |
36 123 |
|
74 |
74 |
Yes |
36 123 |
2.05 |
36 123 |
36 123 |
36 123 |
|
n/a |
2.05 |
47 065 |
36 123 |
2.05 |
#N/A |
36 123 |
1.65 |
41 672 |
Norway |
69 |
Yes |
36 123 |
2.05 |
1.65 |
36 123 |
36 123 |
67 563 |
Poland |
47 |
1.65 |
36 123 |
1.84 |
1.65 |
36 123 |
36 123 |
31 913 |
Portugal |
49 |
1.65 |
36 123 |
2.12 |
36 123 |
1.65 |
36 123 |
34 154 |
Republic of Korea |
50 |
8.8 |
2.05 |
2.05 |
2.05 |
2.05 |
36 123 |
43 240 |
Slovak Republic |
53 |
6.7 |
2.05 |
1.76 |
2.05 |
2.05 |
36 123 |
29 622 |
Slovenia |
48 |
7.1 |
36 123 |
2.00 |
1.65 |
1.65 |
36 123 |
38 411 |
Spain |
60 |
Yes |
36 123 |
2.37 |
36 123 |
1.65 |
36 123 |
41 046 |
Sweden |
62 |
8.5 |
36 123 |
2.52 |
36 123 |
36 123 |
1.65 |
53 928 |
Switzerland |
67 |
8.5 |
36 123 |
2.05 |
1.65 |
36 123 |
36 123 |
65 821 |
Turkey |
51 |
5.5 |
36 123 |
2.05 |
36 123 |
1.65 |
36 123 |
27 814 |
United Kingdom |
67 |
8.5 |
36 123 |
2.52 |
36 123 |
36 123 |
36 123 |
45 851 |
Note: The shades of blue represent the distance each country is from the country in which the intervention currently operates, with a darker shade indicating greater transfer potential based on that particular indicator (see Annex A for further methodological details). n/a = data not available; GNI = gross national income; PPP = purchasing power parity.
Source: ITU (2020[42]), “The ICT Development Index (IDI): conceptual framework and methodology”, https://www.itu.int/en/ITU-D/Statistics/Pages/publications/mis/methodology.aspx; WHO (2015[44]), “Atlas of eHealth country profiles: The use of eHealth in support of universal health coverage”, https://www.afro.who.int/publications/atlas-ehealth-country-profiles-use‑ehealth-support-universal-health-coverage; WHO (n.d.[45]), “Global Health Observatory”, https://www.who.int/data/gho; World Bank (2017[46]), “GNI per capita, PPP (constant 2017 international $)”, https://data.worldbank.org/indicator/NY.GNP.PCAP.PP.KD; European Commission (2018[43]), “Benchmarking Deployment of eHealth among General Practitioners (2018)”, https://op.europa.eu/en/publication-detail/-/publication/d1286ce7‑5c05‑11e9‑9c52‑01aa75ed71a1.
To help consolidate findings from the transferability assessment above, countries have been clustered into one of four groups, based on indicators reported in Table 14.4. Countries in clusters with more positive values have the greatest transfer potential. For further details on the methodological approach used, please refer to Annex A.
Key findings from each of the clusters are below with further details in Figure 14.2 and Table 14.6:
Countries in cluster one have population, political, economic and sector specific arrangements in place to transfer LFYM. Countries in this cluster are therefore less likely to experience issues associated with implementing and operating LFYM in their local context.
Countries in cluster two have population, political and economic arrangements in place to transfer LFYM, but may wish to introduce policies that ensure the sector is ready for LFYM.
Countries in cluster three have a sector that supports LFYM. However, before transferring LFYM, countries in this cluster may want to consider increasing uptake of digital health technologies among the population, ensure LFYM aligns with political priorities and undertake further analysis to assess if LFYM is affordable among the population.
Countries in cluster four would likely support LFYM as it aligns with overarching political priorities. However, before transferring LFYM, countries in this cluster may also want to increase uptake of digital technologies, implement policies to ensure the sector is ready for this type of intervention, and assess overall affordability. It is important to note that Romania, which currently operates LFYM, is in this cluster, indicating although ideal, such conditions are not necessarily pre‑requisites for transferring LFYM.
