This chapter covers MASK (Mobile Airways Sentinel Network), an mHealth app designed to reduce the burden of allergic rhinitis and asthma. The case study includes an assessment of MASK against the five best practice criteria, policy options to enhance performance and an assessment of its transferability to other OECD and EU27 countries.
Integrating Care to Prevent and Manage Chronic Diseases
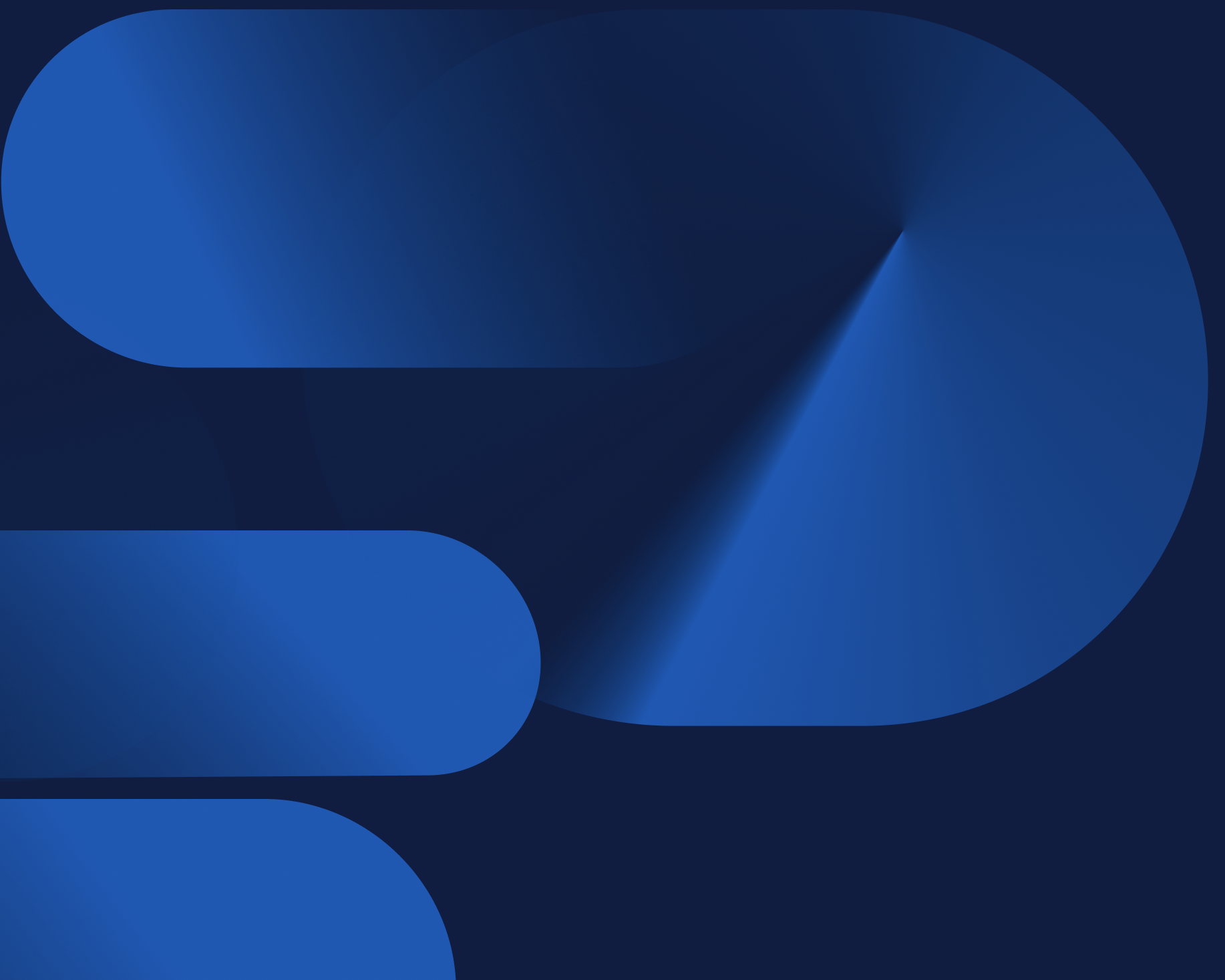
15. Mobile Airways Sentinel Network (MASK), mHealth app
Abstract
MASK (Mobile Airways Sentinel Network): Case study overview
Description: MASK (Mobile Airways Sentinel Network) is an mHealth intervention designed to reduce the burden of allergic rhinitis (AR) and asthma. MASK is broken into two components – one for individuals and the other for health professionals. Individuals can download the MASK-air app for free which includes an allergy diary (a daily recording of symptoms and treatment). Health professionals (GPs and specialists) have access to an MASK-air Companion, an electric decision support system to promote personalised treatment for patients with AR and asthma (i.e. patients share data from the MASK-air app with their health professional, which then informs treatment).
Best practice assessment:
OECD Best Practice assessment of MASK
Criteria |
Assessment |
---|---|
Effectiveness |
MASK has enhanced the knowledge base on AR and asthma, which has been used to improve treatment guidelines. Future evaluations of MASK aim to assess the impact of the intervention on work productivity, quality of life, adherence and symptoms |
Efficiency |
Real-world data (RWD) from the MASK-air app found that 97% of work days are impaired with uncontrolled AR symptoms compared to 20% when controlled An evaluation to estimate the cost per QALY of MASK is underway involving over 17 000 users. |
Equity |
MASK promotes equity by offering the same level of care for AR and asthma to people across the world, further, the intervention is designed to boost uptake amongst vulnerable populations (e.g. by making the app free of charge). However, mHealth apps can widen existing health inequalities given they are more popular among younger, higher-educated populations. |
Evidence‑base |
A mixture of prospective observational and cross-sectional studies were used to build the knowledge base on AR and asthma. For example, cross-sectional data from over 90 000 days was used to calculate the correlation between AR/asthma symptoms and work productivity. |
Extent of coverage |
MASK-air has been downloaded by over 40 000 people across 27 countries. This translates into a participation rate of around 0.1% based on high-level calculations. The proportion of physicians and pharmacists who use MASK in their practice is not known for legal reasons. |
Enhancement options: to enhance effectiveness, policy makers should continue efforts to boost levels of health literacy (HL) and digital HL, particularly amongst priority populations. Boosting digital HL amongst health professionals is also important to ensure they feel confident using digital products when treating patients. To enhance equity, MASK administrators could run promotion campaigns targeting priority populations. Further, to better understand the needs of priority population, new demographic questions could be added to the app allowing data (e.g. education as a proxy for socio‑economic status). To enhance the evidence‑base, an evaluation to determine the impact of MASK on outcomes such as quality of life is encouraged. To enhance the extent of coverage a multi-pronged targeted approach is needed.
Transferability: MASK was originally implemented in 18 countries and was subsequently been transferred to a further 10 (most of which are OECD and non-OECD European countries – e.g. Japan, Australia, Canada, Mexico, Brazil, Argentina). MASK is considered highly transferable given its simple design, further, experts from an international AR/asthma workgroup (ARIA) are located across the world who take responsibility for adapting the intervention to the local context.
Conclusion: MASK is an equity enhancing digital health intervention which has improved the knowledge base on AR and asthma. Subsequent evaluations of MASK using adherence, productivity and quality of life as outcomes, should be of key interest to policy makers and therefore strongly supported.
Intervention description
Allergic rhinitis (AR) and asthma are two of the most common chronic diseases in the world. Asthma affects approximately 339 million people worldwide and is attributable to an estimated 417 000 deaths and 24.8 million disability-adjusted life years (DALYs) annually (WHO, 2020[]).
Despite high levels of AR and asthma, self-management of AR and asthma, as reflected by adherence to medication, is poor. Further, shared decision-making is limited between patients with AR and asthma and health professionals.
To improve self-management of AR and asthma, there has been a growing shift towards mHealth apps. Digital innovations, such as mHealth, are growing increasingly popular given their potential to simultaneously improve patient outcomes and reduce pressure on healthcare systems. The growing role of mHealth apps is reflected at the international policy level, for example, the WHO in 2012 created the “Be He@lthy, Be Mobile” initiative, which produced a handbook on how to implement mHealth apps directly targeting asthma and COPD (WHO and ITU, 2017[]). MASK is one of the examples presented in this document.
MASK (Mobile Airways Sentinel Network) is a digital intervention designed to reduce the burden of AR and asthma. The MASK intervention is broken into two components – a component for individuals and another for health professionals.
MASK is the IT tool of the ARIA (Allergic Rhinitis and its Impact on Asthma) initiative developed from a WHO workshop in 1999. It has been further deployed to 52 countries by the WHO Collaborating Centre on asthma and rhinitis, Montpellier (2004‑14). The third revision of ARIA guidelines (2017) 1 has been taken as the case scenario of the users’ guides to the medical literature on how to interpret and use a clinical practice guideline or recommendation recently published in the JAMA.
