Artificial intelligence and jobs: An urgent need to act
OECD Employment Outlook: Artificial Intelligence and the Labour Market
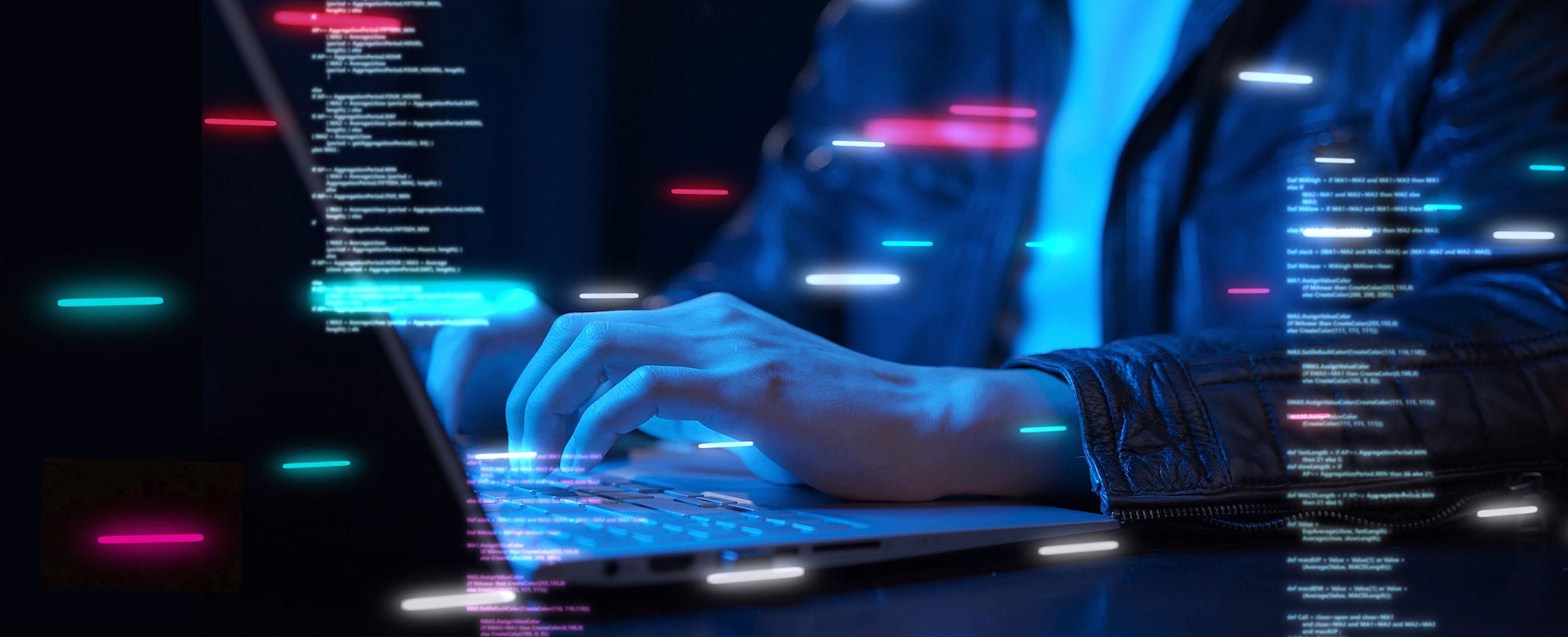
4. Artificial intelligence, job quality and inclusiveness
Abstract
For many workers, the effects of artificial intelligence (AI) will be visible not in terms of lost employment but through changes in the tasks they perform at work and changes in job quality. This chapter reviews the current empirical evidence of the effect of AI on job quality and inclusiveness. For workers with the skills to complement AI, task changes should be accompanied by rising wages, but wages could decline for workers who find themselves squeezed into a diminished share of tasks due to automation. AI may affect job quality through other mechanisms as well. For example, it can reduce tedious or dangerous tasks, but it may also leave workers with a higher-paced work environment. The chapter further shows that using AI to support managers’ tasks affects the job quality of their subordinates. Finally, the chapter shows that using AI affects workplace inclusiveness and fairness, with implications for job quality.
In Brief
Like other automation technologies before it, artificial intelligence (AI) may make some jobs redundant, forcing some workers to find a new job or update their skills (Chapter 3). However, according to workers’ own accounts, so far, the greatest impact of AI has been felt mostly through changes in the tasks they perform in their current roles as well as changes in the working environment. These changes can have a direct impact on the quality of people’s jobs and therefore on their well-being. For example, AI can automate dangerous tasks, which should improve job satisfaction and safety in certain hazardous jobs. On the other hand, workers may find themselves squeezed into a shrinking set of simpler tasks, which could put downward pressure on their wages.
AI also presents other challenges and opportunities for job quality and inclusiveness. For example, when used to support or automate managerial tasks – such as monitoring workers, allocating tasks, or deciding who should receive training or promotion (“algorithmic management”) – AI systems can lead to more data-driven and consistent management and assessment of workers. If not designed and implemented well, however, AI systems can reinforce pre‑existing biases, harm privacy, increase work intensity and reduce autonomy in executing tasks.
This chapter reviews the current empirical evidence of the effect of AI on job quality and inclusiveness, also drawing on recent OECD AI surveys of employers and workers and case studies of AI use in the workplace. While these studies provide unprecedented detail on firms’ current AI implementation and workers’ perceptions of AI, it is important to emphasise that the findings are limited to the manufacturing and finance sectors and that they only capture the perceptions of workers who are employed after AI adoption. A number of key findings emerge:
Workers with AI-specific skills – i.e. workers who develop, train or maintain AI systems – earn high wages and enjoy substantial wage premiums, even compared to observably similar workers with other advanced skills (e.g. software, cognitive, etc.). The highest AI wage premiums are found in management occupations, suggesting substantial demand for workers who know how AI can fit into the broader production process.
For the larger set of workers exposed to AI – i.e. workers who use or interact with AI but do not necessarily have or require special AI skills – the use of AI has had only a minimal impact on wages so far.
These minimal impacts on wages are consistent with empirical findings that suggest that, so far, AI has only had a modest impact on productivity. AI is currently more likely to be adopted by larger and more capital-intensive firms (which tend to be more productive), but productivity gains are small after accounting for observable differences between firms. This said, more recent case study evidence examining specific applications of generative AI finds larger effects on productivity.
The OECD AI surveys find that the majority of workers report higher enjoyment in their jobs and improved mental and physical health due to AI. In case studies of AI use, workers frequently report AI’s capability to improve the safe use of machines as one reason for improved physical safety.
A potential mechanism for AI’s impact on job quality is that the task composition of jobs changes. To date, AI appears to be automating more tedious, repetitive tasks than it creates, while also broadening the range of tasks performed by workers and aiding them in their decision making.
Most workers in the OECD AI surveys said that AI improves autonomy, defined as control over the sequence in which they perform their tasks. This depends, however, on how AI is implemented and used. One in five respondents reported that AI decreased their autonomy, and this share is larger among the relatively small group who report to be subject to algorithmic management.
The advanced monitoring and feedback made possible by using AI for managerial tasks can be more pervasive than human monitoring and feedback. In the OECD AI surveys, workers report higher work intensity after the adoption of AI and, in some cases, less human-to-human interaction. The surveys also show that, when employers’ use of AI involves data collection on workers or how they do their job, the majority of workers are worried about their privacy.
The impact of AI on job satisfaction and health differs across groups of workers, affecting inclusiveness in the workplace. Workers in managerial roles, those with the skills to develop and maintain AI systems, and workers with tertiary degrees tend to report higher job satisfaction and improved health after AI adoption. Workers who are subject to algorithmic management or those who work with AI report instead the least positive outcomes of using AI on their job quality. If implemented well, AI can also increase accessibility and job satisfaction for workers traditionally disadvantaged in the labour market, such as workers with disabilities.
Close to half of workers who use AI in the OECD AI surveys believe that AI has improved how fairly they are being treated by their manager. However, AI systems can struggle with bias, both at the data level and at the system level. While bias is widespread in human decisions as well, the use of biased AI systems carries the risk of multiplying and systematising biases.
AI’s capabilities are progressing faster than the studies measuring its effects. New work will be needed in the future to see if the results of this chapter also hold for newer AI applications such as large language models, and across a larger set of sectors.
Introduction
For most workers, the effect of artificial intelligence (AI) is likely to be felt through changes to the tasks they perform in their current jobs and through changes in the working environment, rather than through lost employment. This could happen because AI changes task content – for example, by displacing a manufacturing worker from the visual inspection and quality control of a product, leaving her with a diminished set of elementary tasks. AI may also alter jobs without fundamentally changing their task content. For instance, the job of food delivery has remained fundamentally unchanged over the past few decades. However, where 30 years ago one might have taken direction from a manager, now an algorithm dictates where one must deliver, how to get there, and even determines if the worker keeps their job on the delivery platform based on anonymous customer reviews. How AI changes tasks and more generally shapes the work environment has important implications for the quality of people’s jobs and ultimately their well-being.
The OECD job quality framework sets out a comprehensive structure for measuring and assessing job quality, focusing on those aspects of a job that have been shown to be particularly important for workers’ well-being (OECD, 2014[1]). These include things such as the level and distribution of earnings, employment security and the quality of the working environment. The latter includes the cumulative effect of job demands such as work intensity and performing physically demanding tasks. It also includes job resources that help workers cope with difficult demands such as worker autonomy to change the order of tasks or the method of work.
A core question addressed in this chapter is to what extent AI leads to wage changes (Section 4.1). AI has the potential to change the task profile of jobs, automating some tasks, complementing others and introducing new tasks (see Chapter 3). These tasks changes should result in cost savings for firms and higher productivity (Acemoglu and Restrepo, 2018[2]). However, whether the expected productivity increases from AI lead to wage increases or decreases for workers is theoretically ambiguous.
Beyond earnings changes, this chapter is also concerned with how AI changes the demands and resources for workers, and what that means for job satisfaction (Section 4.2). An AI-powered chatbot, for example, may automate customer service representatives’ easy or mundane calls, leaving them with only the more complex and involved customer queries. This could result in higher job quality as the representative takes on a higher share of more complex calls which require greater human interaction leading to more rewarding work. On the other hand, the disappearance of the routine calls may deprive the worker of the respite provided by these calls, leading to more mentally taxing shifts. Whether the sum of the new demands and resources brought by AI improves job satisfaction is the other side of job quality explored in this chapter.
AI also brings a new set of issues to the table when it comes to job quality. For example, AI can support or automate supervisory functions previously performed by humans, including giving direction, monitoring and evaluation (“algorithmic management”). This means that some workers may see little to no changes in the tasks they perform, but their manager is supported or replaced by an algorithm, which could, for instance, lead to reductions in privacy and autonomy, and increases in work intensity and stress (Section 4.3).