Figure 14.2. Transferability assessment using clustering, LFYM
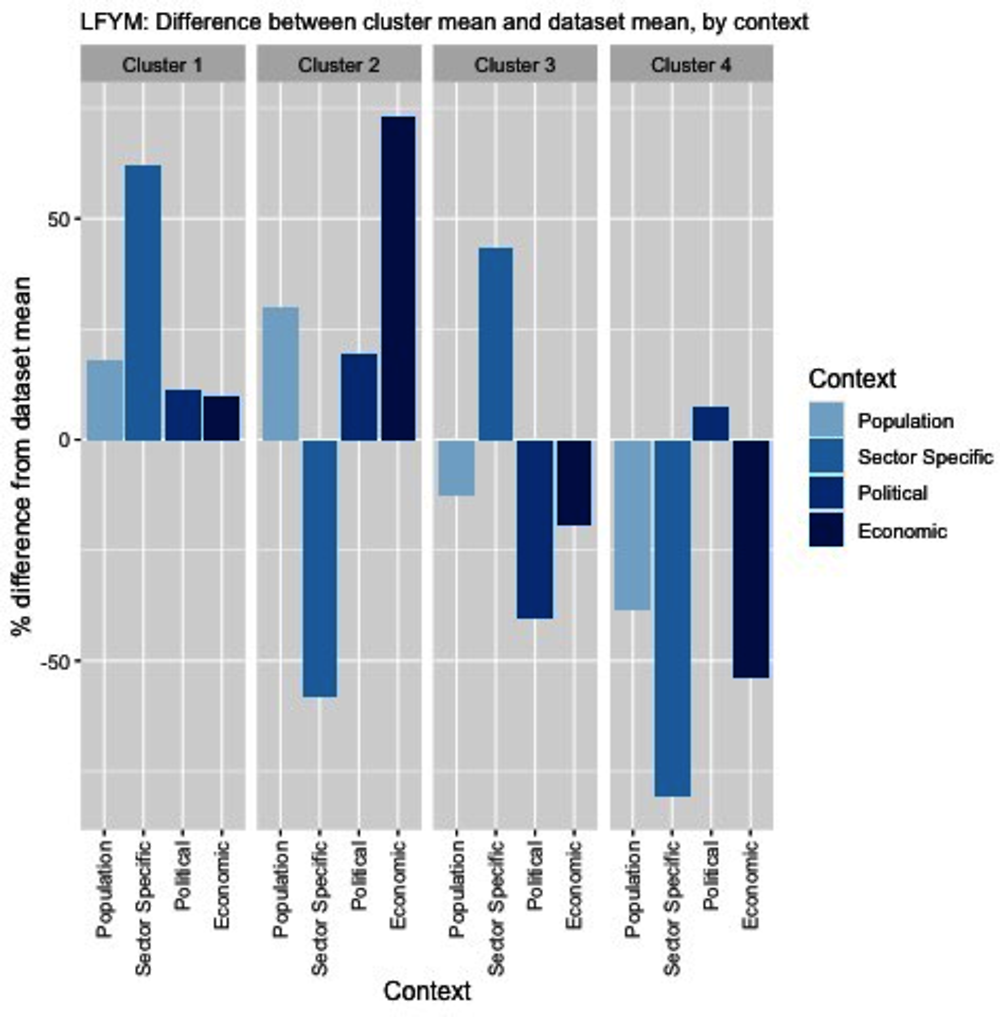
Note: Bar charts show percentage difference between cluster mean and dataset mean, for each indicator.