MASK for individuals
The MASK-air mHealth app is an online allergy diary designed for individuals with AR or asthma and is the central component of the MASK intervention (Bousquet et al., 2020[]). The app encourages users to record their symptoms by answering a range of questions daily. Specifically, users are prompted to answer questions related to:
Allergy symptoms: rate nose, eye, asthma, and overall allergy symptoms using a visual analogue scale (VAS) (i.e. from “not at all bothersome” to “extremely bothersome”)
Work: work today (yes/no) and if yes how allergies affected productivity (VAS from “not at all bothersome” to “no work possible”)
Education: attend classes in an academic setting (yes/no) and if yes how allergies affected productivity
Treatment: note down the treatments used that day (the app includes a full list of over-the‑counter (OTC) and prescribed medications specific to each country).
Users of the app can “go further” and answer additional questionnaires, although not on a daily basis – for example, the CARAT (Control of Allergic Rhinitis and Asthma Test) and the EQ‑5D. The former is a validated instrument to summarise the clinical status of AR and asthma in the previous month (users are prompted to answer the questionnaire after the first app use), while the latter is a commonly applied questionnaire to assess health-related quality of life.
A new pollen feature was uploaded to the app early 2021. The new feature describes the level of pollen exposure in the user’s local area allowing for an “easy and fast documentation of pollen allergy counts”. Users can therefore plan daily outdoor activities to minimise pollen exposure (Bousquet et al., 2019[]).
MASK is a Class 2 Medical Device therefore it is possible to upload information from the app to the user’s electronic health record (EHR).
The app is available in 28 countries (most of which are OECD countries) and 18 languages, and is free of charge. In May 2021, the International Pharmaceutical Federation, the global body representing pharmacy, and pharmaceutical sciences and education, agreed to join MASK.
MASK for health professionals
Physicians and pharmacists will have access to the digital tool, the MASK-air Companion, via their tablet and a physician web-based questionnaire. These tools are inter-operable with the app. The Companion is an electronic decision support system to assist health professionals diagnose and provide personalised treatment to patients. Based on this information, health professionals can work with patients to develop tailored treatment using guidelines embedded within MASK.
OECD Best Practices Framework assessment
This section analyses MASK against the five criteria within OECD’s Best Practice Identification Framework – Effectiveness, Efficiency, Equity, Evidence‑base and Extent of coverage (see Box 15.1 for a high-level assessment of MASK). Further details on the OECD Framework can be found in Annex A.
Box 15.1. Assessment of MASK e‑platform, multiple countries
Effectiveness
MASK has enhanced the knowledge base of on AR and asthma, which has been used to improve treatment guidelines
Future evaluations of MASK will assess the impact of the intervention on work productivity, quality of life, adherence and symptoms
The impact of mHealth apps for AR and asthma on final outcomes (e.g. quality of life) is limited in the broader literature
Efficiency
Real-world data (RWD) from the MASK-air app detail the extent to which AR and asthma symptoms affect productivity
An evaluation to estimate the cost per QALY of MASK is underway involving over 17 000 users
Equity
MASK promotes equity by offering the same level of care for AR and asthma to people across the world, further, the intervention has been designed to boost uptake amongst vulnerable populations (e.g. by making the app free of charge).
Conversely, mHealth apps, such as MASK, have the potential to widen existing health inequalities given they more popular amongst younger, higher educated populations.
Evidence‑base
A mixture of prospective observational and cross-sectional studies were used to build the knowledge base on AR and asthma. For example, cross-sectional data from thousands of participants was used to calculate the correlation between AR/asthma symptoms and work productivity.
Extent of coverage
The MASK-air app has been downloaded by over 40 000 people across 28 countries. This translates into a participation rate of around 0.1% based on high-level calculations.
The proportion of physicians and specialists who use MASK is not known for legal reasons
Effectiveness
The effectiveness criterion reflects whether outcomes (final or intermediate) of the intervention were achieved. Final outcomes reflect the ultimate objective of policy makers, for this reason, they can take many years to achieve. In the interim, intermediate outcomes are collected which directly relate to final outcomes.
Intermediate and final outcomes of interest to the MASK intervention are summarised in Box 15.2. The remainder of this section explores MASK’s progress towards achieving these outcomes, with a specific focus on intermediate outcomes given this is where most progress has been made.
Box 15.2. MASK intervention intermediate and final outcomes
Intermediate outcomes
Improved treatment for people with AR and asthma through:
Better knowledge of symptoms and treatments
Better self-management of AR and asthma / patient empowerment
Development of personalised treatment plans (shared decision-making)
Final outcomes
Reduce AR and asthma symptoms
Improve patient quality of life
Improve work productivity and indirect costs
Reduce pressure on healthcare systems
Intermediate outcomes
To date, the main achievement of MASK has been its contribution towards enhancing the knowledge base on AR and asthma using real-world data (RWD).
Better knowledge of symptoms and treatments: the impact allergic airway diseases have on multimorbidity is well established, however, until recently less was known on the dynamics of daily symptoms (Sousa‐Pinto et al., 2022[]). The MASK-air allergy diary collects daily data on symptoms and is therefore well-placed to address this knowledge gap. For example, a one‑year prospective observational study using symptom data from 4 210 users (and 32 585 days) discovered considerable intra‑individual variability of allergic multimorbidity, including a previously unrecognised extreme pattern of uncontrolled multimorbidity (MACVIA working group, 2018[]).
In terms of treatments, two cross-sectional studies using MASK-air data confirmed hypotheses that people do not use treatment on a daily basis, rather, they increase treatment with the onset of symptoms – i.e. VAS scores (which reflect symptom severity) were higher on days when patients used treatment (Bousquet et al., 2018[]) (Bédard et al., 2019[]). This finding led to a change in treatment guidelines for AR and asthma patients.
The novel discoveries using MASK-air data were confirmed in epidemiologic studies and genomic studies (Lemonnier et al., 2020[]). Example discoveries include knowledge that:
Eye symptoms are more common in polysensitised patients (i.e. those who are sensitive to more than one allergen family), regardless of whether they have asthma (Siroux et al., 2019[])
Eye symptoms are positively associated with the severity of nasal symptoms and predict severe asthma (Amaral et al., 2018[]; Raciborski et al., 2019[])
The severity of allergic diseases increases with the number of allergic multimorbidities (Amaral et al., 2018[])
Some medications work better when taken simultaneously (Toppila‐Salmi et al., 2019[]).
A better understanding of symptoms and treatments allows health professionals to provide more appropriate care to patients. The direct impact this has had on final patient outcomes is not yet known.
Better self-management of AR and asthma: a cross-sectional, observational study with approximately 6 000 MASK-air users found adherence to pharmacological treatment is approximately 10% (The MASK Group, 2019[]). Data linking the impact of MASK-air on adherence to medication is not available. More recently, data from the MASK-air app was used to assess adherence in patient reporting inhaled corticosteroid (ICS) and long-acting ß2‑agonist (ICS+LABA) use. The analysis of the data found 30% of patients treated by inhaled steroids have an adherence rate between 60‑70% depending on the medication (Sousa-Pinto et al., forthcoming[]).
Development of personalised treatment plans: next-generation guidelines to treat patients with AR were based on RWD from MASK-air in addition to existing GRADE‑based guidelines as well as data from randomised controlled trials. Specifically, these information sources were used to develop the MACVIA (Contre les Maladies Chroniques pour un Vleillissement Actif) algorithm for AR treatment (MACVIA is one of the implementation tools of the European Innovation Partnership on Active and Healthy Ageing). The algorithm is built into the electronic decision support system for health professionals – the Allergy Diary Companion – therefore, health professionals will be able to prescribe personalised treatment based on patient-specific data. For example, the algorithm recommends treatments based on VAS scores (i.e. symptom severity), current medications and patient preferences (Bousquet et al., 2020[]) (see Annex 15.A). Since the upgrade of MASK to a C2MD, health professionals have had access to these guidelines in their Allergy Diary Companion.
Development of symptom medication scores (SMSs). SMSs are needed to investigate effect sizes of allergic rhinitis (AR) treatments. A combined symptom-medication score (CSMS) for allergic rhinitis (ARIA-EAACI Task Force) has been designed from MASK RWD. This approach is unique and allows for a standardisation of randomised controlled trials, RWD and clinical practice. In a 2021 paper, CSMS data from the MASK air app was found to be valid, reliable and accurate, therefore, it can be used as a primary endpoint for future rhinitis trials (Sousa‐Pinto et al., 2022[]). Further, a daily electronic symptom medication score for asthma has been recently validated (Sousa-Pinto et al., 2023[]).
Deployment to asthma phenotypes: Eight novel phenotypes of asthma have been identified in 8 000 users of MASK-air. A new asthma-resistant phenotype associates uncontrolled asthma despite treatment and uncontrolled rhinitis and conjunctivitis (Bousquet et al., 2022[]).
Final outcomes
The impact of MASK final outcomes is not yet known given, to date, the focus has been to build the knowledge base on AR and asthma. The impact of similar mHealth apps on final outcomes is limited in the literature (WHO and ITU, 2017[]). For example, a systematic review undertaken by the WHO identified just two examples of where mHealth apps improved outcomes for users with asthma (both of which were in the United States):
Farooqui et al. (2015[]) evaluated an asthma management mHealth app for children and adolescents and found it improved measures by users to avoid asthma triggers
Britto et al. (2017[]) evaluated an intervention that used text messages to improve outcomes and found it led to modest improvements in asthma control, adherence to treatment and quality of life.