This chapter also reviews the potential of AI to improve labour market inclusiveness. Inclusive workplaces not only improve access to higher quality jobs for disadvantaged workers, but other workers also stand to benefit because workers value workplaces they perceive as fair to their co-workers as well as to themselves (Dube, Giuliano and Leonard, 2019[3]; Heinz et al., 2020[4]). By promoting more objective performance evaluations, for example, AI can increase fairness for workers who have traditionally suffered from bias in the labour market with the additional benefit of improving the job quality of their peers by improving the perceived fairness of their employer. However, if not designed and implemented well, AI can have negative effects on inclusiveness and fairness in the workplace. Through its reliance on data trained on past human experience, for example, AI risks introducing or reinforcing systematic bias into a range of labour market decisions if not implemented with care (Section 4.4).
This chapter reviews the empirical literature on the effect of AI on job quality and inclusiveness. This is a nascent, but active, area of research and new work will be needed in the future to see if the results of this chapter hold with the adoption of new AI applications such as large language models (ChatGPT, for example). The chapter begins by reviewing the empirical literature on the effect of AI on wages and productivity (Section 4.1). The chapter then elaborates on the effect of AI on job quality more generally including on job demands and resources (Section 4.2). Section 4.3 discusses the impact of algorithmic management on job quality and Section 4.4 explains how AI can increase fairness, but also the potential bias AI may inject into the labour market. The chapter concludes by offering some policy suggestions for promoting a positive impact of AI on job quality (Section 4.5).
4.1. Workers with AI skills earn significant wage premiums, but it is too soon to see AI’s effects on labour productivity
The accumulating evidence on wages for workers with AI skills finds that these workers earn substantial wage premiums. However, for the larger set of workers exposed to AI, the technology appears to have had minimal effects on wages so far. AI is currently more likely to be adopted by larger and more capital-intensive firms, but after adjusting for observable differences between firms, the literature currently finds only modest gains to productivity.
4.1.1. Some workers with the right skills have seen wage gains after AI adoption
The changing composition of tasks due to the adoption of AI brings theoretically ambiguous predictions for the direction of wage changes. AI changes tasks through two main channels. First, AI can create entirely new tasks for workers (see Chapter 3). These are often, but not exclusively, new jobs for workers with AI skills (see as well Chapter 5).1 When AI creates new tasks for workers, it should lead to rising wages for affected workers.
In addition, AI can automate a set of tasks within a firm or occupation, which leads to two competing effects producing ambiguous changes in wages. First, a productivity effect arises from the costs savings from AI. If these cost savings are sufficiently large, this productivity effect leads to greater demand for tasks that are not yet automated and pushes up wages for workers who have not seen their tasks automated by AI. However, automation also leads to a displacement effect that leads to lower wages. Intuitively, when tasks are automated, affected workers are bunched into a few sets of tasks putting downward pressure on wages.2
Workers with AI skills earn significant wage premiums
Workers with AI skills are a small, but rapidly growing share of the OECD employed population, and they earn relatively high wages. Green and Lamby (2023[5]) use Lightcast job posting data combined with labour force surveys to measure the size and characteristics of the AI workforce – defined as workers with a combination of skills in statistics, computer science and machine learning.3 They find that the share of the employed population with AI skills is small – at most 0.3% of those employed in OECD countries, on average – but growing rapidly. In EU countries, they show that nearly half the AI workforce has labour earnings in the top two deciles of the labour earnings distribution, which is higher than for the employed population with a tertiary degree in these countries.
The available evidence for workers with AI skills in the United States suggests that they receive a significant wage premium. Alekseeva et al. (2021[6]) use Lightcast data of online job postings which include offered wages and skills demanded to measure wage premiums of AI skills. After controlling for skills demanded and the local labour market, they find a wage premium of 11% for job postings that require AI skills within the same firm, and 5% within the same firm and job title. The wage premium is higher than the premiums associated with other skills commonly demanded in high-paying occupations (e.g. software, cognitive and management skills). The highest wage premium for AI skills is found in management occupations, suggesting that employers value skillsets that signal understanding of how to deploy AI in the broader production process (see Chapter 3).
Significant wage premiums for AI skills are also found in a wider set of Anglophone countries. Manca (2023[7]) also uses Lightcast data but from a larger set of countries including the United Kingdom, Canada, Australia, New Zealand in addition to the United States. The analysis finds that job postings demanding skills closely related to AI skills (Machine Learning, for example) pay significantly higher wages on average in the five Anglophone countries. The analysis further shows that occupations with skill bundles most similar to skills associated with AI offer wage premiums that range from 4% in Australia and New Zealand to over 10% in the United States.
Surveys of workers with AI skills find that they are similarly optimistic about the future trajectory of wages. The OECD AI surveys of employers and workers (hereafter: “OECD AI surveys”, see Box 4.1) find that, among workers who actively develop or maintain AI systems, 47% in manufacturing and 50% in finance expected that their wages would increase after the implementation of AI. This contrasts with 18% and 29% in finance and manufacturing, respectively, who expected that wages would decrease (Lane, Williams and Broecke, 2023[8]).
Box 4.1. The OECD surveys of AI use, and case studies of AI
While there is a growing body of research on the impact of AI on job quality, there has been little analysis to date examining what happens in organisations and to workers when AI is introduced. To fill this void, the OECD conducted surveys and case study interviews of workers and firms that have adopted AI in the workplace. Both the case studies and firm surveys focused on two sectors to better understand the specific, AI-related technologies used in those sectors. The two chosen sectors, finance/insurance and manufacturing, offer a higher prevalence of AI use compared to other sectors, as well as heterogeneity between the two sectors in terms of worker profile.
The OECD AI surveys of employers and workers (OECD AI surveys, henceforth)
Wishing to capture workers’ and employers’ own perceptions of the current and future impact of AI on their workplaces, the OECD surveyed a total of 5 334 workers and 2 053 firms in the manufacturing and financial sectors in Austria, Canada, France, Germany, Ireland, the United Kingdom and the United States. The surveys examine how and why AI is being implemented in the workplace; its impact on management, working conditions and skill needs; its impact on worker productivity, wages and employment; what measures are being put in place to manage transitions; and concerns and attitudes surrounding AI. The most frequently reported uses of AI include data analytics and fraud detection in the finance sector, and production processes and maintenance tasks in manufacturing.
The OECD commissioned two surveys between mid-January and mid-February 2022. The polling firm conducted a telephone survey of employers by contacting management representatives of companies with 20 or more employees. The sampling frames for the employer survey were predominantly provided by Dun & Bradstreet, which claims to have the largest business databank worldwide. The employer survey was weighted by country and firm size. The worker survey was implemented as an online survey using access panels – databases of individuals who have indicated a willingness to participate in future online surveys for compensation. The worker survey was weighted by age, education, gender and country. One disadvantage of these panels, which may undermine representativeness, is that they exclude those without internet access.
The OECD case studies of AI implementation (OECD AI case studies, henceforth)
The OECD case studies examine the perceived impact of AI technologies on job quantity, skills needs and job quality at the firm level in the same two sectors as the surveys – i.e., finance/insurance and manufacturing – and in eight countries: Austria, Canada, France, Germany, Ireland, Japan, the United Kingdom and the United States. In each country, the OECD engaged a research team to recruit firms that had implemented AI technologies and to carry out semi-structured interviews with different stakeholders able to speak to the impact of the technology on workers.
The research teams sought interviews with a range of different stakeholders to capture a variety of perspectives. The stakeholders interviewed included workers affected by the AI technology, managers, human resource personnel, AI technology developers or suppliers, AI implementation leads, and worker representatives. A total of 90 firms participated in the project. In firm recruitment, the researchers were free to identify potentially suitable firms as they chose, including using existing personal or professional contacts/networks and/or making new contacts. The OECD supported the research teams by advertising the project and using its own network. Across the 90 firms, 325 interviews were held as part of the case studies: 147 in finance, 154 in manufacturing and 24 in the energy and logistics sectors.1
1. Due to difficulties encountered in firm recruitment, AI case studies researchers were encouraged to recruit a limited set of firms in the energy and logistics sector.
Source: Lane, Williams and Broecke (2023[8]), “The impact of AI on the workplace: Main findings from the OECD AI surveys of employers and workers”, https://doi.org/10.1787/ea0a0fe1-en; Milanez (2023[9]), “The impact of AI on the workplace: Evidence from OECD case studies of AI implementation”, https://doi.org/10.1787/2247ce58-en.
Workers exposed to AI have seen stable or increasing wages, but more research is needed
Some researchers find wage gains for the larger set of workers exposed to recent advances in AI, who do not necessarily have AI skills. Felten, Raj and Seamans (2021[10]) measure exposure to AI as progress in AI applications from the Electronic Frontier Foundation’s AI Progress Measurement project and connect it to abilities from the Occupational Information Network (O*NET)4 using crowd-sourced assessments of the connection between applications and abilities.5 They find that a one standard deviation increase in exposure to AI is associated with a 0.4 percentage point increase in wage growth. This is largely driven by occupations that require a high level of familiarity with software, but the research design does not separate workers with AI skills (Felten, Raj and Seamans, 2019[11]).6
Research using the same AI exposure measure, but different data sets from the United States, similarly finds that workers exposed to AI tend to earn more. Using data from the United States over the period 2011‑18, and exploiting short panels following workers over time, Fossen and Sorgner (2019[12]) find that the more workers are exposed to AI, the higher their wages. In their preferred specification (using the same exposure measure as above), a one standard deviation increase in AI exposure increases wages by over 4%. Similar positive results are obtained using different measures of AI exposure, but the effect is attenuated by workers who change jobs.7
In contrast, the OECD case studies of AI implementation (hereafter: “OECD AI case studies”, see Box 4.1) find that, so far, AI has led to little wage changes (Milanez, 2023[9]). Case study interviewees most often reported that the wages of workers most affected by AI technologies remained unchanged (84% of case studies). In fewer instances, interviewees reported wage increases (15% of case studies). Increases tended to be due to greater complexity of tasks or new skill acquisition following training or to increases in performance metrics that impact wages. Most commonly, wage increases were on account of greater complexity of tasks or new skill acquisition following training. Notably, instances of wage increases tended to occur in Austrian case studies, where collective bargaining over such matters can be strong (see Chapter 7 for the role of social partners in AI implementation).