Source: ITU (2020[42]), “The ICT Development Index (IDI): conceptual framework and methodology”, https://www.itu.int/en/ITU-D/Statistics/Pages/publications/mis/methodology.aspx; WHO (2015[44]), “Atlas of eHealth country profiles: The use of eHealth in support of universal health coverage”, https://www.afro.who.int/publications/atlas-ehealth-country-profiles-use‑ehealth-support-universal-health-coverage; WHO (n.d.[45]), “Global Health Observatory”, https://www.who.int/data/gho; World Bank (2017[46]), “GNI per capita, PPP (constant 2017 international $)”, https://data.worldbank.org/indicator/NY.GNP.PCAP.PP.KD; European Commission (2018[43]), “Benchmarking Deployment of eHealth among General Practitioners (2018)”, https://op.europa.eu/en/publication-detail/-/publication/d1286ce7‑5c05‑11e9‑9c52‑01aa75ed71a1.
Table 14.6. Countries by cluster, LFYM
Cluster 1 |
Cluster 2 |
Cluster 3 |
Cluster 4 |
---|---|---|---|
Australia Belgium Canada Croatia Estonia Finland France Italy Japan Lithuania Netherlands New Zealand Republic of Korea Sweden United Kingdom |
Denmark Germany Iceland Ireland Luxembourg 1.65rway Switzerland United States |
Austria Czech Republic Hungary Israel Malta Mexico Portugal Slovenia Spain Turkey |
Bulgaria Chile Colombia Costa Rica Cyprus Greece Latvia Poland Romania Slovak Republic |
New indicators to assess transferability
Data from publically available datasets is not ideal to assess the transferability of LFYM, for example, there is no publically available data set on whether a country has regulations in place that forbid direct-to-consumer genetic testing. Therefore, Box 14.5 outlines several new indicators policy makers should consider before transferring LFYM.
Box 14.5. New indicators to assess transferability
In addition to the indicators within the transferability assessment, policy makers are encouraged to collect data for the following indicators:
Population context
How acceptable are digital personalised health interventions? (e.g. are they an appropriate response high obesity rates? Does the public understand the intervention and its purpose?)
What is the population’s willingness to share medical data with a private company?
Does the population trust their personal health information will be used, stored and managed appropriately?
What proportion of the population has access to a smartphone?
Sector specific context (digital health sector)
What, if any, compatible interventions exist?
What, if any, competing interventions exist? (e.g. other nutrition health apps)
How acceptable are apps and/or PN to improve diets amongst the health care profession?
What are the regulations regarding the use of genetic information?
Political context
Has the intervention received political support from key decision-makers?
Has the intervention received commitment from key decision-makers?
Economic context
What is the cost of implementing the intervention in the target context? (e.g. how do infrastructure costs differ between the primary and target context?)
Conclusion and next steps
LFYM is a promising mobile and web-based PN intervention with the potential to prevent as well as treat existing health care conditions. Given the intervention is currently in its infancy, data to evaluate the intervention against the OECD Framework was not available. Instead, this case study reviewed similar digital PN interventions which revealed many meet best practice criteria, for example, a recent systematic review found over 70% of studies conclude PN is cost-effective (Milanne Galekop, 2019[19]). However, similar to other digital health interventions, LFYM has the potential to widen social inequalities given mHealth apps are less accessible to certain priority population groups (e.g. lower SES).
A review of the literature highlighted several high-level policy options LFYM administrators could consider to enhance effectiveness, the evidence‑based, equity and extent of coverage. These include, but are not limited to, offering users targeted social support and motivational messages; additional promotion of the service to disadvantaged groups such as those with a lower socio‑economic status; as well as boosting public acceptability by ensuring transparency in regard to how data, genetic data in particular, is used and stored.
A transferability assessment comparing Romania, where LFYM is being piloted, and OECD and non-OECD European countries indicates the intervention is broadly transferable. For example, compared to Romania, most countries have higher levels of internet use and access among the public and health profession. However, before determining transferability, it is important to collect new types of information including regulation regarding the collection and use of genetic information.
Box 14.6 outlines next steps for policy makers and funding agencies regarding the LFYM intervention.
Box 14.6. Next steps for policy makers and funding agencies
Next steps for policy makers and funding agencies to enhance LFYM are listed below:
Support policies to enhance the use of mHealth apps such as boosting digital health literacy and ensuring health professionals have access to the necessary digital infrastructure at work
Support policies to improve access to mHealth apps by disadvantaged groups such as people living in regional/rural areas and elderly population
Closely follow LFYM developments in particular evaluation findings which can be used to develop subsequent policies (e.g. funding to scale‑up or transfer the intervention)
Promote findings from the LFYM case study to better understand what countries/regions are interested in transferring the intervention.