Efficiency
Given the relatively low cost of mHealth interventions and the high societal costs of AR and asthma, MASK has the potential to be highly efficient. Previous economic evaluations conclude productivity losses are the primary cost associated with AR (i.e. impaired performance and presenteeism) (Bousquet et al., 2018[]). For example, a systematic review on the impact of rhinitis on work productivity found that 3.6% of people with AR missed work time while symptoms impaired work performance for 35.9% of people (Vandenplas et al., 2018[]). In Europe work productivity losses due to AR are estimated to cost between EUR 30‑50 billion per year (Zuberbier et al., 2014[]).
Data from the MASK-air app support previous findings on the economic impact of AR. A cross-sectional study using data from MASK-air (n=1 136 users over 5 659 days) assessed the impact of work productivity on uncontrolled AR. Results from the analysis found that when AR symptoms are controlled, work impairment occurs in less than 20% of all days compared to over 90% when symptoms are not controlled (Bousquet et al., 2017[]). The same study also measured the correlation between work productivity and specific AR symptoms. Results from the analysis found positive, strong correlations between work impairment and rhinitis (r=0.73) and asthma symptoms in users with asthma (r=0.60) (Bousquet et al., 2017[]). A study comparing the costs of MASK (see Box 15.3) to the impact it has on quality of life is underway involving nearly 18 000 users. Results from Bousquet et al. (2017[]) are supported by a more recent study involving over 7 000 users (The MASK Study Group, 2020[]).
Box 15.3. Cost of developing and implementing MASK
The cost of implementing MASK between 2014‑20 was EUR 6.04 million, which was funded primarily through EU grants and private funding (Figure 15.1). The estimated cost for adding a country that wishes to transfer MASK is EUR 19 000 (or EUR 26 000 if MASK is not available in the local language). This includes various services such as project supervision, regulatory verification, terms of use translation, and app store implementation. For the full price list, see Annex 15.B.
Figure 15.1. Cost of developing and implementing MASK
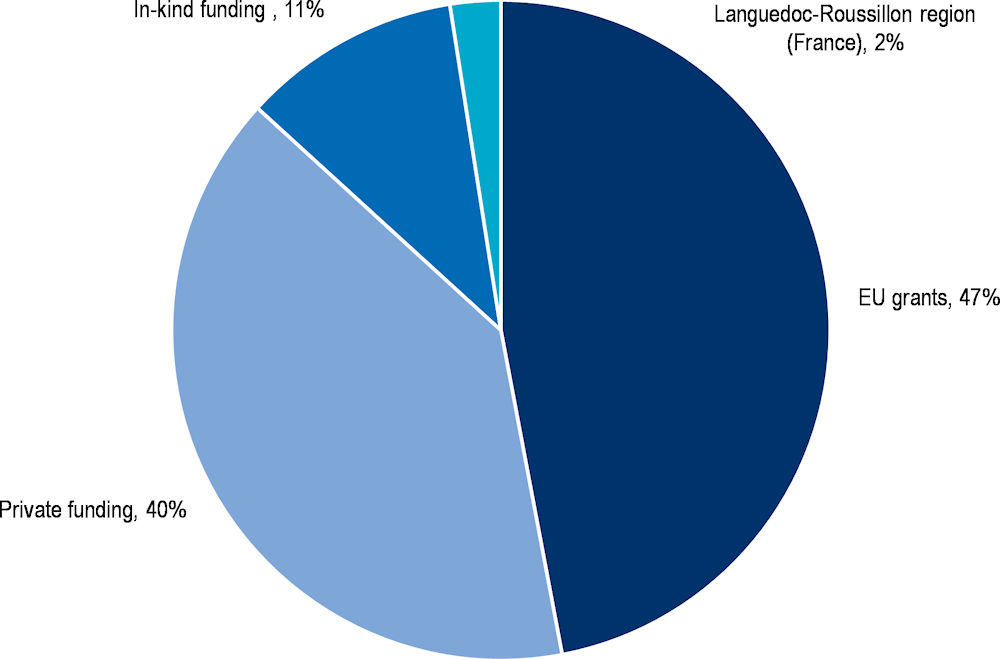
Source: Data provided by MASK administrators.
Equity
MASK promotes equity by offering the same level of care to people across the world, further, the intervention has been designed to boost uptake amongst vulnerable populations. MASK is a demonstration project part of the WHO Global Alliance against Chronic Respiratory Diseases (GARD), which places a strong emphasis on ensuring equity of access to healthcare. MASK promotes equity by offering people across the world (including those in low- to high-income countries) access to the same quality of care for treating patients with AR and asthma (i.e. the clinical guidelines are the same across the world). Further, the app has been designed to accommodate the needs of certain priority populations by making it easy-to‑use and free of charge.
In the region of Puglia, Italy, a specific effort to engage older people in the app is underway. The region’s “Allergy Unit”, which is dedicated to older patients, has invested in boosting health literacy levels. Initial research into the uptake of the MASK-air is promising with 60% of older age people stating they have the skills to use the app.
Despite efforts to boost uptake amongst priority populations, mHealth apps have the potential to widen existing health inequalities. Digital health interventions such as mHealth apps are more popular amongst younger, higher educated populations (Bol, Helberger and Weert, 2018[]; Azzopardi-Muscat and Sørensen, 2019[]). For example, research undertaken by OECD estimated adults in the highest income quartile are 50% more likely to use the internet to research health information, compared to adults in the lowest income quartile (OECD, 2019[]). Other groups less likely to use digital health interventions include older populations and those living in rural areas due to factors such as cost, lower digital health literacy skills and limited broadband access (Bol, Helberger and Weert, 2018[]; Azzopardi-Muscat and Sørensen, 2019[]; Oliveira Hashiguchi, 2020[])
Evidence‑base
Evidence to assess the effectiveness and efficiency of MASK relates to the intervention’s contribution towards enhancing the knowledge base on AR and asthma. Therefore, it is not appropriate to assess the evidence‑base of MASK using the Quality Assessment Tool for Quantitative Studies from the Effective Public Health Practice Project. Instead, this section summarises the methodology for a selection of articles cited under the section assessing the ‘Effectiveness’ and ‘Efficiency’ of MASK (see Box 15.4).
Box 15.4. Quality of evidence supporting MASK
A description of the methodology for a selection of studies outlined in the sections on “Effectiveness” and “Efficiency” are summarised below.
Better knowledge of symptoms and treatments
MASK-air data was used to assess how people with AR and asthma treat themselves (i.e. patient behaviour). To undertake this analysis, a prospective observational study using MASK-air data was used. All users who were registered between May 2015 and November 2018 were included (no inclusion criteria) in order to maximise the sample size (n=9 122 users across 22 countries). Given an insufficient number of users reporting data over five days, a longitudinal analysis was not possible, instead non-parametric tests and medians were used to undertake the analysis (Bousquet et al., 2018[]).
A similar methodology using data from over 9 000 users across 122 054 days was used by Bèdard et al. (2019[]) to assess how people treat themselves.
A 2022 longitudinal study covering over 16 000 weeks of data collected from the MASK-air app concluded that patients were treating themselves according to their symptoms (Sousa‐Pinto et al., 2022[]).
Self-management of AR and asthma
Adherence to medication for users of MASK was assessed using a cross-sectional study over a period of 20 months. Users were included in the study if they recorded symptoms for at least seven days (not necessarily consecutively) within the MASK-air app. Data from 1 195 users across 22 countries were included in the study. Secondary adherence was measured using the Medication Possession Ratio (MPR) (ratio of days that medication was reported to have been used over a set period of time) and the Proportion of Days Covered (PDC) (days of reporting medication divided by the time interval defined by the first and last day the app was used) (The MASK Group, 2019[]).
Adherence was also assessed in a forthcoming paper by Sousa-Pinto et al. (forthcoming[]). The analysis relied on real-world data collected from 100s of patients over several hundreds of weeks.
Impact of AR on work productivity
The impact of AR on work productivity was analysed using a cross-sectional study using data from the MASK-air app. Data was collected from (over 7 000 users, 98 303 days) across 25 countries over the period 1 June 2016 to 31 October 2018. Correlations between symptoms and work productivity were calculated using Spearman’s Rank test.
A similar methodology was used by Bèdard et al. (2020[]) involving over 14 000 users across nearly 206 000 days.
Extent of coverage
Individuals
As of December 2020, the MASK-air app had been downloaded by over 40 000 people across 27 countries. Of these users, 45% are aged 16‑90 years and regularly use the app by logging their symptoms via the VAS questions (Figure 15.2). The total number of users is highest in Italy (1 786), however, prolonged use is highest in Mexico where over 700 people have used the app for 14 days or more. On a daily basis, MASK-air has approximately 1 000 users, which increases during the pollen season.
The participation rate (the proportion of the eligible population who access an intervention) in MASK-air ranges from 0.004% in Canada to 1.22% in Lithuania, based on high-level calculations1 (average 0.11% across all countries) (OECD.Stat, 2018[]; World Bank, 2019[]; Institute for Health Metrics and Evaluation, 2019[]). This level of participation aligns with existing information on mHealth uptake, for example, previous research by OECD revealed around 2.2% of the adult population (15‑64 years) use mobile apps to improve their health and fitness (Goryakin et al., 2017[]; OECD, 2019[]).