Although AI does not currently appear to be putting downward pressure on wages, surveys of workers who use AI find that they are worried about future changes to wages. The OECD AI surveys found that, when asked what impact they expect of AI on wages in the next 10 years, 42% of workers surveyed in finance expected that AI would decrease wages. A further 23% expected that wages would remain the same and 16% expected wages to increase. In manufacturing, 41% of workers suggested that AI would decrease wages, followed by 25% suggesting that wages would remain the same and 13% reporting that wages would increase (Lane, Williams and Broecke, 2023[8]). Workers with a university degree and managers were among the most likely to say they expected their wages to increase which is consistent with much of the other empirical findings in this chapter. Additionally, men – particularly in finance – were more likely than women to expect a wage increase and less likely to expect a wage decrease due to AI. These results indicate that AI may put further pressure on currently existing wage inequalities.
4.1.2. Larger, more productive firms tend to adopt AI, but its effects on labour productivity are so far inconclusive
Wage changes induced by AI adoption ultimately stem from the productivity gains enjoyed by firms after AI implementation. The limited effect of AI on wages – particularly for workers exposed to AI – may reflect its limited impact on productivity so far. This section summarises the current empirical evidence for the effect of AI on labour productivity.
Larger, more productive firms are more likely to adopt AI
Larger, more productive firms are more likely to adopt AI. A representative sample of businesses from the United States in 2018 finds that the incidence of AI adoption rises with firm size and the average wage of the firm (Acemoglu et al., 2022[13]). Similarly, an OECD study on the United Kingdom in 2019 finds that AI adopters tend to be towards the top of their industry’s productivity distribution. Using information from websites, patents and job postings to identify AI adopters, the report finds that AI adoption increases with firm size (Calvino et al., 2022[14]).
Harmonised, cross-country survey evidence also finds that larger firms are more likely to adopt AI. Calvino and Fontanelli, (2023[15]) analyse official firm-level surveys of AI use across 11 OECD countries using a common statistical methodology and find that AI is more widely adopted by larger firms. The authors speculate that this may be because larger firms have more resources to allow the adoption of complementary assets to fully leverage AI (see also Chapter 3).
The positive relationship between firm size and AI adoption is also confirmed from studies using job vacancies in the United States. Alekseeva et al. (2021[6]) use Lightcast job vacancy data to measure firm-level hiring of workers with AI skills as a proxy for AI adoption. They find that AI adoption is positively associated with a firm’s level of sales, employment and market capitalisation. This finding is confirmed in the United States using a different database of job postings and CVs and again using sales to measure firm size (Babina et al., 2020[16]).
AI appears to be producing modest productivity increases, but the evidence is far from conclusive
Although larger, more productive firms are more likely to adopt AI, evidence for a positive causal relationship between AI and productivity is so far tenuous. Using the same employer survey from the U.S. Census Bureau, Acemoglu et al. (2022[13]) find that AI and dedicated equipment are not correlated with labour productivity. Using a regression model to adjust for observable differences in firms that adopt AI from those that do not, they find a modest positive, but not statistically significant, effect of AI adoption on productivity. The authors provide a few reasons for this finding, including that the effects of AI on labour productivity had not fully materialised at the time of the study (the data are from 2018), and/or that the various automation technologies are often adopted together, muddying any clear interpretation of the effect on AI productivity.
Using job postings and a database of CVs with job histories, Babina et al. (2020[16]) document a strong and consistent pattern of higher growth among U.S. firms that invest more in AI: a one‑standard-deviation increase in a resume‑based measure of AI investments over an 8‑year period corresponds to a 20.3% increase in sales, a 21.7% increase in employment, and a 22.4% increase in market valuation. However, AI investments are not associated with changes in sales per worker (a rough measure of labour productivity), total factor productivity, or process patents (i.e. patents focusing on process innovation). In other words, while sales and employment increase from AI, they increase proportionally, leading to no statistically significant changes in productivity.8
Evidence from a larger set of OECD countries similarly finds inconclusive effects of AI on productivity. Calvino and Fontanelli (2023[15]) find that productivity premia emerge for AI adopters, but only when adopted with complementary assets. Looking only at the United Kingdom, Calvino et al. (2022[14]) report that when focusing on larger firms (more than 249 employees), productivity premia emerge for AI adopters. However, when including all firms in the sample, the effect is no longer statistically significant. Furthermore, when focusing on the “intensive margin” of AI adoption, using the differential hiring rate of workers with AI skills, productivity premia again are present for firms with at least 250 employees. The authors also find that much of this productivity premium for firms hiring workers with AI skills comes from managers and high-skill professionals, which is consistent with evidence on wages (above) and job satisfaction (see Section 4.2), as well as employment and skills found in other chapters in this volume (Chapters 3 and 5, respectively).
When asked directly, however, employers do report that AI increases productivity. The OECD AI surveys asked what effect AI adoption had on productivity. Fifty-seven percent of employers in finance and 63% of employers in manufacturing said AI had a positive impact on worker productivity, compared to 8% and 5% who said it had a negative impact (Lane, Williams and Broecke, 2023[8]). The positive reported impact on worker productivity is consistent with emerging studies of adoption of generative AI applications in the workplace, although these applications tend to be quite specific in scope, and it is too soon to see how they generalise to a wider set of firms (see Box 4.2).
Workers who use AI also believe it has a positive impact on their performance. The same OECD AI surveys find that a majority of workers who interact with AI (either themselves, developing AI or managing others who use AI) report that their performance has improved after the introduction of AI. Across both sectors, over 80% of workers who work with AI report higher job performance. Workers with a university degree as well as workers in management and professional occupations report higher performance from AI than those without a university degree and workers in production and non-supervisory roles.9
Box 4.2. Emerging evidence shows that generative AI produces productivity gains and equalises performance between workers in the same roles
Emerging research on the effect of generative AI applications in the workplace finds that they increase productivity and often increase the performance of the least experienced or lowest skilled workers. For instance, Brynjolfsson, Li and Raymond (2023[17]) find that a generative AI application, which makes real-time suggestions for how customer support workers should answer calls, increases productivity by 14% defined as the number of calls resolved in an hour. The application was introduced gradually over time to allow the researchers to compare workers using the application to those who did not yet have access. The researchers find that the gains in productivity mostly accrue to the least experienced workers, suggesting that the generative AI application infers and implicitly conveys the behavioural patterns of the most productive customer support workers to those who are least skilled or experienced.
Generative AI applications may also improve workers’ writing skills. In an experiment by Noy and Zhang (2023[18]), business professionals using ChatGPT performed writing tasks in less time and produced output of higher quality than those working unaided. The researchers assigned all business professionals two writing tasks, and only suggested ChatGPT between the first and second tasks to a randomly selected subset of participants. They find that the poorest performers from the first writing task saw quality improvements and completion time decrease after exposure to ChatGPT. On the other hand, those with the highest quality writing after the first task saw no increase in quality due to the use of ChatGPT, but they did complete the writing assignment faster.
An experiment using an AI tool to help programmers write basic code also finds that generative AI applications improve productivity specifically for the least experienced. Peng et al. (2023[19]) ran an experiment where programmers were incentivised to complete a coding task as quickly as possible. A randomly selected treated group had access to CoPilot, a generative AI programme that suggests code and functions in real time depending on the context. The study finds that programmers in the treated group completed the task over 50% faster than those in the control group, and that the benefits accrued most to the least experienced programmers. Overall, these results again suggest that generative AI may decrease performance inequality in the workplace (see also Section 4.4).
4.2. Using AI is associated with higher job satisfaction and occupational safety, but there are some risks
This section reviews the nascent literature on the effect of AI on occupational safety and job satisfaction analysed through the framework of changing workplace demands and resources. It draws mostly on the OECD AI surveys and OECD AI case studies, as well as on studies in the wider literature. As with many studies of job quality and technology adoption, the results often apply only to workers who remain in firms after they have adopted AI, which may be a non-representative sample of workers exposed to AI.
4.2.1. So far, AI use appears to be associated with greater job satisfaction
Workers who use AI report higher enjoyment in their jobs. The OECD AI surveys find that more than half (63%) of AI users in finance and manufacturing reported that AI had improved enjoyment either by a little or by a lot (Lane, Williams and Broecke, 2023[8]). Despite being positive about the impact of AI on their own performance, results varied based on how workers interact with AI. The workers who reported the greatest positive effect on their enjoyment were those who develop or maintain AI, and those who manage workers who are using AI. Workers using AI or who are subject to algorithmic management were the least likely to report greater enjoyment after the introduction of AI, although most workers in each group still said it improved their enjoyment on the job (see also Section 4.3).
Other surveys of workers similarly observe that AI adoption is associated with greater worker satisfaction. Ipsos (2018[20]) polled over 6 000 workers across six OECD countries (France, Germany, Spain, the United Kingdom, the United States and Canada) in June 2018 on their feelings towards AI and effects of AI on the workplace after adoption. In all countries surveyed, at least 59% of workers interviewed in each country said that AI had positive effects on their well-being at work. Most workers also said that AI had led to positive implications for the appeal of their work.
Survey evidence from Japan similarly finds that AI adoption is correlated with greater job satisfaction. Surveying over 10 000 workers in Japan, Yamamoto (2019[21]) finds that adoption or planned AI adoption increases job satisfaction. However, the same survey finds that AI adoption is also concomitant with increased stress. Similarly, the authors find conflicting results on both job demands and resources. With the adoption of AI, workers report less routine work, but also more complex non-routine tasks.
AI is more likely to automate tedious, repetitive tasks
One reason AI may be leading to greater enjoyment on the job is that AI is more likely to automate than create repetitive tasks. The OECD AI surveys asked both workers and firms what types of tasks AI is creating or automating (see also Chapter 3). In both sectors, roughly twice as many employers said that AI had automated repetitive (greater than 50%) tasks than created them, and these differences were statistically significant (Lane, Williams and Broecke, 2023[8]).
The OECD AI case studies similarly find that AI adoption often results in fewer tedious and repetitive tasks. In finance, this was often through a reduction in simple administrative tasks. For example, a UK financial firm implemented an AI system to assist with a range of activities including mortgage underwriting, interest rate adjustments, commercial banking and brokerage. Workers view these changes as improvements to their job quality because their work has become less administrative and they saw greater value in more time spent supporting customers and colleagues across the firm (Milanez, 2023[9]). Workers also stressed the reduction of time spent on tedious tasks, giving them greater opportunities to do more research, planning and project management.
AI broadens and deepens the task content of jobs and gives workers greater autonomy in their work
AI’s ability to process and model large amounts of data can help decision making and deepen workers engagement with their work. In the OECD AI surveys, 70% and 56% of AI users in finance and manufacturing, respectively, reported that AI assisted them with decision making with overwhelmingly positive effects (Lane, Williams and Broecke, 2023[8]). The results were similarly positive when the same workers were asked whether AI helped them make better decisions and whether AI helped them make faster decisions. The same survey finds that managers – i.e. positions which rely heavily on decision making – are generally the most positive about the impact of using AI on their job quality than other workers. One potential explanation for this is that partial automation of management tasks can increase efficiency in supervision and administrative tasks or the quality of managers’ decisions, allowing them to focus on the more complex and interpersonal tasks, with potentially positive impacts on their productivity and job satisfaction.