References
[4] Adams, S. et al. (2019), “Perspective: Guiding Principles for the Implementation of Personalized Nutrition Approaches That Benefit Health and Function”, Advances in Nutrition, https://doi.org/10.1093/advances/nmz086.
[15] Ambeba, E. et al. (2015), “The use of mHealth to deliver tailored messages reduces reported energy and fat intake”, Journal of Cardiovascular Nursing, Vol. 30/1, pp. 35-43, https://doi.org/10.1097/JCN.0000000000000120.
[21] Azzopardi-Muscat, N. and K. Sørensen (2019), “Towards an equitable digital public health era: promoting equity through a health literacy perspective”, European journal of public health, Vol. 29/3, pp. 13-17, https://doi.org/10.1093/eurpub/ckz166.
[20] Bol, N., N. Helberger and J. Weert (2018), “Differences in mobile health app use: A source of new digital inequalities?”, The Information Society, Vol. 34/3, pp. 183-193, https://doi.org/10.1080/01972243.2018.1438550.
[29] Browne, S. et al. (2019), “Effectiveness of interventions aimed at improving dietary behaviours among people at higher risk of or with chronic non-communicable diseases: an overview of systematic reviews”, Eur J Clin Nutr, Vol. 73, pp. 9-23, https://doi.org/10.1038/s41430-018-0327-3.
[34] Celis-Morales, C., J. Lara and J. Mathers (2015), Personalising nutritional guidance for more effective behaviour change, Cambridge University Press, https://doi.org/10.1017/S0029665114001633.
[16] Celis-Morales, C. et al. (2017), “Effect of personalized nutrition on health-related behaviour change: evidence from the Food4Me European randomized controlled trial”, International Journal of Epidemiology, Vol. 46/2, https://doi.org/10.1093/ije/dyw186.
[40] Chen, J. et al. (2017), “The use of smartphone health apps and other mobile health (mHealth) technologies in dietetic practice: a three country study”, Journal of Human Nutrition and Dietetics, Vol. 30/4, pp. 439-452, https://doi.org/10.1111/jhn.12446.
[18] Dalziel, K. and L. Segal (2007), “Time to Give Nutrition Interventions a Higher Profile: Cost-Effectiveness of 10 Nutrition Interventions”, Health Promotion International, Vol. 22/4.
[9] Deloitte (2017), Global mobile consumer trends, 2nd edition Mobile continues its global reach into all aspects of consumers’ lives, https://www2.deloitte.com/content/dam/Deloitte/us/Documents/technology-media-telecommunications/us-global-mobile-consumer-survey-second-edition.pdf.
[25] Effective Public Health Practice Project (1998), Quality assessment tool for quantitative studies, https://www.nccmt.ca/knowledge-repositories/search/14.
[43] European Commission (2018), Benchmarking Deployment of eHealth among General Practitioners (2018), https://op.europa.eu/en/publication-detail/-/publication/d1286ce7-5c05-11e9-9c52-01aa75ed71a1.
[3] Fallaize, R. et al. (2013), “An insight into the public acceptance of nutrigenomic-based personalised nutrition”, Nutrition Research Reviews, Vol. 26/1, pp. 39-48, https://doi.org/10.1017/S0954422413000024.
[6] Forster, H. et al. (2014), “Online dietary intake estimation: The food4me food frequency questionnaire”, Journal of Medical Internet Research, Vol. 16/6, https://doi.org/10.2196/jmir.3105.
[5] Forster, H. et al. (2016), Personalised nutrition: The role of new dietary assessment methods, Cambridge University Press, https://doi.org/10.1017/S0029665115002086.
[26] Goryakin, Y. et al. (2017), The Role of Communication in Public Health Policies - The case of obesity prevention in Italy, OECD, Paris, http://www.salute.gov.it/imgs/C_17_pubblicazioni_2647_allegato.pdf.