Figure 15.2. MASK-air users by days of use
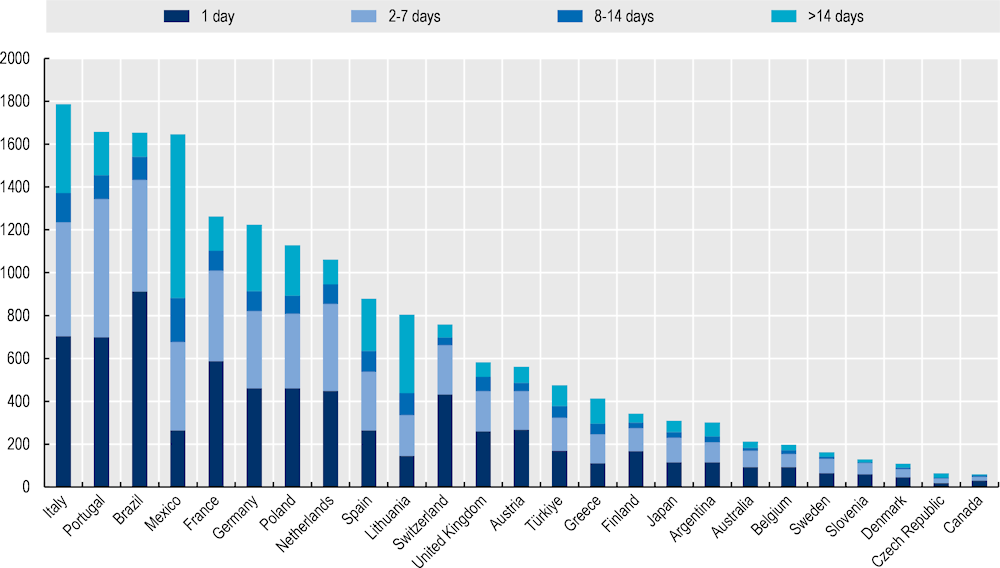
Source: Data provided to OECD by MASK-air administrators.
Health professionals
The number of physicians and pharmacists who use MASK is not known due to privacy laws. This information will become available when MASK is upgraded to a C2MD.
Policy options to enhance performance
Policy options available to high-level policy makers (e.g. region / state / national governments) and MASK administrators are outlined in this section and refer to each of the five best practice criteria.
Enhancing effectiveness
Higher levels of population health literacy (HL) will enhance the effectiveness of mHealth apps such as MASK-air. HL refers to an “individual’s knowledge, motivation and skills to access, understand, evaluate and apply health information” (OECD, 2018[]). When people are health literate they are more likely to act on health information they receive, take greater responsibility for their own health (e.g. by adhering to medication), as well as engage in shared decision-making. Recent analysis estimated that more than half of OECD countries with available data had low levels of HL (OECD, 2018[]). To address low rates of adult health literacy, OECD have outlined a four‑pronged policy approach (OECD, 2018[]):
Strengthen the health system role: establish national strategies and framework designed to address HL
Acknowledge the importance of HL through research: measure and monitor the progress of HL interventions to better understand what policies work
Improve data infrastructure: improve international comparisons of HL as well as monitoring HL levels over time
Strengthen international collaboration: share best practice interventions to boost HL across countries.
In addition to the above, high-level policy makers could consider actions directly targeting individuals, for example, encouraging HL at schools, and providing HL counselling and training in community and workplace settings. Enhanced HL will also increase uptake (i.e. extent of coverage) of MASK-air.
Digital health products, such as MASK, require users to be digitally health literate. Healthcare systems are growing increasingly digital as evidenced by the growing number of countries with national eHealth strategies (WHO, 2015[]). Therefore, in addition to improving HL, policy makers should promote digital HL so that people can apply their health knowledge/skills to digital products. Any policy efforts should have a specific focus on groups of the population who face barriers to accessing and utilising eHealth products, such as mHealth apps, given these groups often stand to benefit most (e.g. those with a lower socio-economic status) (Oliveira Hashiguchi, 2020[]).
Health professionals must also be digitally health literate in order to feel confident using digital products when treating patients. Among OECD countries, one‑third of health workers do not feel accustomed to using digital solutions “due to gaps in knowledge and skills in data analytics” (OECD, 2019[]). To ensure health professionals can “safely and effectively” adopt digital work tools (e.g. mHealth apps), it is important they receive adequate support via training and education, for example by (OECD, 2019[]):
Developing digital health competency frameworks that inform what changes to the education of health professionals are needed, with a particular focus on physicians. For example, the EU*US eHealth Work Project (2016‑18) developed an international competency framework and aligning education content to enhance the digital skills of health professionals (EU*US eHealth Work Project, 2019[]).
Developing concrete guidelines on how to integrate digital health topics into education and training programs for health professionals, for example, as done by the Swiss Competence and Co‑ordination Centre of the Confederation and the Cantons.
Integrating digital skills into Continuous Professional Development (CPD) programs, to ensure health professional skills align with latest digital developments.
Enhancing efficiency
Efficiency is calculated by obtaining information on effectiveness and expressing it in relation to inputs used. Therefore, policies to boost effectiveness without significant increases in costs will have a positive impact on efficiency.
Enhancing equity
Policies to increase access and utilisation of MASK among priority population groups can reduce health inequalities. There are groups in the population who are less likely to utilise and therefore benefit from digital health products, such as MASK-air – e.g. the older population are less likely to be digitally health literate, while economically disadvantaged groups may not have regular access to the internet (Bol, Helberger and Weert, 2018[]; Azzopardi-Muscat and Sørensen, 2019[]; Oliveira Hashiguchi, 2020[]). Governments and other relevant policy makers can respond by focusing efforts to build HL and digital HL on priority population groups. More direct action that can be implemented by MASK administrators include:
targeted promotion campaigns as well as the provision of detailed, tailored, advice on how to use the app and its benefits
collecting data that can be disaggregated by priority population groups (e.g. information on level of education as a proxy for SES status). This information can subsequently be used to amend MASK to suit the needs of priority populations.
Failing to address the needs of priority population groups risks widening existing health inequalities.
Enhancing the evidence‑base
The impact of MASK on final outcomes will be of key interest to policy makers and is therefore encouraged. To date, studies evaluating the impact of MASK have focused on the intervention’s impact on building the knowledge base around AR and asthma. Going forward, an evaluation to understand the impact of MASK on final outcomes (e.g. patient quality of life, work productivity and health system costs) is encouraged given this of key interest to policy makers.
Key steps involved in undertaking an evaluation are outlined in OECD’s Guidebook on Best Practices in Public Health. These steps are summarised below to assist MASK administrators in future evaluation efforts:
Design the evaluation study
Develop a logic model: a logic model summarises the main elements of an intervention and provides a visual overview of the relationship between inputs, activities, outputs and outcomes. Example programme logics for mHealth apps can be found in WHO’s Be He@lthy, Be Mobile handbook for asthma and COPD (WHO and ITU, 2017[]).
Select evaluation indicators: indicators for each element within the programme logic need to be specified. Example outcome indicators for MASK may include EQ‑5D (patient quality of life) and work productivity. Indicators should be SMART (specific, measurable, achievable, relevant and time‑bound) and where possible be stratified to understand the intervention’s impact on inequalities (as discussed under “Enhancing efficiency”).
Choose a study design: process evaluations assess whether an intervention was implemented as intended whereas an outcome evaluation assesses the impact the intervention had on outcomes. Regarding the latter, it is necessary to choose a study design that is appropriate for the intervention.
Choose a data collection method: any evaluation of MASK will largely rely on real-world data collected from the app. Additional primary sources of data may also be collected, for example, from user surveys.
Execute the evaluation study
Collect the data: data collection methods should consider logistics, consent, privacy, data security and other ethical considerations. Given data from MASK has been used in numerous studies, no significant barriers are foreseen. In regards to timeline, data is typically collected at the start, middle and end of an intervention. This is less relevant for MASK given it has already been implemented and is ongoing. Nevertheless, it may be useful when evaluating the impact of MASK in a new country.
Analyse the data: it is not possible to detail all the various methods available to analyse data here, however, a first step for any intervention is to analyse descriptive statistics including a look at the pattern of missing data.
Act on evaluation results
Follow-up action: results from the evaluation will provide useful information on how the intervention can be adapted to improve performance.
Disseminate results: evaluation results should be conveyed to the target audience via appropriate channels. In particular, it is important to convey “lessons learnt” and how these will be incorporated into the future design of MASK.
Enhancing extent of coverage
A multi-pronged approach is needed to boost uptake of MASK-air among the public. Several strategies exist to boost uptake of mHealth apps amongst the public. For example, ensuring the app is easy-to‑use, free‑of-charge and safe (i.e. privacy is ensured), all of which are characteristics of MASK-air. More specific strategies that are relevant to MASK-air are outlined in WHO’s Be He@lthy, Be Mobile: a handbook on how to implement mHealth for asthma and COPD (see Box 15.5) (WHO and ITU, 2017[]).