The OECD AI surveys also find that AI adoption is associated with greater autonomy over workers’ tasks. Autonomy, in turn, appears to be positively associated with performance and working conditions. Most workers in finance (58%) and manufacturing (59%) said AI had increased the control they have over the sequence in which they perform their tasks compared to 20% and 21% of AI users who said that AI had decreased control.
In the OECD case studies, workers reported performing a greater range of tasks after the introduction of AI (Milanez, 2023[9]). A financial services provider in the United Kingdom implemented a chatbot used for customer service. The chatbot assists customers to serve themselves by directing them to the answers to frequently asked questions. Customer service representatives now handle a reduced volume of basic customer queries, which has helped diversify the range of topics they cover with customers. One representative interviewed as part of the case study summarised the shift in tasks: “The work is more interesting, definitely. It adds variety because customers don’t ask the same things every time.” She also described how the technology allows workers to form more personal relationships, making the work more rewarding.
AI can change the social environment of the workplace
Increased use of AI in the workplace may decrease human contact to the detriment of job quality. This may be, for example, because AI-powered chatbots answer workers’ questions they would otherwise ask a human co-worker or HR advisor, or because an algorithm rather than a human manager “tells” workers when their next shifts will be. Increasing use of AI in the workplace can, therefore, have negative effects on people’s well-being and productivity at work, because reducing the social dimension of work can generate an isolation feeling among workers (Nguyen and Mateescu, 2019[22]). Some experiments also show that human-machine interactions via AI-powered chatbots can increase people’s selfish behaviour (Christakis, 2019[23]), which could negatively affect the well-being of co-workers.
However, other experiments show opposite results and suggest that human-machine interactions can improve human-human interactions. For instance, Traeger et al. (2020[24]) find that people who interacted with an AI-powered social robot or chatbot while performing a task were more relaxed and conversational, laughed more and were better able to collaborate, although the effect depended on the type of social skills the robot portrayed. For instance, robots that provided neutral fact-based statements were not as successful in improving human-human interactions as those that admitted mistakes or told jokes. See Chapter 6 for a discussion of the policy responses to a lack of human determination and human interaction due to AI use in the workplace.
4.2.2. AI is associated with improved mental health and physical safety but also greater work intensity
AI generally leads to improved mental health and physical safety
AI systems may be able to help with occupational safety and health. Monitoring systems can help alert workers who are at risk of stepping too close to dangerous equipment, for example, or who may not be following safety procedures (Wiggers, 2021[25]). AI systems are also being developed to detect non-verbal cues, including body language, facial expressions and tone of voice: these systems can be used in the workplace to detect workers who are overworked and those whose mental well-being is at risk (Condie and Dayton, 2020[26]).10 For example, train drivers on the Beijing-Shanghai high-speed rail line were wearing “brain monitoring devices” inserted into their caps. The firm manufacturing these devices claims these devices measure different types of brain activities, including fatigue and attention loss, with an accuracy of more than 90%. If the driver falls asleep, the cap triggers an alarm (Chen, 2018[27]). These monitoring systems, however, often entail extensive data collection and the accompanying risks to data protection.
The OECD AI surveys find that mental health and physical health improved after the introduction of AI, although the benefits are not enjoyed evenly. In manufacturing, 55% of workers using AI said that AI had improved their mental health and 54% in finance. In manufacturing, over 60% of workers using AI reported greater physical health as well.11 Men and workers with a tertiary education report much higher rates of mental and physical health improvements compared to women and those who do not have a tertiary degree, with under 40% of the latter groups reporting improved mental and physical health in the finance sector (Lane, Williams and Broecke, 2023[8]).
The OECD AI case studies confirm AI’s role in improving physical safety. In many of the worker responses, AI implementation was reported as improving physical safety by improving the capabilities of dangerous machines, which then allowed workers to be physically separated from them. For example, an Austrian manufacturer implemented an AI software that controls a straightening machine used to correct the concentricity of steel rods. Before the introduction of AI, workers controlled the straightening which could lead to accidents, but now workers simply need to monitor the machine from behind a barrier (Milanez, 2023[9]). To give a further example, the rise of AI and notably computer vision, has allowed sophisticated trash-sorting robots to be deployed to recycling plants – at present, recycling workers face some of the highest risk of workplace incident (Nelson, 2018[28]).12 The previous examples show how the use of robots with embedded AI can “[remove] workers from hazardous situations” (EU-OSHA, 2021[29]).
AI may also lead to a higher pace and intensity of work
The introduction of AI may also result in a faster pace of work. The OECD AI surveys find that 75% of surveyed workers in finance, and 77% in manufacturing who use AI reported that AI had increased the pace at which they performed their tasks. These workers were more than five times more likely to report that AI had increased pace than decreased it (Lane, Williams and Broecke, 2023[8]). However, the authors caution that the increase in work pace may not necessarily lead to greater stress because it is often concomitant with greater worker control over the sequence used to complete tasks (see above).13
The OECD AI case studies provide further evidence that AI may lead to increasing intensity of work. AI applications may automate the “easy” set of a worker’s tasks leaving them with the same amount of work, but without the “break” afforded by the easy problems. For example, an AI developer interviewed as part of the case studies from a Canadian manufacturing firm reported that they purposefully did not automate all of the easy tasks so that workers could benefit from the mental health break when they are completing fewer demanding tasks (Milanez, 2023[9]). This example showed a keen awareness of the stress to workers from completing more tasks of greater intensity at a higher pace. It is not obvious, however, that all firms are adopting AI in the same way.
4.3. AI-led reorganisation or automation of managers’ tasks has downstream effects on their subordinates
AI can help managers in their jobs, for instance, by providing training recommendations tailored to individual workers, or by optimising work schedules according to team members’ preferences and availability. AI can also fully automate some or all managerial tasks, for instance by automatically assigning shifts to workers without the need for any human intervention. These various forms of “algorithmic management” (see Box 4.3) can affect the job quality of subordinates regardless of whether they interact with AI to do their jobs. The impact of algorithmic management on subordinates’ job quality may depend on the degree of AI involvement in managerial decision making, although evidence differentiating between supported and automated management decisions is still lacking. For example, a human manager can take their knowledge of a workers’ team spirit into account – data that may not be recorded and therefore cannot factor in AI systems’ decision making.
Box 4.3. Algorithmic management
Algorithmic management consists of using AI to either support or automate management decisions – such as deciding who should receive a bonus, training or promotion – or other managerial tasks such as monitoring workers.1 For instance, AI can select CVs that have the best fit with a job description while respecting diversity criteria, it can optimise the assignment of tasks or training to workers based on their characteristics and preferences, and it can monitor large numbers of workers at any time and location.
Although some AI systems may be capable of performing each of these tasks independently without the need for any human intervention (i.e., full automation in algorithmic management), the most likely scenario is that managers receive AI-powered recommendations which they can (but do not have to) implement in their own decision making. For instance, managers are usually able to review and overrule algorithmic evaluations of workers or automatic shift assignments (Wood, 2021[30]). It will be important to ensure that managers are able to critically evaluate and overrule AI-powered recommendations.
Regulating algorithmic management
One of the reasons why full automation in algorithmic management is rare, is that it is limited by regulation in several countries. For instance, the European General Data Protection Regulation (GDPR) effectively prohibits algorithmic management that entails fully automatic decision making, and many non-European countries have GDPR-like legislation as well – see Chapter 6.
While algorithmic management is increasingly used in warehouses, retail and hospitality, and manufacturing (Briône, 2020[31]; Wood, 2021[30]; Jarrahi et al., 2021[32]), it remains most common in platform work (e.g. to assign shifts to riders). The European Union’s Platform Work Directive is a landmark legislative proposal, designed to address the challenges posed by algorithmic management in platform work. With a focus on ensuring fair working conditions, the directive aims to regulate the use of algorithms by platforms to manage and monitor workers. It places a strong emphasis on transparency and accountability, requiring platforms to provide clear information about the functioning and impact of algorithms on workers’ rights and performance evaluations. The directive also promotes the right to collective representation, enabling workers to negotiate and challenge algorithmic management practices. By tackling algorithmic bias, enhancing transparency, and empowering workers, the EU Platform Work Directive seeks to establish a more balanced and human-centric approach to platform work within the European Union (European Commission, 2021[33]).
1. A common, related, term is people analytics which describes the use of statistical tools, including AI systems, to measure, report and understand the workforce’s performance in various dimensions (Briône, 2020[31]).
The OECD AI surveys find that around 7% of workers in manufacturing and 6% of those in finance report that they are managed by AI. This could either mean that they are aware that their manager receives AI-powered recommendations, or that certain or all managerial tasks are automated. In both sectors, men are more likely to report being subject to algorithmic management than women (8% compared to 5%, respectively). Being subject to algorithmic management is also more common among respondents with a university degree (8%, compared to 5% among those without a university degree) and among those who are born in another country than where they work (9% compared to 6% among natives).
Despite being overall positive about the impact of AI on their own performance and working conditions, respondents who are subject to algorithmic management are less positive than respondents who interact with AI in another way (see Figure 4.1). Compared to other interactions with AI, being subject to algorithmic management appears to be particularly less beneficial for workers’ own job performance.
These results might, however, be affected by the fact that the survey question on algorithmic management can only identify workers who are aware that they are subject to it. While workers who are subject to fully automated managerial tasks are probably aware of it, they may not be aware that their manager is “merely” supported by AI in their decision making. Although this means that the results in this section may be driven by workers who are subject to full automation of some managerial tasks, it is uncertain if and how the impact on their job quality would differ from that of workers whose manager is supported by AI.
Possible reasons why workers who are subject to algorithmic management are less positive about AI’s impact on their jobs include: the risk of increased work intensity, loss of worker autonomy, and privacy infringements. This section discusses these three risks in further detail. The use of algorithmic management also raises a more fundamental question about whether fully automated decision making should be allowed in the workplace for decisions that affect people’s opportunities and well-being – an issue addressed in Chapter 6.