[28] Hwang, K. et al. (2010), “Social support in an Internet weight loss community”, International Journal of Medical Informatics, Vol. 79/1, pp. 5-13, https://doi.org/10.1016/j.ijmedinf.2009.10.003.
[42] ITU (2020), The ICT Development Index (IDI): conceptual framework and methodology, https://www.itu.int/en/ITU-D/Statistics/Pages/publications/mis/methodology.aspx (accessed on 26 February 2021).
[31] Jane, M. et al. (2018), “Social media for health promotion and weight management: A critical debate”, BMC Public Health, Vol. 18/1, https://doi.org/10.1186/s12889-018-5837-3.
[33] Janssen, V. et al. (2013), “Lifestyle modification programmes for patients with coronary heart disease: a systematic review and meta-analysis of randomized controlled trials”, European Journal of Preventive Cardiology, Vol. 20/4, pp. 620-640, https://doi.org/10.1177/2047487312462824.
[23] Jarke, H. (2018), “mHealth – Friend or Foe in Reducing Inequality? A Systematic Literature Review”, https://doi.org/10.31237/OSF.IO/W8QGJ.
[11] Kroeze, W., A. Werkman and J. Brug (2006), “A systematic review of randomized trials on the effectiveness of computer-tailored education on physical activity and dietary behaviors”, Annals of Behavioral Medicine, Vol. 31/3, pp. 205-223, https://doi.org/10.1207/s15324796abm3103_2.
[17] Laddu, D. and M. Hauser (2019), Addressing the Nutritional Phenotype Through Personalized Nutrition for Chronic Disease Prevention and Management, W.B. Saunders, https://doi.org/10.1016/j.pcad.2018.12.004.
[27] Livingstone, K. et al. (2017), “Characteristics of European adults who dropped out from the Food4Me Internet-based personalised nutrition intervention”, Public Health Nutrition, Vol. 20/1, pp. 53-63, https://doi.org/10.1017/S1368980016002020.
[14] Maes, L. et al. (2011), “Pilot evaluation of the HELENA (Healthy Lifestyle in Europe by Nutrition in Adolescence) Food-O-Meter, a computer-tailored nutrition advice for adolescents: A study in six European cities”, Public Health Nutrition, Vol. 14/7, pp. 1292-1302, https://doi.org/10.1017/S1368980010003563.
[2] Marsaux, C. et al. (2015), “Effects of a Web-Based Personalized Intervention on Physical Activity in European Adults: A Randomized Controlled Trial.”, Journal of medical Internet research, Vol. 17/10, p. e231, https://doi.org/10.2196/jmir.4660.
[19] Milanne Galekop (2019), A systematic review of the cost-effectiveness of personalized interventions with a nutritional component, Erasmus School of Health Policy and Management, https://preventomics.eu/wp-content/uploads/2020/02/Milanne-Galekop_ISPOR_2019_2.pdf.
[35] Moreira, L. (2018), “Health literacy for people-centred care: Where do OECD countries stand?”, OECD Health Working Papers, No. 107, OECD Publishing, Paris, https://doi.org/10.1787/d8494d3a-en.
[8] NutriCare (2019), Let Food Be Your Medicine, https://www.nutricare.life/.
[7] Nutricare (2020), Improve your Destiny!, https://www.nutricare.life/ (accessed on 9 June 2020).
[36] OECD (2022), Guidebook on Best Practices in Public Health, OECD Publishing, Paris, https://doi.org/10.1787/4f4913dd-en.
[39] OECD (2020), OECD Reviews of Public Health: Korea: A Healthier Tomorrow, OECD Reviews of Public Health, OECD Publishing, Paris, https://doi.org/10.1787/be2b7063-en.
[24] OECD (2019), Health in the 21st Century: Putting Data to Work for Stronger Health Systems, OECD Health Policy Studies, OECD Publishing, Paris, https://doi.org/10.1787/e3b23f8e-en.