Box 15.5. Promotion and recruitment for asthma and COPD mHealth apps
WHO has developed a “Be He@lthy, Be Mobile” handbook on how to implement mHealth for asthma and COPD. Within the handbook there are several recommendations on how to optimise an mHealth’s promotion and recruitment strategy, specifically by considering (WHO and ITU, 2017[]):
The target audience and the most effective method for reaching them
Who the public view is the “owner” of the intervention as this will guide the promotional campaign
Whether the promotional campaign can be linked to or leveraged by organisations or public figures with a high media profile related to respiratory disease control or prevention
Previous lessons learnt in terms of effective promotional techniques
The local mobile network environment (e.g. can unsolicited text messages be sent to those with mobile phones?)
Whether incentives are appropriate to boost uptake (preferably non-monetary)
Whether marketing and promotional campaigns can be leveraged by stakeholder agencies
The timing of the campaign, for example, by first running a “soft launch” to address any unforeseen issues.
To increase uptake amongst health professionals, MASK must continue to be trusted and non-burdensome. Several strategies exist to boost uptake of digital products amongst health workers. Salient examples include (OECD, 2019[]):
ensuring the digital product is developed based on robust evidence in order to build trust
involving health professionals (i.e. end-users) in the design of the digital product given they are best placed to understand patient needs and wants
ensuring the digital product is easy-to‑use and can be integrated into current practices given health professionals are often under significant time pressure.
The above examples are current features of the MASK intervention. Nevertheless, before implementing any new features (e.g. updates to the app), the needs of health professionals should be taken into account.
Transferability
This section explores the transferability of MASK and is broken into three components: 1) an examination of previous transfers; 2) a transferability assessment using publicly available data; and 3) additional considerations for policy makers interested in transferring MASK.
Previous transfers
MASK was initially deployed across 18 countries and has been transferred to a further 10. The majority of MASK countries are also OECD members (see Table 15.1). The intervention will continue to be scaled-up across Europe as part of the European Innovation Partnership on Active and Healthy Ageing (EIP-AHA) Twinning Project (see Box 15.6) and globally by the ARIA group and the Global Alliance Against Chronic Respiratory Diseases (GARD), a WHO alliance.
Box 15.6. EIP-AHA Twinning Project
As part of the EIP-AHA Twinning Project, the MASK-air app is being transferred to 25 reference sites. A reference site may be a country, region or organisation. The purpose of the Twinning Project is to assess the transferability of MASK-air to a range of different contexts and to better understand the burden, diagnosis and management of rhinitis (Bousquet et al., 2019[]).
The core components of MASK-air are the same across all countries, however, the app is adapted to suit the needs of each country. MASK relies on its experts from the ARIA (Allergic Rhinitis and Its Impact on Asthma)2 workgroup to adapt the intervention – at present there are nearly 700 ARIA experts across 92 countries. Experts are responsible for translating the app into the local language, wording questions appropriately and adapting the medication list to align with available over-the‑counter medicines.
Table 15.1. Countries where MASK is available
Country group |
Countries |
---|---|
OECD |
Argentina, Austria, Australia, Brazil, Belgium, Canada, the Czech Republic, Germany, Denmark, Spain, Finland, France, Japan, Greece, Hungary, Italy, Lithuania, Mexico, the Netherlands, Poland, Portugal, Slovenia, Sweden, Switzerland, Türkiye, the United Kingdom Implementation underway in: Romania |
Other |
Lebanon |
Transferability assessment
Methodological framework
Details on the methodological framework to assess transferability can be found in Annex A.
Several indicators to assess the transferability of MASK were identified (Table 15.2). Indicators were drawn from international databases and surveys to maximise coverage across OECD and non-OECD European countries. Please note, the assessment is intentionally high level given the availability of public data covering OECD and non-OECD European countries.
MASK is available in most OECD countries (Table 15.1), therefore results from the transferability assessment can instead be used to identify areas to enhance the impact of MASK.
Table 15.2. Indicators to assess the transferability of MASK
Indicator |
Reasoning |
Interpretation |
---|---|---|
Population context |
||
% of individuals using the internet for seeking health information in the last 3 months |
MASK is more likely to be successful in a population comfortable seeking health information online |
🡹 value = more transferable |
ICT Development Index* |
MASK is more likely to be successful in digitally advanced countries |
🡹 value = more transferable |
Sector context (digital health sector) |
||
Legislation exists to protect the privacy of personally identifiable data of individuals, irrespective of whether it is in paper or digital format |
mHealth apps work in settings where users feel their personal data is safe. Therefore, MASK is more transferable to countries with legislation to protect patient data. |
‘Yes’ = more transferable |
eHealth composite index of adoption amongst GPs** |
MASK users can share results from the app with their GP, therefore, MASK is more transferable to countries where GPs are comfortable using eHealth technologies |
🡹 value = more transferable |
Entity providing incentives and guidance for mHealth apps |
MASK is more likely to be successful in countries where there is an entity responsible for promoting mHealth apps |
‘Yes’ = more transferable |
mHealth programs for clinical decision support are available |
MASK is more transferable to countries where health professionals utilise mHealth apps to support clinical decision making |
‘Yes’ = more transferable |
Political context |
||
A national eHealth policy or strategy exists |
MASK is more transferable to countries with national policies to support eHealth |
‘Yes’ = more transferable |
A dedicated national telehealth policy or strategy exists |
MASK is more transferable to countries with national policies to support telehealth |
‘Yes’ = more transferable |
Economic context |
||
Special funding is allocated for the implementation of the national eHealth policy or strategy |
TEC is more likely to be successful if there already is allocated funding for eHealth |
‘Yes’ = more transferable |
Note: *The ICT development index represents a country’s information and communication technology capability. It is a composite indicator reflecting ICT readiness, intensity and impact (ITU, 2020[]). **The eHealth composite index of adoption amongst GPs is made up of adoption in regards to electronic health records, telehealth, personal health records and health information exchange (European Commission, 2018[]).
Source: ITU (2020[]), “The ICT Development Index (IDI): conceptual framework and methodology”, https://www.itu.int/en/ITU-D/Statistics/Pages/publications/mis/methodology.aspx; WHO (2015[]), “Atlas of eHealth country profiles: The use of eHealth in support of universal health coverage”, https://www.afro.who.int/publications/atlas-ehealth-country-profiles-use-ehealth-support-universal-health-coverage; European Commission (2018[]), “Benchmarking Deployment of eHealth among General Practitioners (2018)”, https://op.europa.eu/en/publication-detail/-/publication/d1286ce7-5c05-11e9-9c52-01aa75ed71a1; OECD (2019[]), “Individuals using the Internet for seeking health information – last 3 m (%) (all individuals aged 16‑74)”; WHO, “Existence of operational policy/strategy/action plan to reduce unhealthy diet related to NCDs (Noncommunicable diseases)”, https://apps.who.int/gho/data/node.imr.NCD_CCS_DietPlan?lang=en; World Bank (2017[]), “GNI per capita, PPP (constant 2017 international $)”, https://data.worldbank.org/indicator/NY.GNP.PCAP.PP.KD.
Results
Sweden has been chosen as the “owner setting” given it scores well against criteria relevant for assessing the transferability of MASK (i.e. contextual factors in the country are conducive to the success of MASK) (Table 15.3). For example, Sweden has good information and communication technology capability (ICT score of 8.5 versus 7.4 average across all countries). Given MASK already operates in the majority of countries analysed, findings from the assessment can inform countries on areas to improve to enhance the impact of MASK.
Key areas countries could improve include boosting eHealth adoption amongst health professionals (such as GPs) (see section “Enhancing effectiveness”); compared to Sweden, eHealth adoption is approximately 20% lower in countries with available data. Improving levels of digital HL in the public is also important as it increases the proportion of people seeking health information online (currently 62% of people in Sweden seek health information online versus 54% on average amongst other countries).