Figure 4.1. Workers who are subject to algorithmic management benefit less from AI
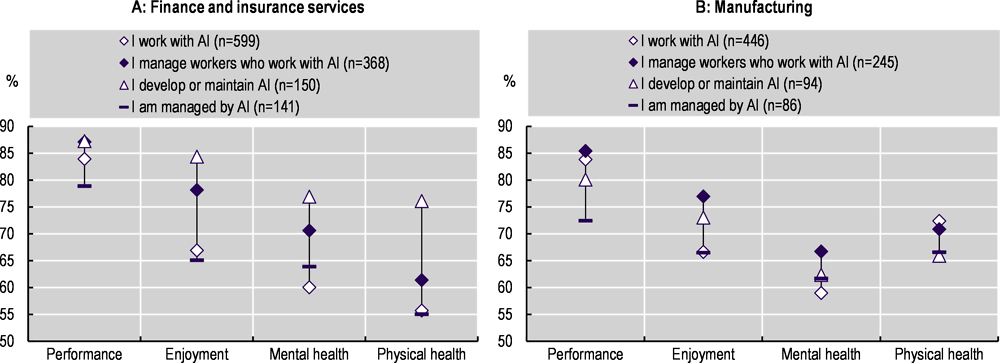
Note: Workers in companies that have adopted AI were asked: “Which of these statements best describes your interaction with AI at work: I work with AI; I manage workers who work with AI; I develop/maintain AI; I am managed by AI; I interact with AI in another way; I have no interaction with AI at work” (Exclusive response option. Otherwise, respondents could select multiple answers). Those who interact with AI were then asked: “How do you think AI has changed your own job performance (performance)/how much you enjoy your job (enjoyment)?/your physical health and safety in the workplace (physical health)?/your mental health and well-being in the workplace (mental health)?” The figure shows the proportion of AI users who said that each of these outcomes were improved (a lot or a little) by AI.
Source: Lane, Williams and Broecke (2023[8]), “The impact of AI on the workplace: Main findings from the OECD AI surveys of employers and workers”, https://doi.org/10.1787/ea0a0fe1-en.
4.3.1. Algorithmic management raises risks for increased work intensity
Algorithmic management can increase work intensity. The constant and pervasive monitoring and data-driven performance evaluations made possible by AI can create a high-stress environment with negative impacts on mental health, as employees may feel constantly scrutinised and under pressure to perform. Additionally, workers who are subject to excessive monitoring may feel compelled to sacrifice their personal time and work-life balance. For example, AI-led telematics systems used to monitor and manage delivery drivers are often introduced with the declared intention to increase drivers’ safety, but they put such pressure on drivers to “beat their time” that the resulting work intensification decreases workplace safety. In some warehouses, wearable AI-powered devices to monitor and manage workers score employees and communicate picking targets continuously. Combined with the threat of layoff, this can generate increased work intensity, leading to heightened stress and physical burnout, and create potentially physically dangerous situations at work (Moore, 2018[34]). In some countries, workers’ legal right to disconnect should offer protection against this (e.g. Belgium, France, Italy and Spain (Eurofound, 2021[35]).
The results from the OECD AI surveys confirm that there is a risk that algorithmic management increases work intensity, although this appears to depend on the context in which it is implemented. Among workers in finance, 85% of those who report being subject to algorithmic management experienced an increase in their pace of work due to AI, compared to 74% among those who interact with AI in another way (Figure 4.2). However, in manufacturing, the share of workers who experienced an increase in their pace of work due to AI is similar for those who are subject to algorithmic management and those who interact with AI in another way (76% and 78%, respectively).
Figure 4.2. The pace of work of subordinates increases with algorithmic management
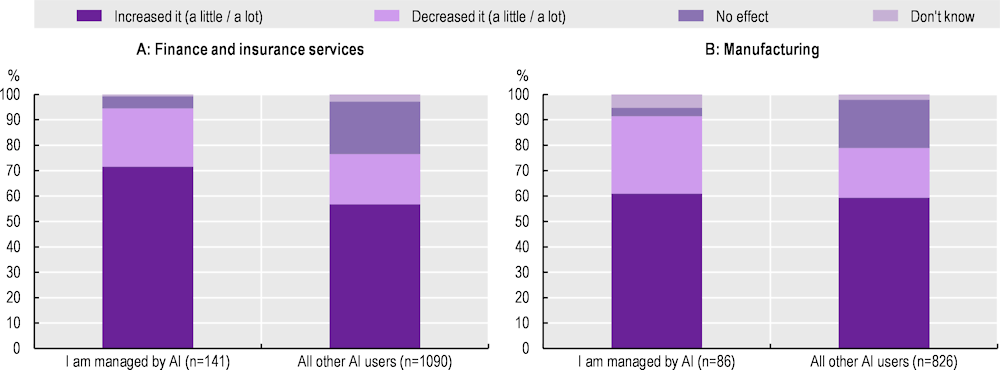
Note: AI users were asked: “How has AI changed how you work, in terms of the pace at which you perform your tasks?”
Source: Lane, Williams and Broecke (2023[8]), “The impact of AI on the workplace: Main findings from the OECD AI surveys of employers and workers”, https://doi.org/10.1787/ea0a0fe1-en.
4.3.2. Algorithmic management affects subordinates’ space for autonomy
There is a risk that systematic management and monitoring through AI systems reduces space for workers’ autonomy and sense of control over how to execute their tasks, especially if taken to the extreme and involving the full automation of managerial tasks. For instance, evidence on warehouse workers subject to full automation in algorithmic management shows that these workers are often denied the ability to make marginal decisions about how to execute their work, or even how to move their own limbs (Briône, 2020[31]). Devices used in some call centres give feedback to employees on the strength of their emotions to alert them of the need to calm down (Briône, 2020[31]). Other industries – including consultancy, banking, hotels and platform work – are also adopting software that enables continuous real-time performance reviews either by managers or by clients (Wood, 2021[30]). These extreme levels of monitoring and performance feedback made possible by algorithmic management can make workers feel commoditised, and it can create a sense of alienation (Fernández-Macias et al., 2018[36]; Bucher, Fieseler and Lutz, 2019[37]; Frischmann and Selinger, 2018[38]; Maltseva, 2020[39]; Jarrahi et al., 2021[32]). It may also decrease employees’ engagement with work, since for many, work is an integral part of finding meaning and purpose in life (Saint-Martin, Inanc and Prinz, 2018[40]; Hegel, 1807[41]; OECD, 2014[42]; Bowie, 1998[43]).
A lack of transparency and explainability of decisions based on AI systems would also reduce workers’ autonomy. For example, not providing explanations for decisions affecting them does not enable workers to adapt their behaviour in ways to improve their performance (Loi, 2020[44]). Additionally, a lack of transparency and explainability of AI systems can create a sense of arbitrariness in algorithmic decision making, thereby decreasing trust in these systems and hindering the possibility to contest or redress wrongful outcomes – see Chapter 6.
While the OECD AI surveys find that, on average, AI adoption is associated with greater autonomy over workers’ tasks (see Section 4.2.1), respondents who report that they are subject to algorithmic management have more polarised views on their sense of control than the overall sample. A larger proportion of those managed by AI report that AI increased their autonomy in finance, while the opposite is true in manufacturing – see Figure 4.3. These findings suggest that the impact of algorithmic management on workers’ sense of autonomy depends on the way and the context in which it is implemented.
Figure 4.3. Algorithmic management impacts subordinates’ sense of control over their tasks
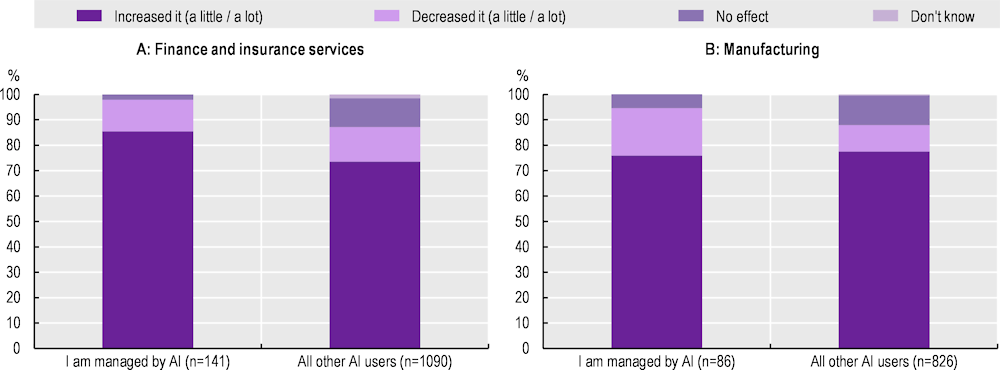
Note: AI users were asked: “How has AI changed how you work, in terms of the control you have over the sequence in which you perform your tasks?”
Source: Lane, Williams and Broecke (2023[8]), “The impact of AI on the workplace: Main findings from the OECD AI surveys of employers and workers”, https://doi.org/10.1787/ea0a0fe1-en.
4.3.3. Algorithmic management raises risks for workers’ privacy
Even if the extensive worker surveillance made possible by AI systems may not be legal in many OECD countries (see Chapter 6), some AI-powered monitoring and surveillance software include features leaving very little privacy to workers. While managers monitoring their workers is not a new phenomenon, the type of data that can be processed by using AI is much more elaborate than data processed by humans or other technologies (Ajunwa, Crawford and Schultz, 2017[45]; Sánchez-Monedero and Dencik Sanchez-Monederoj, 2019[46]). This can lead to infringements of workers’ privacy, which not only violates their fundamental rights (see Chapter 6), but privacy infringements and cybersecurity breaches (or fears thereof) can also have psychosocial impacts and affect people’s well-being at work more generally.
The use of remote surveillance software has exponentially increased as workers shifted to mass teleworking during the COVID‑19 crisis. For example, ActivTrak, an AI-powered tool that continuously monitors how employees are using their time on computers, went from 50 client companies to 800 in March 2020 alone. Teramind, which uses AI to provide automatic real-time insights in employee behaviour on their computers, such as unauthorised access to sensitive files, reported a triple‑digit percentage increase in new leads since the pandemic began (Morrison, 2020[47]).
Wearable devices that capture sensitive physiological data on workers’ health conditions, habits, and possibly the nature of their social interaction with other people are another example. While this information can be collected and used to improve employees’ health and safety, it can also be used by employers to inform consequential judgments (Maltseva, 2020[39]). Chapter 7 discusses the risk that employers use AI-powered worker surveillance to detect workers’ intention to organise as a trade union.
The OECD AI surveys show that, among workers who report that their employers’ use of AI involves the collection of data on workers or how they do their work,14 more than half expressed worries regarding their privacy (Lane, Williams and Broecke, 2023[8]). Additionally, 58% and 54% in finance and manufacturing, respectively, said that they worry that too many of their data are being collected.
4.4. The impact of AI on inclusiveness and bias in the labour market
The adoption of AI is likely to improve job quality for some groups of workers, but it could worsen it for others, affecting labour market inclusiveness. At the same time, AI systems can help reduce bias in the workplace and strengthen fairness, but only if bias is addressed in AI’s development and implementation.