[10] OECD (2019), The Heavy Burden of Obesity: The Economics of Prevention, OECD Health Policy Studies, OECD Publishing, Paris, https://doi.org/10.1787/67450d67-en.
[22] Oliveira Hashiguchi, T. (2020), “Bringing health care to the patient: An overview of the use of telemedicine in OECD countries”, OECD Health Working Papers, No. 116, OECD Publishing, Paris, https://doi.org/10.1787/8e56ede7-en.
[1] Ordovas, J. et al. (2018), “Personalised nutrition and health”, BMJ (Online), Vol. 361, https://doi.org/10.1136/bmj.k2173.
[30] Schulz, D. et al. (2014), “Economic evaluation of a web-based tailored lifestyle intervention for adults: Findings regarding cost-effectiveness and cost-utility from a randomized controlled trial”, Journal of Medical Internet Research, Vol. 16/3, https://doi.org/10.2196/jmir.3159.
[32] Sharpe, E., E. Karasouli and C. Meyer (2017), “Examining Factors of Engagement With Digital Interventions for Weight Management: Rapid Review”, JMIR Research Protocols, Vol. 6/10, p. e205, https://doi.org/10.2196/resprot.6059.
[13] Springvloet, L. et al. (2015), “Short-and medium-term efficacy of a web-based computer-tailored nutrition education intervention for adultsincluding cognitive and environmental feedback: Randomized controlled trial”, Journal of Medical Internet Research, Vol. 17/1, p. e23, https://doi.org/10.2196/jmir.3837.
[12] Sternfeld, B. et al. (2009), “Improving Diet and Physical Activity with ALIVE. A Worksite Randomized Trial”, American Journal of Preventive Medicine, Vol. 36/6, pp. 475-483, https://doi.org/10.1016/j.amepre.2009.01.036.
[38] Stewart-Knox, B. et al. (2009), “Attitudes toward genetic testing and personalised nutrition in a representative sample of European consumers”, British Journal of Nutrition, Vol. 101/7, pp. 982-989, https://doi.org/10.1017/S0007114508055657.
[41] WHO (2021), Addressing mobile health, https://www.who.int/activities/Addressing-mobile-health (accessed on 14 March 2021).
[44] WHO (2015), Atlas of eHealth country profiles: The use of eHealth in support of universal health coverage, Global Observatory for eHealth, https://www.afro.who.int/publications/atlas-ehealth-country-profiles-use-ehealth-support-universal-health-coverage.
[45] WHO (n.d.), Global Health Observatory, https://www.who.int/data/gho (accessed on 25 August 2021).
[37] WHO (n.d.), Interventions on diet and physical activity: what works - summary report, https://www.who.int/dietphysicalactivity/summary-report-09.pdf?ua=1.
[46] World Bank (2017), GNI per capita, PPP (constant 2017 international $).
Notes
← 1. A smart scale measures weight and other body composition metrics and automatically records and uploads this information to an app or other online service.
← 2. The dietary assessment covers: Carbohydrates, Fibre, Protein, Omega 3, Omega 6, Calcium, Chloride, Copper, Iron, Iodine, Potassium, Magnesium, Manganese, Sodium, Phosphorus, Selenium, Zinc, Vitamin A, Vitamin B1, Vitamin B2, Vitamin B3, Vitamin B5, Vitamin B6, Vitamin B7, Folate, Vitamin B12, Vitamin C, Vitamin D, Vitamin E, and Vitamin K.
← 3. Phenotypic data includes information such as age, sex, ethnicity and disease profile,
← 4. For contextual purposes, the National Institute of Heath and Care Excellence (NICE) applies a cost per QALY threshold of between GBP 20 000‑30 000 (EUR 24 010‑36 016) when developing advice on which health interventions to fund or not.
← 5. Phenotypic data includes information such as age, sex, ethnicity and disease profile,
← 6. A forum allowing users to interact with one another is planned for Q1 of 2021.
← 7. Intermediate outcomes that are known to relate directly to the final outcome – for example HbA1c levels to diabetes prevalence, cholesterol levels to heart attacks, or calorie intake to obesity prevalence.