Table 15.3. Transferability assessment by country (OECD and non-OECD European countries) – MASK
A darker shade indicates MASK may operate better in that particular country
Country |
Individuals using the Internet for seeking health information – last 3 m (%) |
ICT Development Index (2015) |
Legislation exists to protect the privacy of personally identifiable data of individuals, irrespective of whether it is in paper or digital format |
eHealth composite index of adoption score amongst GPs in Europe |
An entity providing incentives and guidance for innovation, research and evaluation of health apps exists |
mHealth programmes that provide clinical decision support (operating levels and types) |
A national eHealth policy or strategy exists |
A dedicated national telehealth policy or strategy exists |
The proportion of funding contribution for eHealth programmes provided by public funding sources over the previous two years |
---|---|---|---|---|---|---|---|---|---|
Sweden |
62 |
8.50 |
Yes |
2.52 |
Yes |
National |
Yes |
No |
Very high |
Australia† |
42 |
8.20 |
n/a |
n/a |
n/a |
n/a |
Yes |
No |
Very high |
Austria† |
53 |
7.50 |
Yes |
1.91 |
No |
n/a |
No |
No |
Very high |
Belgium† |
49 |
7.70 |
Yes |
2.07 |
No |
National |
Yes |
Combined* |
Very high |
Bulgaria |
34 |
6.40 |
Yes |
1.81 |
No |
n/a |
Yes |
Combined |
Low |
Canada† |
59 |
7.60 |
Yes |
n/a |
n/a |
National, local |
Yes |
No |
Very high |
Chile |
27 |
6.10 |
Yes |
n/a |
n/a |
n/a |
Yes |
No |
Very high |
Colombia |
41 |
5.00 |
Yes |
n/a |
n/a |
National, intermediate |
n/a |
Yes |
n/a |
Costa Rica |
44 |
6.00 |
Yes |
n/a |
n/a |
n/a |
Yes |
Yes |
Very high |
Croatia |
53 |
6.80 |
Yes |
2.18 |
Yes |
n/a |
Yes |
Yes |
Very high |
Cyprus |
58 |
6.30 |
1.93 |
No |
n/a |
Yes |
Combined |
Very high |
|
Czech Republic† |
56 |
7.20 |
Yes |
2.06 |
Yes |
n/a |
No |
Combined |
Low |
Denmark† |
67 |
8.80 |
Yes |
2.86 |
No |
Regional |
Yes |
Yes |
Very high |
Estonia |
60 |
8.00 |
Yes |
2.79 |
Yes |
Intermediate |
Yes |
No |
Very high |
Finland† |
76 |
8.10 |
Yes |
2.64 |
n/a |
Regional |
Yes |
Combined |
Very high |
France† |
50 |
8.00 |
n/a |
2.05 |
n/a |
n/a |
n/a |
n/a |
n/a |
Germany† |
66 |
8.10 |
n/a |
1.94 |
n/a |
n/a |
n/a |
n/a |
n/a |
Greece† |
50 |
6.90 |
Yes |
1.79 |
No |
n/a |
Yes |
Combined |
Very high |
Hungary† |
60 |
6.60 |
Yes |
2.03 |
No |
n/a |
No |
No |
Very high |
Iceland |
65 |
8.70 |
Yes |
n/a |
No |
National |
Yes |
No |
Very high |
Ireland |
57 |
7.70 |
Yes |
2.10 |
n/a |
n/a |
Yes |
No |
Low |
Israel |
50 |
7.30 |
Yes |
n/a |
n/a |
n/a |
No |
Yes |
Very high |
Italy† |
35 |
6.90 |
Yes |
2.19 |
No |
Local |
Yes |
Yes |
Very high |
Japan† |
n/a |
8.30 |
Yes |
n/a |
n/a |
National |
Yes |
No |
n/a |
Korea |
50 |
8.80 |
n/a |
n/a |
n/a |
n/a |
n/a |
n/a |
n/a |
Latvia |
48 |
6.90 |
Yes |
1.83 |
No |
National |
Yes |
Combined |
Low |
Lithuania† |
61 |
7.00 |
Yes |
1.65 |
Yes |
National |
Yes |
Yes |
High |
Luxembourg |
58 |
8.30 |
Yes |
1.78 |
No |
Local |
Yes |
Combined |
Very high |
Malta |
59 |
7.50 |
Yes |
n/a |
Yes |
n/a |
No |
No |
Very high |
Mexico† |
50 |
4.50 |
Yes |
n/a |
n/a |
n/a |
No |
No |
High |
Netherlands† |
74 |
8.40 |
Yes |
n/a |
Yes |
Local |
Yes |
No |
Very high |
New Zealand |
n/a |
8.10 |
Yes |
n/a |
n/a |
International, National, local |
Yes |
Combined |
Low |
Norway |
69 |
8.40 |
Yes |
n/a |
No |
n/a |
Yes |
Yes |
Very high |
Poland† |
47 |
6.60 |
Yes |
1.84 |
No |
Local |
Yes |
Combined |
Very high |
Portugal† |
49 |
6.60 |
Yes |
2.12 |
Yes |
Local |
No |
Yes |
High |
Romania |
33 |
5.90 |
Yes |
1.79 |
No |
n/a |
Yes |
n/a |
n/a |
Slovak Republic |
53 |
6.70 |
n/a |
1.76 |
n/a |
n/a |
n/a |
n/a |
|
Slovenia† |
48 |
7.10 |
Yes |
2.00 |
No |
n/a |
No |
No |
Very high |
Spain† |
60 |
7.50 |
Yes |
2.37 |
Yes |
Regional |
No |
No |
Very high |
Switzerland† |
67 |
8.50 |
Yes |
n/a |
No |
Local |
Yes |
No |
Low |
Türkiye† |
51 |
5.50 |
Yes |
n/a |
Yes |
National |
No |
Combined |
Low |
United Kingdom† |
67 |
8.50 |
Yes |
2.52 |
Yes |
Intermediate |
Yes |
Yes |
Very high |
United States |
38 |
8.10 |
Yes |
n/a |
n/a |
National |
Yes |
No |
n/a |
Note: n/a indicates data is missing. * Combined with eHealth policy or strategy. † indicates MASK currently operates in the country.
Source: See Table 15.2.
To help consolidate findings from the transferability assessment above, countries have been clustered into one of three groups, based on indicators reported in Table 15.2. Countries in clusters with more positive values have the greatest transfer potential. For further details on the methodological approach used, please refer to Annex A.
Key findings from each of the clusters are below with further details in Figure 15.3 and Table 15.4:
Several factors important for implementing and operating MASK are present in countries that fall under cluster one. For example, access to digital tools and digitally literate populations, as well as relatively high levels of funding for eHealth tools. Certain countries in this cluster could experience issues implementing MASK if the tool fails to align with high-level political objectives. Cluster one includes several countries that have previously transferred MASK including Australia, Austria, Canada, Denmark and Sweden.
Based on available data, countries in cluster two typically have strong digital health sectors that promote tools such as MASK (e.g. high levels of digital literacy among GPs and incentives to use mHealth apps). However, these countries may experience implementation barriers due to economic and political factors. It is important to note that certain countries in cluster two currently use MASK, indicating the factors used to assess transferability, although important, are not critical to the tool’s success.
Political objectives in countries that fall under cluster three tend to promote the use of mHealth apps such as MASK (e.g. A national eHealth and telehealth policy). Nevertheless, the readiness of the population and health sector utilise digital health tools may act as implementation barriers.
Figure 15.3. Transferability assessment using clustering – MASK
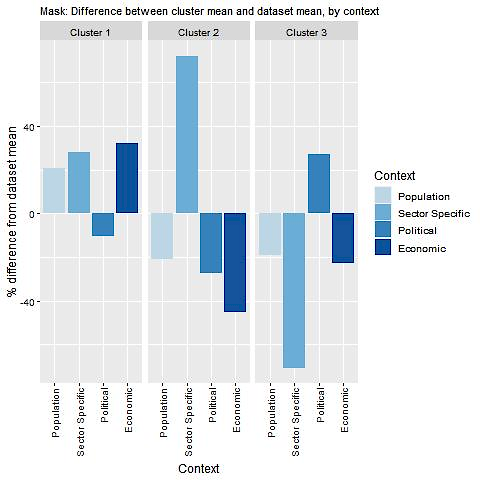
Note: Bar charts show percentage difference between cluster mean and dataset mean, for each indicator.
Source: See Table 15.2.
Table 15.4. Countries by cluster – MASK
Cluster 1 |
Cluster 2 |
Cluster 3 |
---|---|---|
Australia Austria Canada Croatia Denmark Estonia Finland Hungary Iceland Luxembourg Malta Netherlands Norway Slovenia Spain Sweden United Kingdom United States |
Czech Republic Israel Lithuania Mexico Portugal Türkiye |
Belgium Bulgaria Chile Costa Rica Cyprus Greece Ireland Italy Latvia New Zealand Poland Romania Switzerland |
Note: The following countries were omitted due to high levels of missing data: Colombia, France, Germany, Japan, Korea and the Slovak Republic.
New indicators to assess transferability
Data from publicly available datasets is not sufficient to assess the transferability of MASK. For example, there is no publicly available information the level of public acceptability of mHealth interventions. Therefore, Box 15.7 outlines several new indicators policy makers should consider before transferring MASK.
Box 15.7. New indicators, or factors, to consider when assessing transferability – MASK
In addition to the indicators within the transferability assessment, policy makers are encouraged to collect data for the following indicators:
Population context
How acceptable are mHealth interventions amongst the public?
Do patients have the skills to access healthcare online?
What proportion of the population has access to a smartphone and the internet?
Does the population trust their personal health information will be used, stored and managed appropriately?
Sector-specific context (digital health)
What, if any, compatible interventions exist?
What, if any, competing interventions exist? (e.g. other asthma/AR mHealth apps)
Are healthcare providers supportive of using digital products to treat patients with asthma/AR among the health profession?
Do regulations support integration of MASK into the healthcare guidelines? (relevant for countries who do not fall under GDPR rules (Genders Data Protection Regulation))
Political context
Has the intervention received political support from key decision-makers?
Has the intervention received commitment from key decision-makers?
Economic context
Are there additional cost of implemented the intervention in the target setting beyond those estimated by MASK administrators (see Box 15.3)?
Conclusion and next steps
MASK is a digital intervention designed to reduce the burden of AR and asthma. The MASK intervention is broken into two components – one for individuals and the other for health professionals. Individuals can download the MASK-air app, free‑of-charge, which includes a series of questions users fill out in regard to their daily symptoms and treatments. This information can be shared with health professionals who will have access to a compatible MASK-air Companion, which will act as an electronic decision support system once MASK is upgraded to a C2MD and a physician’s web-based questionnaire.