Labour market inclusiveness and fairness are relevant for job quality (Barak and Levin, 2002[48]; Brimhall et al., 2022[49]). Disadvantaged workers (e.g. workers with disabilities, ethnic minorities, or non-native speakers) tend to have better access to jobs of good quality in inclusive labour markets with benefits for their job satisfaction, stress levels and sense of fairness. Moreover, there can be positive spill-over effects on the job quality of other workers who value fairness in the workplace.
4.4.1. AI can improve inclusiveness for some disadvantaged groups but not for others
By improving accessibility of the workplace for workers that are typically at a disadvantage in the labour market, AI can improve inclusiveness in the workplace. AI-powered assistive devices to aid workers with visual, speech or hearing impairments, or prosthetic limbs, are becoming more widespread, improving the access to, and the quality of work for people with disabilities (Smith and Smith, 2021[50]; Touzet, forthcoming[51]). For example, speech recognition solutions for people with dysarthric voices, or live captioning systems for deaf and hard of hearing people can facilitate communication with colleagues and access to jobs where inter-personal communication is necessary.
AI can also enhance the capabilities of low-skilled workers, with potentially positive effects on their wages and career prospects. For example, AI’s capacity to translate written and spoken word in real-time can improve the performance of non-native speakers in the workplace. Moreover, recent developments in AI-powered text generators, such as ChatGPT, can instantly improve the performance of lower-skilled individuals in domains such as writing, coding or customer service (see Box 4.2).
At the same time, some AI systems that are used in the workplace are harder if not impossible to use for individuals with low levels of digital skills such as the low-skilled more generally, older workers or women, with negative impacts on inclusiveness. Although using AI may only require moderate digital skills, in the OECD on average, more than a third of adults lack even the most basic digital skills (Verhagen, 2021[52]). Additionally, the availability and quality of translations and other AI-generated text depends on the number of users that speak a certain language, leading to inequalities in who can benefit from the technology (Blasi, Anastasopoulos and Neubig, 2022[53]).
Employers and workers often have conflicting views of AI’s potential to improve the inclusiveness for disadvantaged groups. Almost half of the employers in finance and manufacturing surveyed in the OECD AI surveys believe that AI would help workers with disabilities, but for low-skilled and older workers, employers are more likely to say that AI would harm rather than benefit them (Figure 4.4). Although employers think that women are more likely to be helped than harmed by AI, female AI users are, themselves, less positive about AI’s impact on their job quality than men, and part of this difference remains when controlling for the fact that male and female AI users tend to have different occupations (Lane, Williams and Broecke, 2023[8]). The findings thus raise questions about whether the current uses of AI could risk leaving certain workers behind as AI diffuses more widely. Chapter 5 discusses in detail the impact of AI on skills and the implications for inclusiveness.
Figure 4.4. Employers think that workers with disabilities are likely to be helped by AI, while older and low-skilled workers could face some harm
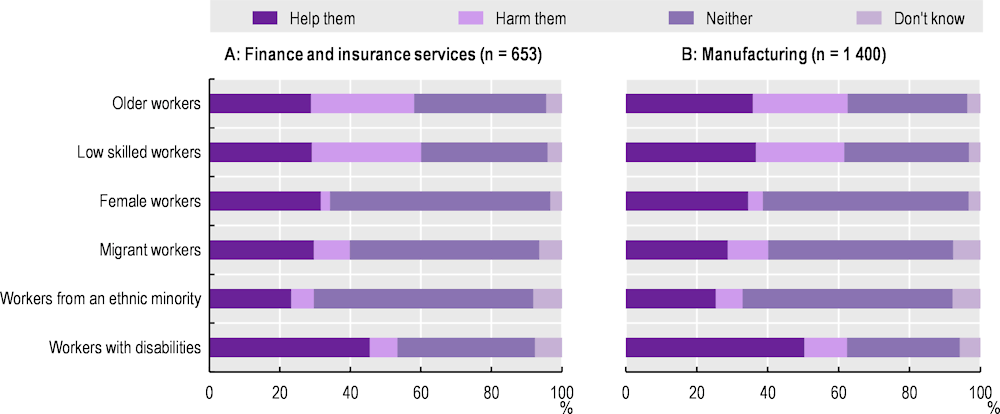
Note: All employers (regardless of whether they adopted AI) were asked: “I’m going to name a few different groups of workers. For each of them, please tell me whether you think artificial intelligence is more likely to help them or harm them or neither help nor harm them in their work.”
Source: Lane, Williams and Broecke (2023[8]), “The impact of AI on the workplace: Main findings from the OECD AI surveys of employers and workers”, https://doi.org/10.1787/ea0a0fe1-en.
4.4.2. If not designed and implemented well, AI may systematise existing human biases
AI systems used in the workplace are still not always designed and implemented in ways that are unbiased and can therefore have different effects on job quality across groups. For example, since AI can promote more objective performance evaluations (Broecke, 2023[54]), it could bring better opportunities for recognition and promotions for workers who have traditionally suffered from bias in the labour market, such as women or older workers. However, if AI replicates existing biases the effect will be the opposite, leading to more systematic discrimination. The potential for AI to increase fairness in the workplace resulting in greater job quality and inclusiveness hinges on how well AI overcomes and mitigates inherent biases.
The OECD AI surveys find that 45% and 43% of workers who use AI in finance and manufacturing, respectively, think that AI has improved how fairly their manager or supervisor treats them (Figure 4.5). These positive results notwithstanding, around one in ten AI users think that AI has worsened fairness in management. This suggests that AI’s impact on fairness depends on the way the system is designed and used by managers.
Figure 4.5. AI has the potential to improve fairness in management, but not for all workers
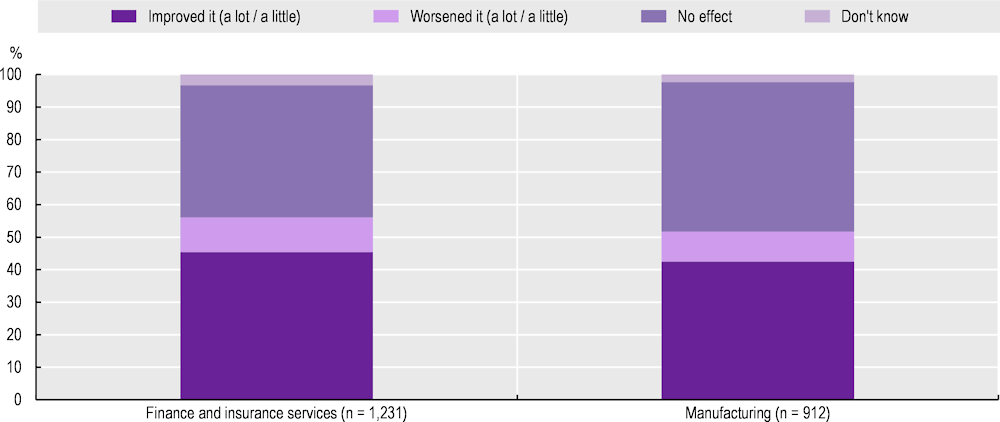
Note: AI users were asked: “How do you think AI has changed how fairly your manager or supervisor treats you (fairness in management)?”
Source: Lane, Williams and Broecke (2023[8]), “The impact of AI on the workplace: Main findings from the OECD AI surveys of employers and workers”, https://doi.org/10.1787/ea0a0fe1-en.
Bias in AI systems can emerge both at the data or input level and at the system level (Accessnow, 2018[55]; Executive Office of the President of the United States, 2016[56]). At the system level, the choice of parameters and the choice of data on which to train the system are decisions made by humans based on their own judgements. The lack of diversity in the tech industry poses risks in this respect (West, Whittaker and Crawford, 2019[57]). Bias can also be introduced at the data level, as data can be incomplete, incorrect or outdated, and reflect historical biases.
Bias can occur at all points of use of AI systems in the workplace. In hiring – while offering great promise for improving matching between labour demand and supply – there is evidence of bias in who can see job postings, and in the selection and ranking of candidates when AI systems are used (see Chapter 3) (Broecke, 2023[54]). Bias can also emerge when AI systems are used to evaluate performance in the workplace. If the data on which the systems are trained are biased, then seemingly neutral automated predictions about performance will be biased themselves. In addition, when assessing performance, AI systems would not be able to take into account context information, such as personal circumstances, typically not coded in the data used by the algorithm.
The use of AI-powered facial recognition systems to authenticate workers when accessing the workplace or workplace tools also presents challenges linked to bias. Facial recognition systems have been found to perform worse for people of colour (Harwell, 2021[58]), and several researchers question AI’s ability to accurately detect emotions and non-verbal cues across different cultures (Condie and Dayton, 2020[26]).
However, bias is widespread in human decisions as well. A meta‑analysis of 30 years of experiments in the United States found that white job applicants were 36% more likely to receive a call-back than equally qualified African Americans, and 24% more likely than Latinos – with little significant evolution between 1989 and 2015 (Quillian et al., 2017[59]). However, compared with biased human-led decision making, a systematic use of biased AI systems carries the risk of multiplying and systematising bias (Institut Montaigne, 2020[60]), reinforcing historical patterns of disadvantage (Kim, 2017[61]; Sánchez-Monedero and Dencik Sanchez-Monederoj, 2019[46]). Furthermore, discrimination in AI systems is more unintuitive and difficult to detect, which challenges the legal protection offered by non-discrimination law (Wachter, Mittelstadt and Russell, 2021[62]). The challenge for policy is to encourage AI and humans as complements to ensure they collectively reduce bias.
Recent research on the use of AI systems in the workplace highlights both the potential of AI to surmount bias, improve workplace productivity and improve inclusiveness, but also how AI may perpetuate existing inequalities if not implemented properly. Li, Raymond and Berman (2020[63]) designed machine learning algorithms to screen resumes and decide whether to grant first round interviews for high-skill positions in a Fortune 500 company. They show that an algorithm that only uses past firm data to decide who to interview improves quality (as measured by hiring yield) compared to human reviewers, but at the expense of decreased representation for minority candidates. They additionally show that when the algorithm is adapted to not only exploit past data, but to explore candidates who were under-represented in historical data, the algorithm both improves quality and inclusiveness. Crucially, the algorithm is not incentivised to incorporate inclusiveness; it does so by occasionally exploring under-represented profiles because they have higher variance in the data. The results show that AI applications can improve productivity and inclusiveness, but only if they are thoughtfully designed.
4.5. Concluding remarks
This chapter reviews the current literature on the effect of AI on job quality and inclusiveness. There is evidence that workers with AI skills earn a substantial wage premium, even over similar workers with in-demand skills (for example, software skills). However, for the wider set of workers who are exposed to AI but do not necessarily have AI skills, the effect on wages is inconclusive so far. Taking a broader view of job quality which includes the sum of demands on workers and resources available, AI adoption appears to be associated with greater job satisfaction. This is likely because AI automates more tedious tasks than it creates, and it may also help to improve mental health and the physical safety of workers. The latter is often achieved by improving existing machines to the point where workers no longer need to work with them in close proximity. The evidence points to managers, workers with AI skills, and workers with tertiary degrees enjoying improved job quality.
The discussion of job quality is not limited to wages or job demands and resources, but how AI shapes the broader working environment. AI can change how managers execute their tasks and, in some instances, may even become the manager. While the overall impact of AI on job performance and working conditions tends to be positive, in the OECD AI surveys, respondents who are subject to algorithmic management are often less positive than those who interact with AI in other ways. Some are also more likely to say that AI has reduced their autonomy and increased their work intensity. Additionally, most workers are worried about their privacy when their employers’ use of AI includes data collection on workers and, specifically, how they do their job.
The impact of AI on job satisfaction and workers’ health differs across groups of workers, with risks for workplace inclusiveness. Workers in managerial roles, those with the skills to develop and maintain AI systems, and workers with tertiary degrees tend to report higher job satisfaction and improved health after AI adoption. AI systems may also struggle with bias, both at the data and at the system levels. While bias is widespread in human decisions as well, the use of biased AI systems carries the risk of multiplying and systematising biases, leading to decreased inclusiveness of the workplace, with negative impacts on the job satisfaction of disadvantaged groups as well as their colleagues.
Policy can promote AI implementation in the workplace that enhances job quality. Some OECD countries, for example, enable workers to opt-out of full automation in algorithmic management by providing individuals with a right to meaningful human input for important decisions that affect them. Chapter 6 provides a detailed discussion of countries’ policy efforts regarding privacy, full automation in algorithmic management, and measures to prevent and address bias linked to the use of AI systems in the workplace, amongst others. Chapter 7 provides a discussion of the current challenges and opportunities for social partners brought about by AI adoption. Skills policies and agile workplace training systems can also help workers benefit from AI. Chapter 5 provides policy lessons for skills and training with particular attention focused on the broader proliferation of AI in the workplace.
Finally, while this chapter reviews the current literature on the impact of AI on job quality and inclusiveness, it should be viewed as a springboard for further research rather than the final word. The literature on the effect of AI on wages – one of the most vital questions facing AI and the labour market – has focused mostly on the tiny set of workers who have AI skills. There are comparatively fewer empirical studies on the much larger set of workers who will use AI but have no specialised training in computer science or machine learning. For the broader picture of job quality considering all job demands and resources, this chapter has relied extensively on the OECD AI surveys and case studies. These studies have already moved the literature forward greatly, but they rely on just two industries, and they cannot account for unobserved worker selection after AI adoption. Further research is needed on these topics, not only to confirm the findings in this chapter, but because AI is a fast-moving field, and the studies in this chapter are fundamentally backward looking. As AI’s capabilities evolve, what is true today may not hold in the near future.
References
[55] Accessnow (2018), Human rights in the age of artificial intelligence, Accessnow, https://www.accessnow.org/cms/assets/uploads/2018/11/AI-and-Human-Rights.pdf.
[13] Acemoglu, D. et al. (2022), Automation and the Workforce: A Firm-Level View from the 2019 Annual Business Survey, National Bureau of Economic Research, Cambridge, MA, https://doi.org/10.3386/w30659.
[2] Acemoglu, D. and P. Restrepo (2018), “The Race between Man and Machine: Implications of Technology for Growth, Factor Shares, and Employment”, American Economic Review, Vol. 108/6, pp. 1488-1542, https://doi.org/10.1257/aer.20160696.
[45] Ajunwa, I., K. Crawford and J. Schultz (2017), “Limitless Worker Surveillance”, California Law Review, Vol. 105/3, pp. 735-776, https://doi.org/10.15779/Z38BR8MF94.
[6] Alekseeva, L. et al. (2021), “The demand for AI skills in the labor market”, Labour Economics, Vol. 71, p. 102002, https://doi.org/10.1016/j.labeco.2021.102002.
[16] Babina, T. et al. (2020), “Artificial Intelligence, Firm Growth, and Industry Concentration”, SSRN Electronic Journal, https://doi.org/10.2139/ssrn.3651052.
[48] Barak, M. and A. Levin (2002), “Outside of the corporate mainstream and excluded from the work community: A study of diversity, job satisfaction and well-being”, Community, Work & Family, Vol. 5/2, pp. 133-157, https://doi.org/10.1080/13668800220146346.
[53] Blasi, D., A. Anastasopoulos and G. Neubig (2022), “Systematic Inequalities in Language Technology Performance across the World’s Languages”, Proceedings of the 60th Annual Meeting of the Association for Computational Linguistics (Volume 1: Long Papers), https://doi.org/10.18653/v1/2022.acl-long.376.
[66] BLS (2021), 2020 Census of Fatal Occupational Injuries (CFOI), U.S. Bureau of Labour Statistics, https://www.bls.gov/iif/oshcfoi1.htm (accessed on 16 March 2022).
[43] Bowie, N. (1998), “A Kantian Theory of Meaningful Work”, Journal of Business Ethics, Vol. 17/9/10, pp. 1083-1092, https://www.jstor.org/stable/25073937.
[49] Brimhall, K. et al. (2022), “Workgroup Inclusion Is Key for Improving Job Satisfaction and Commitment among Human Service Employees of Color”, Human Service Organizations: Management, Leadership & Governance, Vol. 46/5, pp. 347-369, https://doi.org/10.1080/23303131.2022.2085642.
[31] Briône, P. (2020), My boss the algorithm: an ethical look at algorithms in the workplace, Acas, https://www.acas.org.uk/my-boss-the-algorithm-an-ethical-look-at-algorithms-in-the-workplace/html (accessed on 21 October 2021).
[54] Broecke, S. (2023), “Artificial intelligence and labour market matching”, OECD Social, Employment and Migration Working Papers, No. 284, OECD Publishing, Paris, https://doi.org/10.1787/2b440821-en.
[17] Brynjolfsson, E., D. Li and L. Raymond (2023), Generative AI at Work, National Bureau of Economic Research, Cambridge, MA, https://doi.org/10.3386/w31161.
[64] Brynjolfsson, E., T. Mitchell and D. Rock (2018), “What Can Machines Learn and What Does It Mean for Occupations and the Economy?”, AEA Papers and Proceedings, Vol. 108, pp. 43-47, https://doi.org/10.1257/pandp.20181019.
[37] Bucher, E., C. Fieseler and C. Lutz (2019), “Mattering in digital labor”, Journal of Managerial Psychology, Vol. 34/4, pp. 307-324, https://doi.org/10.1108/JMP-06-2018-0265.
[15] Calvino, F. and L. Fontanelli (2023), “A portrait of AI adopters across countries: Firm characteristics, assets’ complementarities and productivity”, OECD Science, Technology and Industry Working Papers, No. 2023/02, OECD Publishing, Paris, https://doi.org/10.1787/0fb79bb9-en.
[14] Calvino, F. et al. (2022), “Identifying and characterising AI adopters: A novel approach based on big data”, OECD Science, Technology and Industry Working Papers, No. 2022/06, OECD Publishing, Paris, https://doi.org/10.1787/154981d7-en.
[27] Chen, S. (2018), “‘Forget the Facebook leak’: China is mining data directly from workers’ brains on an industrial scale”, South China Morning Post, https://www.scmp.com/news/china/society/article/2143899/forget-facebook-leak-china-mining-data-directly-workers-brains (accessed on 16 March 2022).
[23] Christakis, N. (2019), Will Robots Change Human Relationships?, The Atlantic, https://www.theatlantic.com/magazine/archive/2019/04/robots-human-relationships/583204/ (accessed on 18 October 2021).
[26] Condie, B. and L. Dayton (2020), “Four AI technologies that could transform the way we live and work”, Nature, Vol. 588/7837, https://doi.org/10.1038/d41586-020-03413-y.
[67] Czarnitzki, D., G. Fernández and C. Rammer (2022), “Artificial Intelligence and Firm-Level Productivity”, ZEW Discussion Paper, No. 22-005, ZEW, Mannheim, https://ftp.zew.de/pub/zew-docs/dp/dp22005.pdf.
[3] Dube, A., L. Giuliano and J. Leonard (2019), “Fairness and Frictions: The Impact of Unequal Raises on Quit Behavior”, American Economic Review, Vol. 109/2, pp. 620-663, https://doi.org/10.1257/aer.20160232.
[29] EU-OSHA (2021), Impact of Artificial Intelligence on Occupational Safety and Health, EU-OSHA, https://osha.europa.eu/en/publications/osh-and-.
[35] Eurofound (2021), Right to disconnect, EurWORK, https://www.eurofound.europa.eu/observatories/eurwork/industrial-relations-dictionary/right-to-disconnect (accessed on 1 June 2023).
[33] European Commission (2021), Improving working conditions in platform work, https://ec.europa.eu/commission/presscorner/detail/en/ip_21_6605 (accessed on 11 May 2023).
[56] Executive Office of the President of the United States (2016), Big Data: A Report on Algorithmic Systems, Opportunity, and Civil Rights Executive Office of the President Big Data: A Report on Algorithmic Systems, Opportunity, and Civil Rights, https://obamawhitehouse.archives.gov/sites/default/files/microsites/ostp/2016_0504_data_discrimination.pdf.
[10] Felten, E., M. Raj and R. Seamans (2021), “Occupational, industry, and geographic exposure to artificial intelligence: A novel dataset and its potential uses”, Strategic Management Journal, Vol. 42/12, pp. 2195-2217, https://doi.org/10.1002/smj.3286.
[11] Felten, E., M. Raj and R. Seamans (2019), “The Variable Impact of Artificial Intelligence on Labor: The Role of Complementary Skills and Technologies”, SSRN Electronic Journal, https://doi.org/10.2139/ssrn.3368605.
[36] Fernández-Macias, E. et al. (2018), Game changing technologies: Exploring the impact on production processes and work, Eurofound, https://www.eurofound.europa.eu/sites/default/files/ef_publication/field_ef_document/fomeef18001en.pdf.
[12] Fossen, F. and A. Sorgner (2019), “New Digital Technologies and Heterogeneous Employment and Wage Dynamics in the United States: Evidence from Individual-Level Data”, IZA Discussion Paper Series, No. 12242, IZA, Bonn, Germany, https://docs.iza.org/dp12242.pdf.
[38] Frischmann, B. and E. Selinger (2018), Re-Engineering Humanity, Cambridge University Press, https://doi.org/10.1017/9781316544846.