Data collected from the MASK-air app has been used to enhance the knowledge base related to AR and asthma. Key examples include the impact of AR/asthma symptoms on work productivity as well as a better understanding of how people adhere to medication. This information has been used to improve care guidelines to reflect real world experiences. In coming years, MASK administrators plan to undertake outcome evaluations which will examine the impact of MASK on symptoms, adherence, quality of life and work productivity.
MASK performs particularly well against the equity criterion. MASK reduces health inequalities by, first, offering people across the world access to the same quality of care for treating patients with AR and asthma. And, second, by accommodating the needs of priority populations in the design of the app, for example, by making it easy-to‑use and free of charge.
To enhance the performance of MASK several policy options are available. One key policy, which falls under the responsibility of high-level policy makers (e.g. at the national level), is to boost levels of digital HL amongst the public and health professionals. Policies available to MASK administrators include imputing features that allow data to be disaggregated by priority population groups, as well as using a multi-pronged, targeted approach to boost uptake.
MASK is a highly transferable digital health intervention. MASK was initially implemented in 18 countries and was subsequently transferred to a further 10, most of which are OECD and non-OECD EU countries. The highly transferable nature of MASK is accredited to its simple design and the network of AR and asthma experts who take responsibility for adapting the intervention to the local context.
MASK is an innovative digital health intervention with the potential to significantly improve outcomes (e.g. through change management) for those with AR and asthma while simultaneously reducing pressure on healthcare systems. Next steps for policy makers and funding agencies to promote MASK are outlined in Box 15.8.
Box 15.8. Next steps for policy makers and funding agencies – MASK
Next steps for policy makers and funding agencies to enhance MASK are listed below:
Support policy efforts to boost population health literacy and digital health literacy in order to increase uptake of mHealth apps such as MASK-air
Ensure health professionals receive ongoing education on digital health literacy, for example, by integrating digital health topics into training programs
Promote findings from the MASK case study to better understand what countries/regions are interested in transferring the intervention
Promote “lessons learnt” from countries that have transferred MASK to their local setting.
Annex 15.A. MACVIA algorithm for AR treatment
The figure below shows the step-up/step-down MACVIA algorithm to guide pharmacotherapy clinical decisions for patients with AR.
Annex Figure 15.A.1. MACVIA algorithm to guide pharmacotherapy for patients with AR
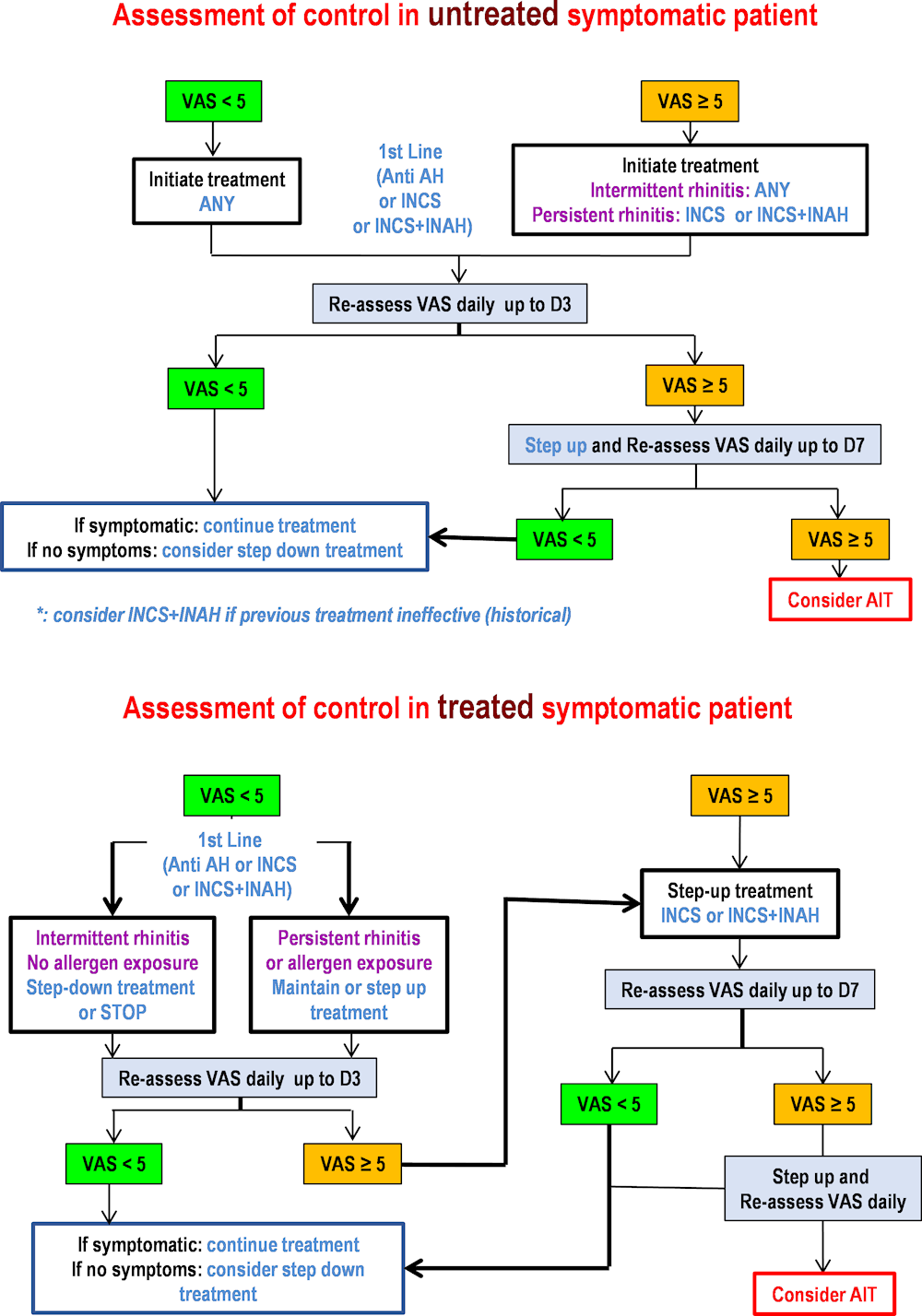
Note: AH = H1‑anti-histamine; INAH = Intra-nasal H1‑anti-histamine; INCS = Intra-nasal corticosteroid; AIT = Allergen immunotherapy; VAS = Visual analogue scale.
Source: Updated version of Bousquet et al. (2020[]), “Next-generation Allergic Rhinitis and Its Impact on Asthma (ARIA) guidelines for allergic rhinitis based on Grading of Recommendations Assessment, Development and Evaluation (GRADE) and real-world evidence”, https://www.doi.org/10.1016/j.jaci.2019.06.049.
Annex 15.B. MASK-air app price list
Annex Table 15.B.1. MASK-air price list (as of 2021)
Services |
Standard pack |
Advanced pack |
Options |
---|---|---|---|
Project supervision and follow up after launching |
✓ |
✓ |
|
Regulatory verification |
✓ |
✓ |
|
Terms of use translation |
Provided by your service |
✓ |
|
Application translation (interface‑daily follow-up profile) |
Provided by your service |
✓ |
|
Multi‑official languages (optional) app availability |
EUR 2 500 / languages |
EUR 2 500 / languages |
✓ |
Additional questionnaires (translation (EQ5D / WPAI: AS / EPWORTH / CARAT): Available in English/Optional translated in your language |
Provided by your service |
✓ |
✓ |
Medication list – appropriate in your country- providing |
Provided by your service |
✓ |
|
Graphic interface adaption |
✓ |
✓ |
EUR 2 500 for specific alphabet |
App store implementation and SO (IOS-Android) |
✓ |
✓ |
|
App store app description translation (IOS-Android) |
Provided by your service |
✓ |
|
Database adaptation |
✓ |
✓ |
|
Website translation and adaptation |
Provided by your service |
✓ |
✓ |
Technical Updates (IOS-Android) in the first year |
✓ |
✓ |
|
EUR 19 000 |
EUR 26 000 |
Source: Price list provided by MASK-air administrators.
References
[11] Amaral, R. et al. (2018), “Disentangling the heterogeneity of allergic respiratory diseases by latent class analysis reveals novel phenotypes”, Allergy, Vol. 74/4, pp. 698-708, https://doi.org/10.1111/all.13670.
[28] Azzopardi-Muscat, N. and K. Sørensen (2019), “Towards an equitable digital public health era: promoting equity through a health literacy perspective”, European journal of public health, Vol. 29/3, pp. 13-17, https://doi.org/10.1093/eurpub/ckz166.
[8] Bédard, A. et al. (2019), “Mobile technology offers novel insights into the control and treatment of allergic rhinitis: The MASK study”, Journal of Allergy and Clinical Immunology, Vol. 144/1, pp. 135-143.e6, https://doi.org/10.1016/j.jaci.2019.01.053.