[5] Green, A. and L. Lamby (2023), “The supply, demand and characteristics of the AI workforce across OECD countries”, OECD Social, Employment and Migration Working Papers, No. 287, OECD Publishing, Paris, https://doi.org/10.1787/bb17314a-en.
[58] Harwell, D. (2021), “Contract lawyers face a growing invasion of surveillance programs that monitor their work”, The Washington Post, https://www.washingtonpost.com/technology/2021/11/11/lawyer-facial-recognition-monitoring/ (accessed on 10 January 2022).
[41] Hegel, G. (1807), The Phenomenology of Spirit, Oxford University Press.
[4] Heinz, M. et al. (2020), “Measuring the Indirect Effects of Adverse Employer Behaviour on Worker Productivity: a field Experiment”, The Economic Journal, Vol. 130/632, pp. 2546-2568, https://doi.org/10.1093/ej/ueaa038.
[60] Institut Montaigne (2020), Algorithms: Please Mind the Bias!, https://www.institutmontaigne.org/ressources/pdfs/publications/algorithms-please-mind-bias.pdf.
[20] Ipsos (2018), The revolution of AI at work, https://www.ipsos.com/sites/default/files/ct/publication/documents/2018-06/revolution_of_ai_at_work-2018.pdf.
[32] Jarrahi, M. et al. (2021), “Algorithmic management in a work context”, Big Data & Society, Vol. 8/2, p. 205395172110203, https://doi.org/10.1177/20539517211020332.
[61] Kim, P. (2017), “Data-Driven Discrimination at Work”, William & Mary Law Review, Vol. 48, pp. 857-936, https://scholarship.law.wm.edu/wmlr/vol58/iss3/4/.
[8] Lane, M., M. Williams and S. Broecke (2023), “The impact of AI on the workplace: Main findings from the OECD AI surveys of employers and workers”, OECD Social, Employment and Migration Working Papers, No. 288, OECD Publishing, Paris, https://doi.org/10.1787/ea0a0fe1-en.
[63] Li, D., L. Raymond and P. Bergman (2020), Hiring as Exploration, National Bureau of Economic Research, Cambridge, MA, https://doi.org/10.3386/w27736.
[44] Loi, M. (2020), People Analytics must benefit the people - An ethical analysis of dtat-driven algorithmic systems in human resources management, Algorithm Watch, https://algorithmwatch.org/en/auto-hr/ethical-analysis-loi/.
[39] Maltseva, K. (2020), “Wearables in the workplace: The brave new world of employee engagement”, Business Horizons, Vol. 63/4, https://doi.org/10.1016/j.bushor.2020.03.007.
[7] Manca, F. (2023), “Six questions about the demand for artificial intelligence skills in labour markets”, OECD Social, Employment and Migration Working Papers, No. 286, OECD Publishing, Paris, https://doi.org/10.1787/ac1bebf0-en.
[9] Milanez, A. (2023), “The impact of AI on the workplace: Evidence from OECD case studies of AI implementation”, OECD Social, Employment and Migration Working Papers, No. 289, OECD Publishing, Paris, https://doi.org/10.1787/2247ce58-en.
[34] Moore, P. (2018), The Threat of Physical and Psychosocial Violence and Harassment in Digitalized Work, ILO, Geneva, https://www.ilo.org/wcmsp5/groups/public/---ed_dialogue/---actrav/documents/publication/wcms_617062.pdf.
[47] Morrison, S. (2020), “Just because you’re working from home doesn’t mean your boss isn’t watching you”, Vox, https://www.vox.com/recode/2020/4/2/21195584/coronavirus-remote-work-from-home-employee-monitoring (accessed on 29 March 2022).
[28] Nelson, B. (2018), “How robots are reshaping one of the dirtiest, most dangerous jobs”, NBC News, https://www.nbcnews.com/mach/science/how-robots-are-reshaping-one-dirtiest-most-dangerous-jobs-ncna866771 (accessed on 16 August 2021).
[22] Nguyen, A. and A. Mateescu (2019), Explainer: Algorithmic Management in the Workplace, Data & Society Research Institute, https://doi.org/10.1145/2702123.2702548.
[18] Noy, S. and W. Zhang (2023), “Experimental Evidence on the Productivity Effects of Generative Artificial Intelligence”, SSRN Electronic Journal, https://doi.org/10.2139/ssrn.4375283.
[1] OECD (2014), “How good is your job? Measuring and assessing job quality”, in OECD Employment Outlook 2014, OECD Publishing, Paris, https://doi.org/10.1787/empl_outlook-2014-6-en.
[42] OECD (2014), OECD Employment Outlook 2014, OECD Publishing, Paris, https://doi.org/10.1787/empl_outlook-2014-en.
[19] Peng, S. et al. (2023), “The Impact of AI on Developer Productivity: Evidence from GitHub Copilot”, https://arxiv.org/pdf/2302.06590.pdf.
[59] Quillian, L. et al. (2017), “Meta-analysis of field experiments shows no change in racial discrimination in hiring over time”, Proceedings of the National Academy of Sciences of the United States of America, Vol. 114/41, pp. 10870-10875, https://doi.org/10.1073/pnas.1706255114.
[40] Saint-Martin, A., H. Inanc and C. Prinz (2018), “Job Quality, Health and Productivity: An evidence-based framework for analysis”, OECD Social, Employment and Migration Working Papers, No. 221, OECD Publishing, Paris, https://doi.org/10.1787/a8c84d91-en.
[46] Sánchez-Monedero, J. and L. Dencik Sanchez-Monederoj (2019), “The datafication of the workplace”, Data Justice Project, European Research Council, https://datajusticeproject.net/wp-content/uploads/sites/30/2019/05/Report-The-datafication-of-the-workplace.pdf.
[50] Smith, P. and L. Smith (2021), “Artificial intelligence and disability: too much promise, yet too little substance?”, AI and Ethics, Vol. 1/1, pp. 81-86, https://doi.org/10.1007/S43681-020-00004-5.
[51] Touzet, C. (forthcoming), “Using AI to reduce the disability employment gap: Opportunities and challenges”, OECD Social, Employment and Migration Working Papers, OECD Publishing, Paris.
[24] Traeger, M. et al. (2020), “Vulnerable robots positively shape human conversational dynamics in a human-robot team”, PNAS, Vol. 117/12, pp. 6370-6375, https://doi.org/10.1073/pnas.19104021.
[52] Verhagen, A. (2021), “Opportunities and drawbacks of using artificial intelligence for training”, OECD Social, Employment and Migration Working Papers, No. 266, OECD Publishing, Paris, https://doi.org/10.1787/22729bd6-en.
[62] Wachter, S., B. Mittelstadt and C. Russell (2021), “Why Fairness Cannot Be Automated: Bridging the Gap Between EU Non-Discrimination Law and AI”, Computer Law & Security Review, Vol. 41, https://doi.org/10.2139/SSRN.3547922.
[65] Webb, M. (2020), “The Impact of Artificial Intelligence on the Labor Market”, Stanford University, https://www.michaelwebb.co/webb_ai.pdf.
[57] West, S., M. Whittaker and K. Crawford (2019), Discriminating Systems: Gender, Race and Power in AI, AI Now Institute, https://ainowinstitute.org/publication/discriminating-systems-gender-race-and-power-in-ai-2 (accessed on 21 October 2021).
[25] Wiggers, K. (2021), Computer vision-powered workplace safety systems could lead to bias and other harms, VentureBeat, https://venturebeat.com/2021/09/21/computer-vision-powered-workplace-safety-systems-could-lead-to-bias-and-other-harms/ (accessed on 4 November 2021).
[30] Wood, A. (2021), Algorithmic Management: Consequences for Work Organisation and Working Conditions, Joint Research Centre, European Commission, https://joint-research-centre.ec.europa.eu/publications/algorithmic-management-consequences-work-organisation-and-working-conditions_en.
[21] Yamamoto, I. (2019), The impact of AI and information technologies on worker stress, The Research Institute of Science and Technology for Society (RISTEX), Tokyo, Japan, https://cepr.org/voxeu/columns/impact-ai-and-information-technologies-worker-stress.
Notes
← 1. These new tasks and jobs are not limited to workers with AI skills and include the wider set of workers who are able to exploit the new technology, but who do not necessarily have AI skills.
← 2. See Chapter 3 for a more detailed summary, and Acemoglu and Restrepo (2018[2]) for the full discussion.
← 3. See Green and Lamby (2023[5]) for a discussion of the various approaches for identifying AI skills. Most studies use skill demands in job postings and classify postings as requiring AI skills if a posting demands skills in subfields of AI such as machine learning or natural language processing, or software packages frequently used by AI practitioners.
← 4. See Box 3.1 in Chapter 3 for more information.
← 5. Other examples of AI progress and exposure include Brynjolfsson, Mitchell and Rock, (2018[64]) who construct a suitability of machine learning index and Webb (2020[65]) who measures AI progress from patents.
← 6. This suggests that workers with AI skills may be driving the results, which clouds the interpretation for workers exposed to AI but lacking AI skills – see also Chapter 5.
← 7. Alekseeva et al., (2021[6]) similarly find that workers without AI skills but who work in firms with a higher share of workers with AI skills – a proxy for AI adoption – earn higher wages compared to similar workers.
← 8. Using a database of German firms with information on AI adoption in 2018, Czarnitzki, Fernández and Rammer (2022[67]) find evidence that AI increases sales, which they then interpret as productivity. They do not examine the impact on sales per employee as is typical in the literature. It is therefore difficult to interpret their results. However, they find a positive association between employment and sales which would suggest an attenuation of their measured productivity effect consistent with Babina et al. (2020[16]).
← 9. As with all studies of this kind, the results only account for workers who remain with the firm. If workers who find their performance decreases due to AI exit the firm, these responses would not be considered.
← 10. Several researchers, however, are questioning AI’s ability to accurately detect emotions and non-verbal cues across different cultures (Condie and Dayton, 2020[26]).
← 11. AI appears to have improved physical safety much less in finance, but this is not surprising as finance is not usually a physically demanding industry, nor is it known to be physically hazardous to one’s health. It may also be the case that workers whose health deteriorated due to AI were more likely to exit the firm.
← 12. In 2020, the Waste Management and Remediation Services industry had a fatal injury rate of 15 per 100 000 full-time‑equivalent workers, or about 5 times the average rate in the United States (BLS, 2021[66]).
← 13. One should interpret these results with caution, however, as workers who feel particularly stressed by a higher pace of work may have separated from the firm after the introduction of AI.
← 14. Forty-nine percent of workers in finance and 39% in manufacturing said that their company’s application of AI collected data on them as individuals or how they do their work.