[27] Bol, N., N. Helberger and J. Weert (2018), “Differences in mobile health app use: A source of new digital inequalities?”, The Information Society, Vol. 34/3, pp. 183-193, https://doi.org/10.1080/01972243.2018.1438550.
[4] Bousquet, J. et al. (2019), “Mobile Technology in Allergic Rhinitis: Evolution in Management or Revolution in Health and Care?”, The Journal of Allergy and Clinical Immunology: In Practice, Vol. 7/8, pp. 2511-2523, https://doi.org/10.1016/j.jaip.2019.07.044.
[3] Bousquet, J. et al. (2020), “ARIA digital anamorphosis: Digital transformation of health and care in airway diseases from research to practice”, Allergy, Vol. 76/1, pp. 168-190, https://doi.org/10.1111/all.14422.
[22] Bousquet, J. et al. (2018), MASK 2017: ARIA digitally-enabled, integrated, person-centred care for rhinitis and asthma multimorbidity using real-world-evidence, BioMed Central Ltd., https://doi.org/10.1186/s13601-018-0227-6.
[25] Bousquet, J. et al. (2017), “Work productivity in rhinitis using cell phones: The MASK pilot study”, Allergy, Vol. 72/10, pp. 1475-1484, https://doi.org/10.1111/all.13177.
[7] Bousquet, J. et al. (2018), “Treatment of allergic rhinitis using mobile technology with real-world data: The MASK observational pilot study”, Allergy, Vol. 73/9, pp. 1763-1774, https://doi.org/10.1111/all.13406.
[39] Bousquet, J. et al. (2019), “Allergic Rhinitis and its Impact on Asthma (ARIA) Phase 4 (2018): Change management in allergic rhinitis and asthma multimorbidity using mobile technology”, Journal of Allergy and Clinical Immunology, Vol. 143/3, pp. 864-879, https://doi.org/10.1016/j.jaci.2018.08.049.
[16] Bousquet, J. et al. (2020), “Next-generation Allergic Rhinitis and Its Impact on Asthma (ARIA) guidelines for allergic rhinitis based on Grading of Recommendations Assessment, Development and Evaluation (GRADE) and real-world evidence”, Journal of Allergy and Clinical Immunology, Vol. 145/1, pp. 70-80.e3, https://doi.org/10.1016/j.jaci.2019.06.049.
[19] Bousquet, J. et al. (2022), “Identification by cluster analysis of patients with asthma and nasal symptoms using the MASK-air® mHealth app”, Pulmonology, https://doi.org/10.1016/j.pulmoe.2022.10.005.
[21] Britto, M. et al. (2017), “A Randomized Trial of User-Controlled Text Messaging to Improve Asthma Outcomes: A Pilot Study”, Clinical Pediatrics, Vol. 56/14, pp. 1336-1344, https://doi.org/10.1177/0009922816684857.
[38] EU*US eHealth Work Project (2019), EU*US eHealth Work Project Resources, https://www.himss.org/professionaldevelopment/tiger-eu-us-project-resources.
[42] European Commission (2018), Benchmarking Deployment of eHealth among General Practitioners (2018), https://op.europa.eu/en/publication-detail/-/publication/d1286ce7-5c05-11e9-9c52-01aa75ed71a1.
[41] European Commission (2018), Benchmarking Deployment of eHealth among General Practitioners (2018), https://op.europa.eu/en/publication-detail/-/publication/d1286ce7-5c05-11e9-9c52-01aa75ed71a1.
[20] Farooqui, N. et al. (2015), “Acceptability of an interactive asthma management mobile health application for children and adolescents”, Annals of Allergy, Asthma & Immunology, Vol. 114/6, pp. 527-529, https://doi.org/10.1016/j.anai.2015.03.006.
[34] Goryakin, Y. et al. (2017), The Role of Communication in Public Health Policies - The case of obesity prevention in Italy, OECD, Paris, http://www.salute.gov.it/imgs/C_17_pubblicazioni_2647_allegato.pdf.
[33] Institute for Health Metrics and Evaluation (2019), GBD Results Tool | GHDx, http://ghdx.healthdata.org/gbd-results-tool (accessed on 19 September 2019).
[40] ITU (2020), The ICT Development Index (IDI): conceptual framework and methodology, https://www.itu.int/en/ITU-D/Statistics/Pages/publications/mis/methodology.aspx (accessed on 26 February 2021).
[9] Lemonnier, N. et al. (2020), “A novel whole blood gene expression signature for asthma, dermatitis, and rhinitis multimorbidity in children and adolescents”, Allergy, Vol. 75/12, pp. 3248-3260, https://doi.org/10.1111/all.14314.
[6] MACVIA working group (2018), “Daily allergic multimorbidity in rhinitis using mobile technology: A novel concept of the MASK study”, Allergy, Vol. 73/8, pp. 1622-1631, https://doi.org/10.1111/all.13448.
[29] OECD (2019), Health in the 21st Century: Putting Data to Work for Stronger Health Systems, OECD Health Policy Studies, OECD Publishing, Paris, https://doi.org/10.1787/e3b23f8e-en.
[43] OECD (2019), Individuals using the Internet for seeking health information - last 3 m (%) (all individuals aged 16-74), Dataset: ICT Access and Usage by Households and Individuals.
[35] OECD (2019), The Heavy Burden of Obesity: The Economics of Prevention, OECD Health Policy Studies, OECD Publishing, Paris, https://doi.org/10.1787/67450d67-en.
[36] OECD (2018), “Health literacy for people-centred care: Where do OECD countries stand?”, OECD Health Working Papers, No. 107, OECD Publishing, Paris, https://doi.org/10.1787/d8494d3a-en.
[31] OECD.Stat (2018), Population data - Total: all age groups.
[30] Oliveira Hashiguchi, T. (2020), “Bringing health care to the patient: An overview of the use of telemedicine in OECD countries”, OECD Health Working Papers, No. 116, OECD Publishing, Paris, https://doi.org/10.1787/8e56ede7-en.
[12] Raciborski, F. et al. (2019), “Dissociating polysensitization and multimorbidity in children and adults from a Polish general population cohort”, Clinical and Translational Allergy, Vol. 9/1, https://doi.org/10.1186/s13601-019-0246-y.
[10] Siroux, V. et al. (2019), “Association between asthma, rhinitis, and conjunctivitis multimorbidities with molecular IgE sensitization in adults”, Allergy, Vol. 74/4, pp. 824-827, https://doi.org/10.1111/all.13676.
[15] Sousa-Pinto et al. (forthcoming), Adherence to inhaled corticosteroids and long-acting β2-agonists in the MASK-air real-world data.
[17] Sousa‐Pinto, B. et al. (2022), “Development and validation of combined symptom‐medication scores for allergic rhinitis*”, Allergy, https://doi.org/10.1111/all.15199.
[18] Sousa-Pinto, B. et al. (2023), “Development and validation of an electronic daily control score for asthma (e-DASTHMA): a real-world direct patient data study”, The Lancet Digital Health, https://doi.org/10.1016/s2589-7500(23)00020-1.
[5] Sousa‐Pinto, B. et al. (2022), “Consistent trajectories of rhinitis control and treatment in 16,177 weeks: The <scp>MASK</scp> ‐air® longitudinal study”, Allergy, https://doi.org/10.1111/all.15574.
[14] The MASK Group (2019), “Adherence to treatment in allergic rhinitis using mobile technology”, Clinical & Experimental Allergy, Vol. 49/4, pp. 442-460, https://doi.org/10.1111/cea.13333.
[26] The MASK Study Group (2020), “Correlation between work impairment, scores of rhinitis severity and asthma using the MASK‐air”, Allergy, Vol. 75/7, pp. 1672-1688, https://doi.org/10.1111/all.14204.
[13] Toppila‐Salmi, S. et al. (2019), “Risk of adult‐onset asthma increases with the number of allergic multimorbidities and decreases with age”, Allergy, Vol. 74/12, pp. 2406-2416, https://doi.org/10.1111/all.13971.
[23] Vandenplas, O. et al. (2018), “Impact of Rhinitis on Work Productivity: A Systematic Review”, The Journal of Allergy and Clinical Immunology: In Practice, Vol. 6/4, pp. 1274-1286.e9, https://doi.org/10.1016/j.jaip.2017.09.002.
[1] WHO (2020), Asthma, https://www.who.int/news-room/fact-sheets/detail/asthma (accessed on 27 March 2021).
[37] WHO (2015), Atlas of eHealth country profiles: The use of eHealth in support of universal health coverage, Global Observatory for eHealth, https://www.afro.who.int/publications/atlas-ehealth-country-profiles-use-ehealth-support-universal-health-coverage.
[2] WHO and ITU (2017), Be He@lthy, Be Mobile: a handbook on how to implement mBreatheFreely, https://www.who.int/activities/Addressing-mobile-health.
[32] World Bank (2019), Population ages 0-14 (% of total population).
[44] World Bank (2017), GNI per capita, PPP (constant 2017 international $), https://data.worldbank.org/indicator/NY.GNP.PCAP.PP.KD.
[24] Zuberbier, T. et al. (2014), “Economic burden of inadequate management of allergic diseases in the European Union: a GA2LEN review”, Allergy, Vol. 69/10, pp. 1275-1279, https://doi.org/10.1111/all.12470.